Open Access
ARTICLE
Transparent and Accurate COVID-19 Diagnosis: Integrating Explainable AI with Advanced Deep Learning in CT Imaging
1 Department of Information Systems, College of Computer and Information Sciences, King Saud University, Riyadh, 11543, Saudi Arabia
2 Department of Computer Engineering, College of Computer and Information Sciences, King Saud University, Riyadh, 11543, Saudi Arabia
3 Science Technology and Innovation Department, King Saud University, Riyadh, 11543, Saudi Arabia
* Corresponding Author: Mohammad Mehedi Hassan. Email:
(This article belongs to the Special Issue: Intelligent Medical Decision Support Systems: Methods and Applications)
Computer Modeling in Engineering & Sciences 2024, 139(3), 3101-3123. https://doi.org/10.32604/cmes.2024.047940
Received 23 November 2023; Accepted 17 January 2024; Issue published 11 March 2024
Abstract
In the current landscape of the COVID-19 pandemic, the utilization of deep learning in medical imaging, especially in chest computed tomography (CT) scan analysis for virus detection, has become increasingly significant. Despite its potential, deep learning’s “black box” nature has been a major impediment to its broader acceptance in clinical environments, where transparency in decision-making is imperative. To bridge this gap, our research integrates Explainable AI (XAI) techniques, specifically the Local Interpretable Model-Agnostic Explanations (LIME) method, with advanced deep learning models. This integration forms a sophisticated and transparent framework for COVID-19 identification, enhancing the capability of standard Convolutional Neural Network (CNN) models through transfer learning and data augmentation. Our approach leverages the refined DenseNet201 architecture for superior feature extraction and employs data augmentation strategies to foster robust model generalization. The pivotal element of our methodology is the use of LIME, which demystifies the AI decision-making process, providing clinicians with clear, interpretable insights into the AI’s reasoning. This unique combination of an optimized Deep Neural Network (DNN) with LIME not only elevates the precision in detecting COVID-19 cases but also equips healthcare professionals with a deeper understanding of the diagnostic process. Our method, validated on the SARS-COV-2 CT-Scan dataset, demonstrates exceptional diagnostic accuracy, with performance metrics that reinforce its potential for seamless integration into modern healthcare systems. This innovative approach marks a significant advancement in creating explainable and trustworthy AI tools for medical decision-making in the ongoing battle against COVID-19.Keywords
Cite This Article
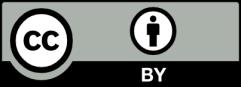