Open Access
ARTICLE
Enhancing Ulcerative Colitis Diagnosis: A Multi-Level Classification Approach with Deep Learning
Department of Information Systems, Faculty of Computing and Information Technology in Rabigh, King Abdulaziz University, Jeddah, 21589, Saudi Arabia
* Corresponding Author: Hasan J. Alyamani. Email:
(This article belongs to the Special Issue: Intelligent Medical Decision Support Systems: Methods and Applications)
Computer Modeling in Engineering & Sciences 2024, 140(1), 1129-1142. https://doi.org/10.32604/cmes.2024.047756
Received 16 November 2023; Accepted 04 February 2024; Issue published 16 April 2024
Abstract
The evaluation of disease severity through endoscopy is pivotal in managing patients with ulcerative colitis, a condition with significant clinical implications. However, endoscopic assessment is susceptible to inherent variations, both within and between observers, compromising the reliability of individual evaluations. This study addresses this challenge by harnessing deep learning to develop a robust model capable of discerning discrete levels of endoscopic disease severity. To initiate this endeavor, a multi-faceted approach is embarked upon. The dataset is meticulously preprocessed, enhancing the quality and discriminative features of the images through contrast limited adaptive histogram equalization (CLAHE). A diverse array of data augmentation techniques, encompassing various geometric transformations, is leveraged to fortify the dataset’s diversity and facilitate effective feature extraction. A fundamental aspect of the approach involves the strategic incorporation of transfer learning principles, harnessing a modified ResNet-50 architecture. This augmentation, informed by domain expertise, contributed significantly to enhancing the model’s classification performance. The outcome of this research endeavor yielded a highly promising model, demonstrating an accuracy rate of 86.85%, coupled with a recall rate of 82.11% and a precision rate of 89.23%.Graphic Abstract
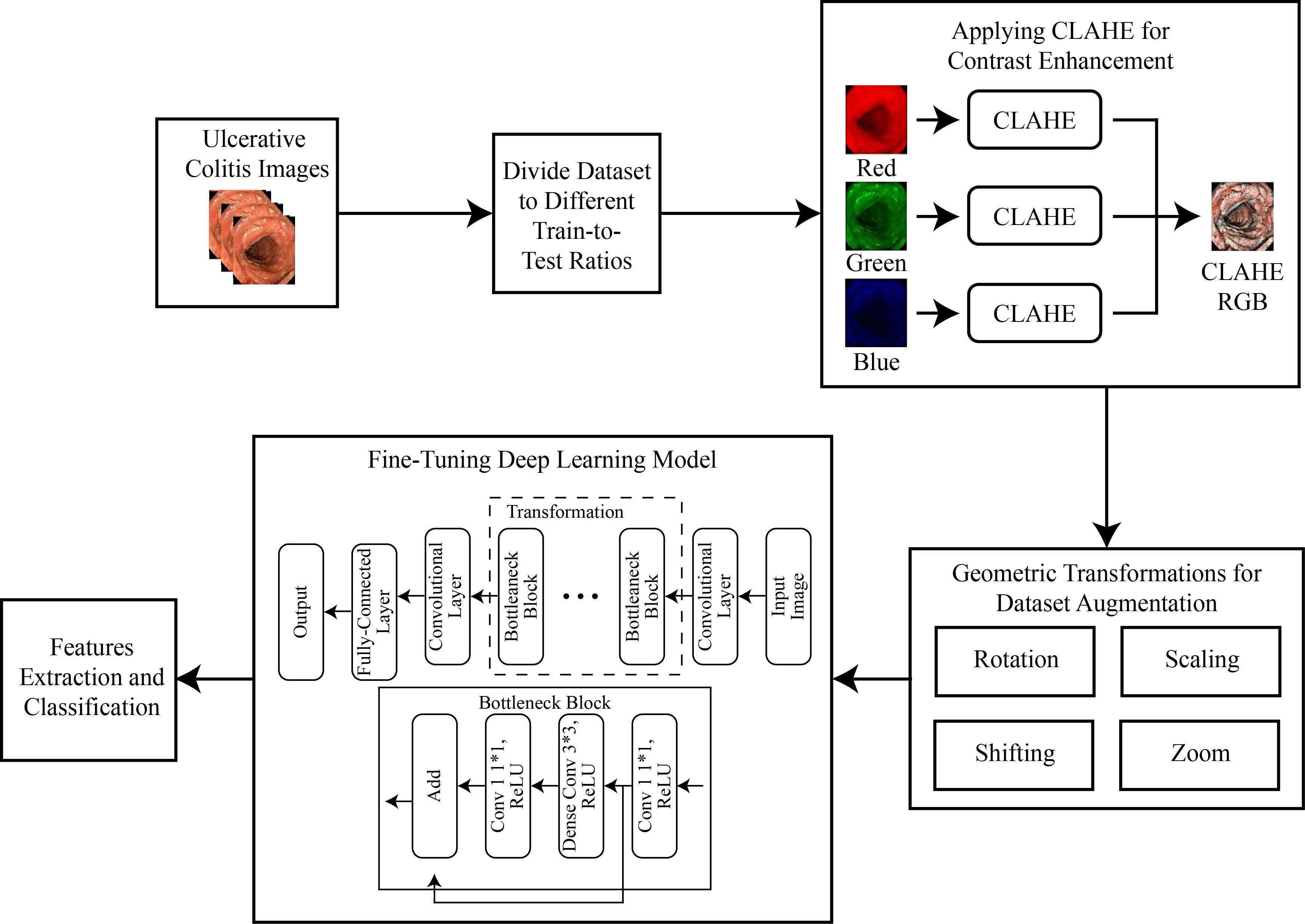
Keywords
Cite This Article
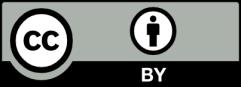
This work is licensed under a Creative Commons Attribution 4.0 International License , which permits unrestricted use, distribution, and reproduction in any medium, provided the original work is properly cited.