Open Access
ARTICLE
Suboptimal Feature Selection Techniques for Effective Malicious Traffic Detection on Lightweight Devices
1 Department of Future Convergence Technology Engineering, Sungshin Women’s University, Seoul, 02844, Korea
2 Department of Convergence Security Engineering, Sungshin Women’s University, Seoul, 02844, Korea
* Corresponding Author: Il-Gu Lee. Email:
(This article belongs to the Special Issue: Advanced Security for Future Mobile Internet: A Key Challenge for the Digital Transformation)
Computer Modeling in Engineering & Sciences 2024, 140(2), 1669-1687. https://doi.org/10.32604/cmes.2024.047239
Received 30 October 2023; Accepted 23 February 2024; Issue published 20 May 2024
Abstract
With the advancement of wireless network technology, vast amounts of traffic have been generated, and malicious traffic attacks that threaten the network environment are becoming increasingly sophisticated. While signature-based detection methods, static analysis, and dynamic analysis techniques have been previously explored for malicious traffic detection, they have limitations in identifying diversified malware traffic patterns. Recent research has been focused on the application of machine learning to detect these patterns. However, applying machine learning to lightweight devices like IoT devices is challenging because of the high computational demands and complexity involved in the learning process. In this study, we examined methods for effectively utilizing machine learning-based malicious traffic detection approaches for lightweight devices. We introduced the suboptimal feature selection model (SFSM), a feature selection technique designed to reduce complexity while maintaining the effectiveness of malicious traffic detection. Detection performance was evaluated on various malicious traffic, benign, exploits, and generic, using the UNSW-NB15 dataset and SFSM sub-optimized hyperparameters for feature selection and narrowed the search scope to encompass all features. SFSM improved learning performance while minimizing complexity by considering feature selection and exhaustive search as two steps, a problem not considered in conventional models. Our experimental results showed that the detection accuracy was improved by approximately 20% compared to the random model, and the reduction in accuracy compared to the greedy model, which performs an exhaustive search on all features, was kept within 6%. Additionally, latency and complexity were reduced by approximately 96% and 99.78%, respectively, compared to the greedy model. This study demonstrates that malicious traffic can be effectively detected even in lightweight device environments. SFSM verified the possibility of detecting various attack traffic on lightweight devices.Keywords
Cite This Article
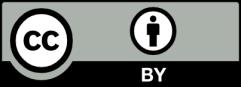
This work is licensed under a Creative Commons Attribution 4.0 International License , which permits unrestricted use, distribution, and reproduction in any medium, provided the original work is properly cited.