Open Access
ARTICLE
Conditional Generative Adversarial Network Enabled Localized Stress Recovery of Periodic Composites
1 Transportation and Municipal Engineering Institute, Powerchina Huadong Engineering Corporation, Hangzhou, 310014, China
2 College of Civil Engineering and Architecture, Zhejiang University, Hangzhou, 310058, China
3 Anhui Transport Consulting & Design Institute Co., Ltd., Hefei, 230088, China
4 Center for Balance Architecture, Zhejiang University, Hangzhou, 310058, China
* Corresponding Authors: Guannan Wang. Email: ; He Zhang. Email:
(This article belongs to the Special Issue: Machine Learning Based Computational Mechanics)
Computer Modeling in Engineering & Sciences 2024, 140(1), 957-974. https://doi.org/10.32604/cmes.2024.047327
Received 02 November 2023; Accepted 30 January 2024; Issue published 16 April 2024
Abstract
Structural damage in heterogeneous materials typically originates from microstructures where stress concentration occurs. Therefore, evaluating the magnitude and location of localized stress distributions within microstructures under external loading is crucial. Repeating unit cells (RUCs) are commonly used to represent microstructural details and homogenize the effective response of composites. This work develops a machine learning-based micromechanics tool to accurately predict the stress distributions of extracted RUCs. The locally exact homogenization theory efficiently generates the microstructural stresses of RUCs with a wide range of parameters, including volume fraction, fiber/matrix property ratio, fiber shapes, and loading direction. Subsequently, the conditional generative adversarial network (cGAN) is employed and constructed as a surrogate model to establish the statistical correlation between these parameters and the corresponding localized stresses. The stresses predicted by cGAN are validated against the remaining true data not used for training, showing good agreement. This work demonstrates that the cGAN-based micromechanics tool effectively captures the local responses of composite RUCs. It can be used for predicting potential crack initiations starting from microstructures and evaluating the effective behavior of periodic composites.Graphic Abstract
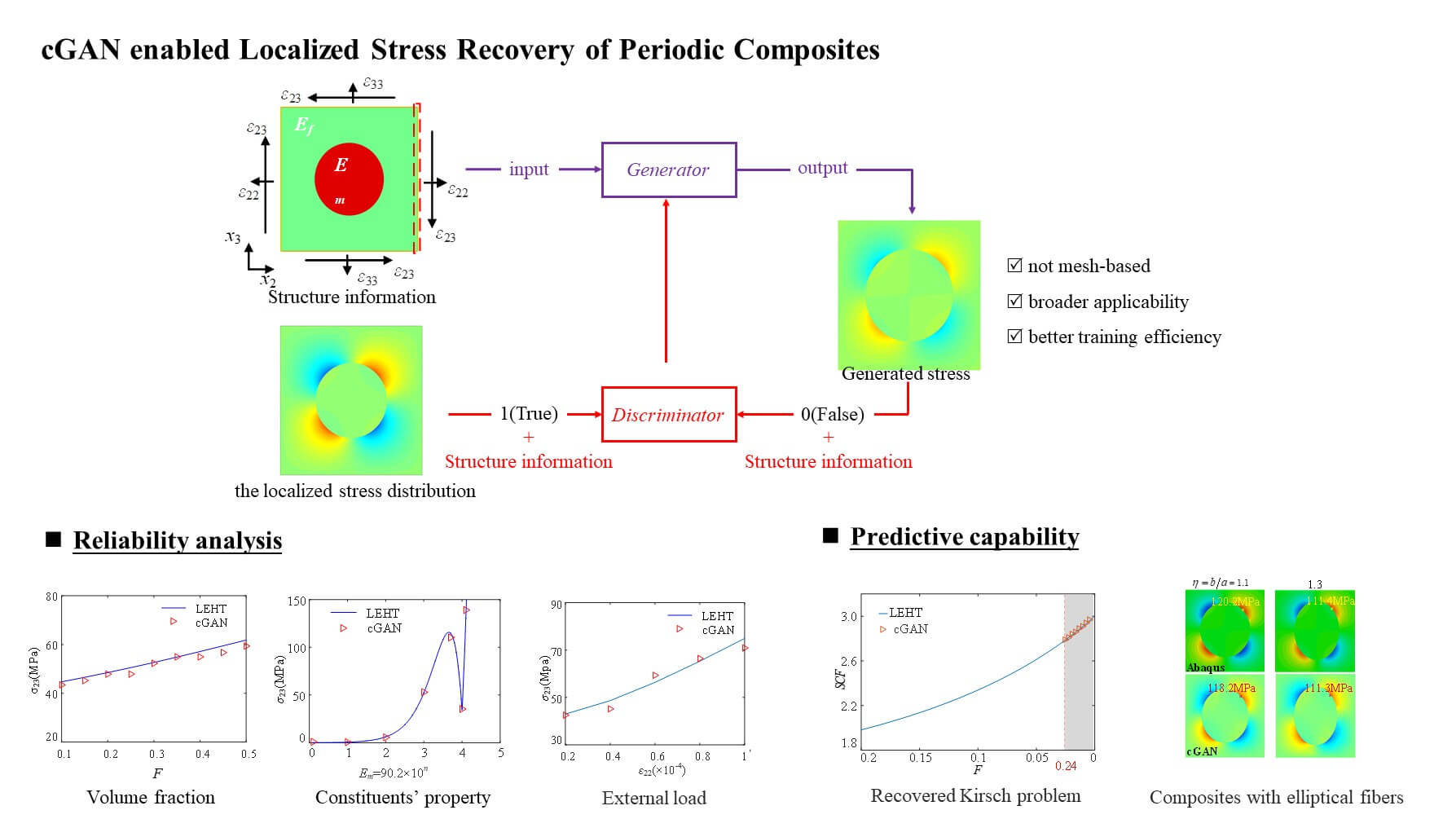
Keywords
Cite This Article
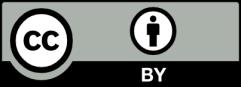
This work is licensed under a Creative Commons Attribution 4.0 International License , which permits unrestricted use, distribution, and reproduction in any medium, provided the original work is properly cited.