Open Access
ARTICLE
Deep Learning Social Network Access Control Model Based on User Preferences
1 School of Computer Science, Zhongyuan University of Technology, Zhengzhou, 450007, China
2 Henan Key Laboratory of Cyberspace Situation Awareness, Zhengzhou, 450001, China
* Corresponding Author: Fangfang Shan. Email:
(This article belongs to the Special Issue: Privacy-Preserving Technologies for Large-scale Artificial Intelligence)
Computer Modeling in Engineering & Sciences 2024, 140(1), 1029-1044. https://doi.org/10.32604/cmes.2024.047665
Received 13 November 2023; Accepted 25 January 2024; Issue published 16 April 2024
Abstract
A deep learning access control model based on user preferences is proposed to address the issue of personal privacy leakage in social networks. Firstly, social users and social data entities are extracted from the social network and used to construct homogeneous and heterogeneous graphs. Secondly, a graph neural network model is designed based on user daily social behavior and daily social data to simulate the dissemination and changes of user social preferences and user personal preferences in the social network. Then, high-order neighbor nodes, hidden neighbor nodes, displayed neighbor nodes, and social data nodes are used to update user nodes to expand the depth and breadth of user preferences. Finally, a multi-layer attention network is used to classify user nodes in the homogeneous graph into two classes: allow access and deny access. The fine-grained access control problem in social networks is transformed into a node classification problem in a graph neural network. The model is validated using a dataset and compared with other methods without losing generality. The model improved accuracy by 2.18 compared to the baseline method GraphSAGE, and improved F1 score by 1.45 compared to the baseline method, verifying the effectiveness of the model.Keywords
Cite This Article
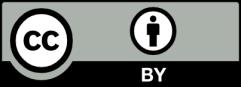
This work is licensed under a Creative Commons Attribution 4.0 International License , which permits unrestricted use, distribution, and reproduction in any medium, provided the original work is properly cited.