Open Access
ARTICLE
An Approach for Human Posture Recognition Based on the Fusion PSE-CNN-BiGRU Model
School of Building and Environment Engineering, Zhengzhou University of Light Industry, Zhengzhou, 450000, China
* Corresponding Author: Xin Geng. Email:
(This article belongs to the Special Issue: AI-Driven Engineering Applications)
Computer Modeling in Engineering & Sciences 2024, 140(1), 385-408. https://doi.org/10.32604/cmes.2024.046752
Received 13 October 2023; Accepted 04 January 2024; Issue published 16 April 2024
Abstract
This study proposes a pose estimation-convolutional neural network-bidirectional gated recurrent unit (PSE-CNN-BiGRU) fusion model for human posture recognition to address low accuracy issues in abnormal posture recognition due to the loss of some feature information and the deterioration of comprehensive performance in model detection in complex home environments. Firstly, the deep convolutional network is integrated with the Mediapipe framework to extract high-precision, multi-dimensional information from the key points of the human skeleton, thereby obtaining a human posture feature set. Thereafter, a double-layer BiGRU algorithm is utilized to extract multi-layer, bidirectional temporal features from the human posture feature set, and a CNN network with an exponential linear unit (ELU) activation function is adopted to perform deep convolution of the feature map to extract the spatial feature of the human posture. Furthermore, a squeeze and excitation networks (SENet) module is introduced to adaptively learn the importance weights of each channel, enhancing the network’s focus on important features. Finally, comparative experiments are performed on available datasets, including the public human activity recognition using smartphone dataset (UCIHAR), the public human activity recognition 70 plus dataset (HAR70PLUS), and the independently developed home abnormal behavior recognition dataset (HABRD) created by the authors’ team. The results show that the average accuracy of the proposed PSE-CNN-BiGRU fusion model for human posture recognition is 99.56%, 89.42%, and 98.90%, respectively, which are 5.24%, 5.83%, and 3.19% higher than the average accuracy of the five models proposed in the comparative literature, including CNN, GRU, and others. The F1-score for abnormal posture recognition reaches 98.84% (heartache), 97.18% (fall), 99.6% (bellyache), and 98.27% (climbing) on the self-built HABRD dataset, thus verifying the effectiveness, generalization, and robustness of the proposed model in enhancing human posture recognition.Keywords
Cite This Article
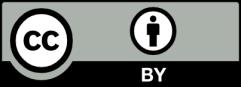
This work is licensed under a Creative Commons Attribution 4.0 International License , which permits unrestricted use, distribution, and reproduction in any medium, provided the original work is properly cited.