Open Access
ARTICLE
Predicting Rock Burst in Underground Engineering Leveraging a Novel Metaheuristic-Based LightGBM Model
1 No. Three Engineering Co., Ltd., CCCC First Highway Engineering Company, Beijing, 101102, China
2 Department of Civil Engineering, Faculty of Engineering, Universiti Malaya, Kuala Lumpur, 50603, Malaysia
3 Department of Civil Engineering, National Institute of Technology Patna, Bihar, 800005, India
4 School of Resources and Safety Engineering, Central South University, Changsha, 410083, China
* Corresponding Author: Biao He. Email:
Computer Modeling in Engineering & Sciences 2024, 140(1), 229-253. https://doi.org/10.32604/cmes.2024.047569
Received 09 November 2023; Accepted 23 January 2024; Issue published 16 April 2024
Abstract
Rock bursts represent a formidable challenge in underground engineering, posing substantial risks to both infrastructure and human safety. These sudden and violent failures of rock masses are characterized by the rapid release of accumulated stress within the rock, leading to severe seismic events and structural damage. Therefore, the development of reliable prediction models for rock bursts is paramount to mitigating these hazards. This study aims to propose a tree-based model—a Light Gradient Boosting Machine (LightGBM)—to predict the intensity of rock bursts in underground engineering. 322 actual rock burst cases are collected to constitute an exhaustive rock burst dataset, which serves to train the LightGBM model. Two population-based metaheuristic algorithms are used to optimize the hyperparameters of the LightGBM model. Finally, the sensitivity analysis is used to identify the predominant factors that may incur the occurrence of rock bursts. The results show that the population-based metaheuristic algorithms have a good ability to search out the optimal hyperparameters of the LightGBM model. The developed LightGBM model yields promising performance in predicting the intensity of rock bursts, with which accuracy on training and testing sets are 0.972 and 0.944, respectively. The sensitivity analysis discloses that the risk of occurring rock burst is significantly sensitive to three factors: uniaxial compressive strength (σc), stress concentration factor (SCF), and elastic strain energy index (Wet). Moreover, this study clarifies the particular impact of these three factors on the intensity of rock bursts through the partial dependence plot.Keywords
Cite This Article
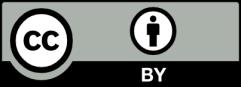