Open Access
ARTICLE
Perception Enhanced Deep Deterministic Policy Gradient for Autonomous Driving in Complex Scenarios
1 Fujian Provincial Key Laboratory of Automotive Electronics and Electric Drive, Fujian University of Technology, Fuzhou, 350118, China
2 Fujian Provincial Universities Engineering Research Center for Intelligent Driving Technology, Fuzhou, 350118, China
* Corresponding Author: Hankun Xiao. Email:
(This article belongs to the Special Issue: Intelligent Analysis of Imperfect Data in Complex Scenes: Modeling, Learning, and Optimization)
Computer Modeling in Engineering & Sciences 2024, 140(1), 557-576. https://doi.org/10.32604/cmes.2024.047452
Received 06 November 2023; Accepted 06 February 2024; Issue published 16 April 2024
Abstract
Autonomous driving has witnessed rapid advancement; however, ensuring safe and efficient driving in intricate scenarios remains a critical challenge. In particular, traffic roundabouts bring a set of challenges to autonomous driving due to the unpredictable entry and exit of vehicles, susceptibility to traffic flow bottlenecks, and imperfect data in perceiving environmental information, rendering them a vital issue in the practical application of autonomous driving. To address the traffic challenges, this work focused on complex roundabouts with multi-lane and proposed a Perception Enhanced Deep Deterministic Policy Gradient (PE-DDPG) for Autonomous Driving in the Roundabouts. Specifically, the model incorporates an enhanced variational autoencoder featuring an integrated spatial attention mechanism alongside the Deep Deterministic Policy Gradient framework, enhancing the vehicle’s capability to comprehend complex roundabout environments and make decisions. Furthermore, the PE-DDPG model combines a dynamic path optimization strategy for roundabout scenarios, effectively mitigating traffic bottlenecks and augmenting throughput efficiency. Extensive experiments were conducted with the collaborative simulation platform of CARLA and SUMO, and the experimental results show that the proposed PE-DDPG outperforms the baseline methods in terms of the convergence capacity of the training process, the smoothness of driving and the traffic efficiency with diverse traffic flow patterns and penetration rates of autonomous vehicles (AVs). Generally, the proposed PE-DDPG model could be employed for autonomous driving in complex scenarios with imperfect data.Keywords
Cite This Article
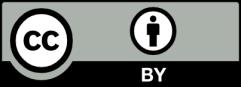