Open Access
ARTICLE
Improving Channel Estimation in a NOMA Modulation Environment Based on Ensemble Learning
1 Elearning Deanship and Distance Education, Umm Al-Qura University, P.O. Box 715, Mekkah, Saudi Arabia
2 Department of Information Systems, College of Computer and Information Sciences, Princess Nourah bint Abdulrahman University, P.O. Box 84428, Riyadh, 11671, Saudi Arabia
* Corresponding Author: Leila Jamel. Email:
Computer Modeling in Engineering & Sciences 2024, 140(2), 1315-1337. https://doi.org/10.32604/cmes.2024.047551
Received 09 November 2023; Accepted 06 March 2024; Issue published 20 May 2024
Abstract
This study presents a layered generalization ensemble model for next generation radio mobiles, focusing on supervised channel estimation approaches. Channel estimation typically involves the insertion of pilot symbols with a well-balanced rhythm and suitable layout. The model, called Stacked Generalization for Channel Estimation (SGCE), aims to enhance channel estimation performance by eliminating pilot insertion and improving throughput. The SGCE model incorporates six machine learning methods: random forest (RF), gradient boosting machine (GB), light gradient boosting machine (LGBM), support vector regression (SVR), extremely randomized tree (ERT), and extreme gradient boosting (XGB). By generating meta-data from five models (RF, GB, LGBM, SVR, and ERT), we ensure accurate channel coefficient predictions using the XGB model. To validate the modeling performance, we employ the leave-one-out cross-validation (LOOCV) approach, where each observation serves as the validation set while the remaining observations act as the training set. SGCE performances’ results demonstrate higher mean and median accuracy compared to the separated model. SGCE achieves an average accuracy of 98.4%, precision of 98.1%, and the highest F1-score of 98.5%, accurately predicting channel coefficients. Furthermore, our proposed method outperforms prior traditional and intelligent techniques in terms of throughput and bit error rate. SGCE’s superior performance highlights its efficacy in optimizing channel estimation. It can effectively predict channel coefficients and contribute to enhancing the overall efficiency of radio mobile systems. Through extensive experimentation and evaluation, we demonstrate that SGCE improved performance in channel estimation, surpassing previous techniques. Accordingly, SGCE’s capabilities have significant implications for optimizing channel estimation in modern communication systems.Keywords
Cite This Article
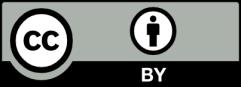
This work is licensed under a Creative Commons Attribution 4.0 International License , which permits unrestricted use, distribution, and reproduction in any medium, provided the original work is properly cited.