Open Access
ARTICLE
Multi-Material Topology Optimization of 2D Structures Using Convolutional Neural Networks
1 College of Aerospace Science and Engineering, National University of Defense Technology, Changsha, 410073, China
2 Defense Innovation Institute, Chinese Academy of Military Science, Beijing, 100071, China
3 Intelligent Game and Decision Laboratory, Chinese Academy of Military Science, Beijing, 100071, China
* Corresponding Authors: Bingxiao Du. Email: ; Wen Yao. Email:
(This article belongs to the Special Issue: Structural Design and Optimization)
Computer Modeling in Engineering & Sciences 2024, 140(2), 1919-1947. https://doi.org/10.32604/cmes.2024.048118
Received 28 November 2023; Accepted 26 February 2024; Issue published 20 May 2024
Abstract
In recent years, there has been significant research on the application of deep learning (DL) in topology optimization (TO) to accelerate structural design. However, these methods have primarily focused on solving binary TO problems, and effective solutions for multi-material topology optimization (MMTO) which requires a lot of computing resources are still lacking. Therefore, this paper proposes the framework of multiphase topology optimization using deep learning to accelerate MMTO design. The framework employs convolutional neural network (CNN) to construct a surrogate model for solving MMTO, and the obtained surrogate model can rapidly generate multi-material structure topologies in negligible time without any iterations. The performance evaluation results show that the proposed method not only outputs multi-material topologies with clear material boundary but also reduces the calculation cost with high prediction accuracy. Additionally, in order to find a more reasonable modeling method for MMTO, this paper studies the characteristics of surrogate modeling as regression task and classification task. Through the training of 297 models, our findings show that the regression task yields slightly better results than the classification task in most cases. Furthermore, The results indicate that the prediction accuracy is primarily influenced by factors such as the TO problem, material category, and data scale. Conversely, factors such as the domain size and the material property have minimal impact on the accuracy.Keywords
Cite This Article
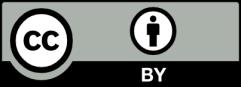
This work is licensed under a Creative Commons Attribution 4.0 International License , which permits unrestricted use, distribution, and reproduction in any medium, provided the original work is properly cited.