Open Access
REVIEW
A Review of Deep Learning-Based Vulnerability Detection Tools for Ethernet Smart Contracts
School of Computer Science and Technology, Zhengzhou University of Light Industry, Zhengzhou, 450001, China
* Corresponding Author: Yaqiong He. Email:
(This article belongs to the Special Issue: The Bottleneck of Blockchain Techniques: Scalability, Security and Privacy Protection)
Computer Modeling in Engineering & Sciences 2024, 140(1), 77-108. https://doi.org/10.32604/cmes.2024.046758
Received 13 October 2023; Accepted 08 January 2024; Issue published 16 April 2024
Abstract
In recent years, the number of smart contracts deployed on blockchain has exploded. However, the issue of vulnerability has caused incalculable losses. Due to the irreversible and immutability of smart contracts, vulnerability detection has become particularly important. With the popular use of neural network model, there has been a growing utilization of deep learning-based methods and tools for the identification of vulnerabilities within smart contracts. This paper commences by providing a succinct overview of prevalent categories of vulnerabilities found in smart contracts. Subsequently, it categorizes and presents an overview of contemporary deep learning-based tools developed for smart contract detection. These tools are categorized based on their open-source status, the data format and the type of feature extraction they employ. Then we conduct a comprehensive comparative analysis of these tools, selecting representative tools for experimental validation and comparing them with traditional tools in terms of detection coverage and accuracy. Finally, Based on the insights gained from the experimental results and the current state of research in the field of smart contract vulnerability detection tools, we suppose to provide a reference standard for developers of contract vulnerability detection tools. Meanwhile, forward-looking research directions are also proposed for deep learning-based smart contract vulnerability detection.Graphical Abstract
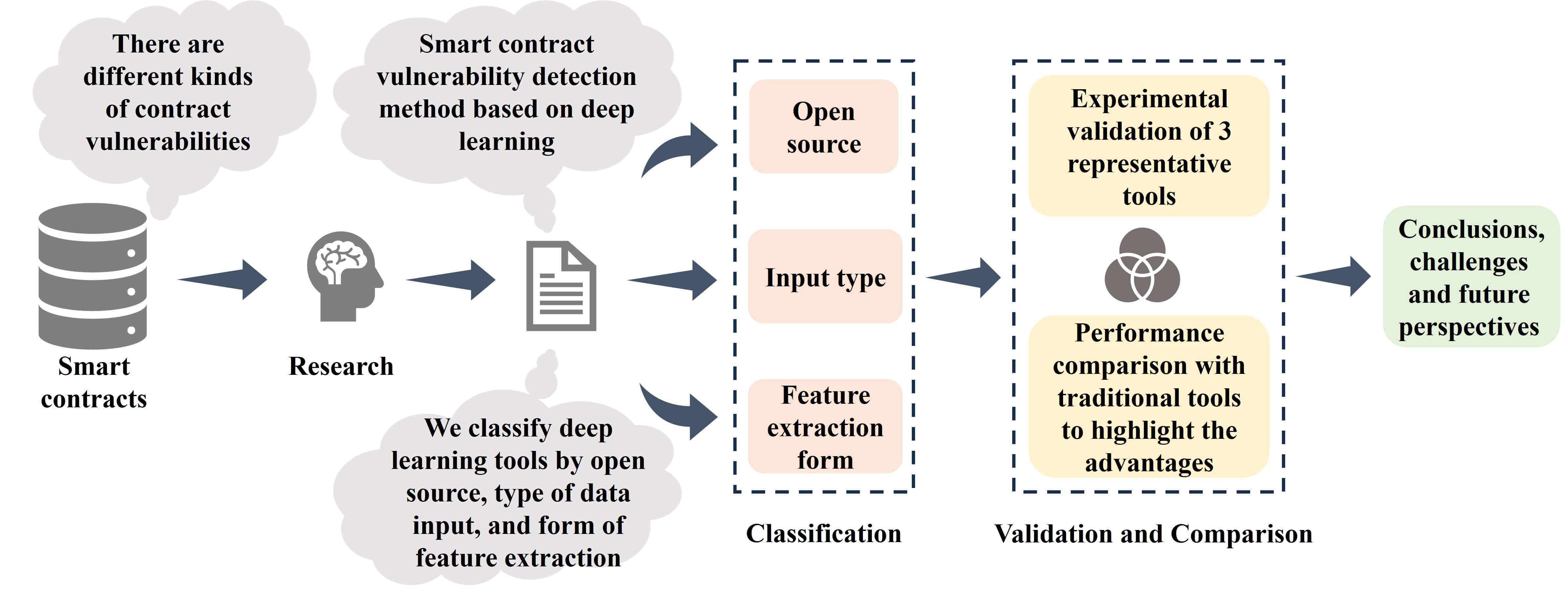
Keywords
Cite This Article
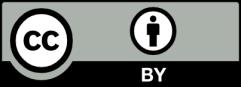