Open Access
ARTICLE
Uniaxial Compressive Strength Prediction for Rock Material in Deep Mine Using Boosting-Based Machine Learning Methods and Optimization Algorithms
School of Resources and Safety Engineering, Central South University, Changsha, 410083, China
* Corresponding Author: Diyuan Li. Email:
Computer Modeling in Engineering & Sciences 2024, 140(1), 275-304. https://doi.org/10.32604/cmes.2024.046960
Received 20 October 2023; Accepted 24 January 2024; Issue published 16 April 2024
Abstract
Traditional laboratory tests for measuring rock uniaxial compressive strength (UCS) are tedious and time-consuming. There is a pressing need for more effective methods to determine rock UCS, especially in deep mining environments under high in-situ stress. Thus, this study aims to develop an advanced model for predicting the UCS of rock material in deep mining environments by combining three boosting-based machine learning methods with four optimization algorithms. For this purpose, the Lead-Zinc mine in Southwest China is considered as the case study. Rock density, P-wave velocity, and point load strength index are used as input variables, and UCS is regarded as the output. Subsequently, twelve hybrid predictive models are obtained. Root mean square error (RMSE), mean absolute error (MAE), coefficient of determination (R2), and the proportion of the mean absolute percentage error less than 20% (A-20) are selected as the evaluation metrics. Experimental results showed that the hybrid model consisting of the extreme gradient boosting method and the artificial bee colony algorithm (XGBoost-ABC) achieved satisfactory results on the training dataset and exhibited the best generalization performance on the testing dataset. The values of R2, A-20, RMSE, and MAE on the training dataset are 0.98, 1.0, 3.11 MPa, and 2.23 MPa, respectively. The highest values of R2 and A-20 (0.93 and 0.96), and the smallest RMSE and MAE values of 4.78 MPa and 3.76 MPa, are observed on the testing dataset. The proposed hybrid model can be considered a reliable and effective method for predicting rock UCS in deep mines.Graphic Abstract
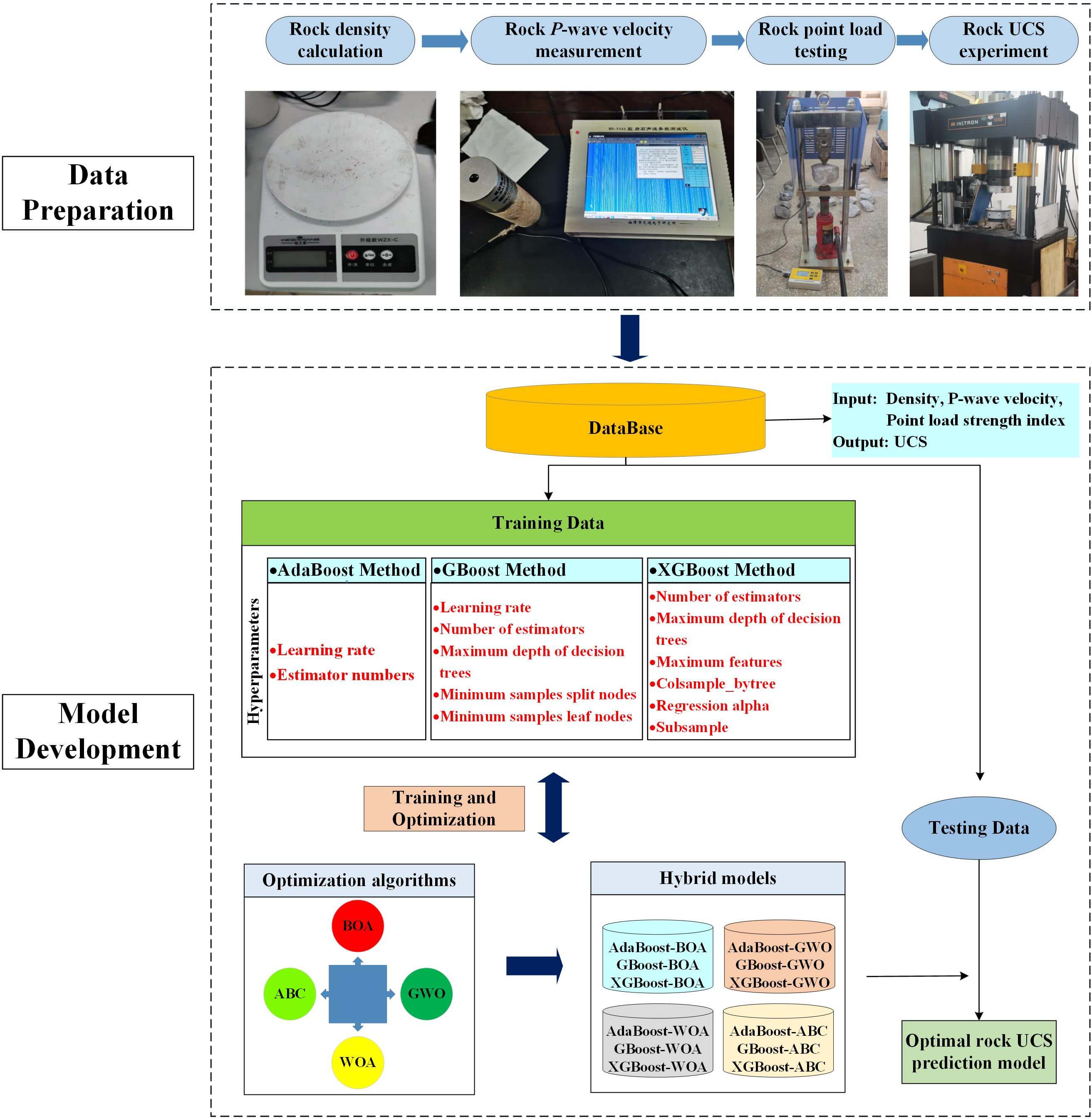
Keywords
Cite This Article
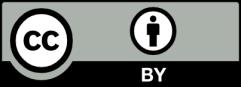
This work is licensed under a Creative Commons Attribution 4.0 International License , which permits unrestricted use, distribution, and reproduction in any medium, provided the original work is properly cited.