Open Access
REVIEW
Saddlepoint Approximation Method in Reliability Analysis: A Review
1 School of Mechanical and Electrical Engineering, University of Electronic Science and Technology of China, Chengdu, 611731, China
2 Institute of Electronic and Information Engineering of UESTC in Guangdong, Dongguan, 523808, China
3 Glasgow College, University of Electronic Science and Technology of China, Chengdu, 611731, China
4 Beijing Research Institute of Mechanical & Electrical Technology, Ltd., Beijing, 100083, China
* Corresponding Authors: Debiao Meng. Email: ; Shiyuan Yang. Email:
(This article belongs to the Special Issue: Computer-Aided Uncertainty Modeling and Reliability Evaluation for Complex Engineering Structures)
Computer Modeling in Engineering & Sciences 2024, 139(3), 2329-2359. https://doi.org/10.32604/cmes.2024.047507
Received 07 November 2023; Accepted 21 December 2023; Issue published 11 March 2024
Abstract
The escalating need for reliability analysis (RA) and reliability-based design optimization (RBDO) within engineering challenges has prompted the advancement of saddlepoint approximation methods (SAM) tailored for such problems. This article offers a detailed overview of the general SAM and summarizes the method characteristics first. Subsequently, recent enhancements in the SAM theoretical framework are assessed. Notably, the mean value first-order saddlepoint approximation (MVFOSA) bears resemblance to the conceptual framework of the mean value second-order saddlepoint approximation (MVSOSA); the latter serves as an auxiliary approach to the former. Their distinction is rooted in the varying expansion orders of the performance function as implemented through the Taylor method. Both the saddlepoint approximation and third-moment (SATM) and saddlepoint approximation and fourth-moment (SAFM) strategies model the cumulant generating function (CGF) by leveraging the initial random moments of the function. Although their optimal application domains diverge, each method consistently ensures superior relative precision, enhanced efficiency, and sustained stability. Every method elucidated is exemplified through pertinent RA or RBDO scenarios. By juxtaposing them against alternative strategies, the efficacy of these methods becomes evident. The outcomes proffered are subsequently employed as a foundation for contemplating prospective theoretical and practical research endeavors concerning SAMs. The main purpose and value of this article is to review the SAM and reliability-related issues, which can provide some reference and inspiration for future research scholars in this field.Keywords
A saddlepoint refers to a stationary point of a non-local extreme point, which is a singular point that is stable in one direction and unstable in the other direction [1]. The curves, surfaces, or hypersurfaces of the saddlepoint neighborhood of a smooth function are all located on different sides of the tangent to the saddlepoint. Fig. 1 illustrates a typical example of a saddlepoint.
Figure 1: Example diagram of saddlepoint
Saddlepoint approximation arises from the approximate statistics of the probability density function (PDF) and cumulative distribution function (CDF), particularly the latter [2,3]. It stands as a potent method among various integral approximation techniques, offering distinct advantages of a straightforward formula, swift computation, and effective approximation. Even in the presence of a limited sample size, the saddlepoint approximation method (SAM) largely meets application demands [4–8]. Throughout history, numerous scholars have explored and propelled the advancement of SAM [9–13]. Hu et al. [14] introduced a saddlepoint approximation technique for reliability assessment, eliminating the need for additional transformations and approximations to the quadratic function. As saddlepoint approximation continues to evolve, it finds extensive utility in both physics and practical engineering fields [15–19]. SAM has been instrumental in developing approximate solutions for robust convex optimization, leading to more resilient and efficient design strategies in engineering [20]. Its applications extend to queueing theory, insurance mathematics and statistics [21–23]. In the area of computational mechanics, the stabilized extended finite elements method for saddlepoint problems has proven effective for handling unfitted interfaces, enhancing the computational simulation of physical phenomena [24].
Particularly in reliability analysis (RA) and reliability-based design optimization (RBDO) domains, SAM can provide an important support. Yuan et al. [25] introduced a reliability-based multidisciplinary design optimization that combines saddlepoint approximation and third-moment techniques. Fig. 2 depicts the basic flow chart of RA using SAM in engineering.
Figure 2: RA based on SAM in engineering
The concept of reliability emerged in the 19th century, aligning with the advancements of the Industrial Revolution. The growing momentum of this era demanded a quantitative approach to reliability, specifically in factory production and the execution of engineering projects [26,27]. Consequently, reliability became an established integral discipline in engineering.
Let f(x)=0f(x)=0 be the true limit-state equation, with β representing the reliability, indicating the distance from the origin to the hyperplane in the standard normal space; then, the geometric representation of reliability and β can be expressed as in Fig. 3.
Figure 3: Geometric representation of reliability and β
Numerous researchers have extensively investigated and formulated various reliability methods tailored for practical engineering applications [28–32]. Relevant progress has been made in optimization and estimation strategies for system reliability assignment problems, demonstrating the importance of optimization methods in reliability engineering [33–36]. Machine learning-based methods are becoming increasingly influential in structural reliability analysis, offering new tools and perspectives for tackling reliability problems [37–40]. RA has been further developed in the following specific branches, including foundational and advanced techniques in moment methods for structural reliability [41–45], computational techniques and machine learning in structural reliability [46–50], time-dependent aspects and non-probabilistic approaches in RA [51–55] and human factors and adaptive techniques in RA [56–60].
RA also holds significant importance in ensuring the smooth operation of entire projects across diverse engineering fields [61–65]. It plays a cornerstone role in the fields [66–70]. Scholars have developed related techniques for fault diagnosis [71] and studied that dynamic and time-varying RA can predict system lifetime under fluctuating conditions [72–76]. This is critical for uninterrupted infrastructure functionality [77–81]. RA also has important analysis and reference significance for the sustained performance of power systems and modern technologies [82–85]. Moreover, the integration of machine learning stands out as one of the primary developmental directions in RA [86–90]. Fig. 4 illustrates the main categories within RA methods, and Fig. 5 depicts the primary categories in approximation methods.
Figure 4: Categories of RA
Figure 5: Categories of approximation in RA
The first-order reliability method (FORM) is one of the crucial and commonly utilized approaches within most probable point (MPP)-based methods [15]. Its fundamental logic involves approximating the actual limit-state function with a plane at the MPP, which occurs on the limit-state surface closest to the origin in the standard normal space [91,92]. FORM offers the advantage of effectively balancing efficiency and accuracy [93–95]. Shin and Lee used FORM to evaluate the accident probability and conducted numerical studies using a single truck model, and obtained results that met the given goals [96]. According to the characteristics of FORM, its fusion with uncertainty analysis yields significant benefits, leading to the development of related methods [97–100]. Additionally, FORM is also widely used in RA across various fields [101–105]. Many researchers focused on enhancing the stability and accuracy of FORM in RA, including innovative methods to control numerical instabilities and update failure points [106–110]. They also provided comparative analyses, theoretical insights, and reviews of FORM within the broader context of reliability methods [111–114]. Advanced integration and optimization of FORM are important future development directions for this method [115–118].
However, FORM overlooks the nonlinearity of the original limit-state function, often resulting in substantial errors in many cases. To address this, the second-order reliability method (SORM) was introduced. SORM involves a quadratic expansion of the limit-state function at the point nearest to the origin in the standard normal coordinate system. By considering the nonlinearity, SORM approximates the limit-state function using a parabolic surface, providing more accurate reliability calculations than FORM [119]. Consequently, SORM commonly utilizes a parabolic approximation of the fitted quadratic polynomial surface and its related theories have been well developed [120–123]. Many related efficient and improved algorithms have been proposed [124–127]. Some novel approximation and extension methods are also used to enhance SORM [128–132]. As an advanced iteration of FORM, SORM is also widely used in engineering. The main areas included are geotechnical engineering and underground structures [133–136], advanced SORM techniques and sensitivity analysis [137–140], design optimization of structures [141–144], RA in mechanical and fatigue analysis [145–147]. Scholars have proven its effectiveness by comparing FORM/SORM with other methods [148,149]. Fig. 6 illustrates the basic principles and distinctions between FORM and SORM.
Figure 6: Basic principles of FORM and SORM
In the context of RA, there are many analysis methods that are closely related to saddlepoint approximation [150–152]. Although FORM is commonly applied in RA, its limitations in handling inaccurate situations for nonlinear problems have prompted the introduction of the SAM, which offers improved solutions [153]. The utilization of second-order saddlepoint approximation (SOSA) in component RA has demonstrated enhanced accuracy compared with the traditional SORM [154].
Reliability metrics have led to advancements in RBDO methods [155–157]. Approximate model technology has important applications in RBDO [158,159]. Many scholars have developed RBDO by integrating novel algorithms [160–163]. Liu et al. developed a Multi-objective RBDO based on probability and interval hybrid model [164]. Meng et al. proposed a general fidelity transformation framework for RBDO with arbitrary precision [165]. Product reliability design optimization aims to achieve optimal design probabilistically, utilizing product reliability as either a constraint or an objective and employing appropriate optimization techniques. The effectiveness of RBDO serves as a critical metric to assess its real-world applicability [166]. However, a prevalent challenge across various RBDO methodologies is their substantial computational demand [167–169]. In this regard, relevant scholars have also proposed some Efficient and Robust RBDO Methods [170–172]. Integrating SAM with RBDO analysis allows for mitigating this issue. Consequently, the fusion of SAM and RBDO has garnered significant attention in academic investigations [173,174]. Fig. 7 illustrates the relationship between reliability analysis and optimization. Moreover, other theoretical methods apply to RA [175–177].
Figure 7: Relationship between reliability analysis and optimization
This article offers an overview of the utilization and progression of SAMs in RA and RBDO. It can serve as a valuable reference and guide for subsequent research in this domain. The article is structured into five sections. The second section presents the fundamental algorithmic logic of the general SAM. The third section presents the core algorithmic logic and distinct features of recently enhanced SAMs. The fourth section discusses the application and performance of various SAMs in RA and RBDO engineering examples, summarizing the merits of each approach. Finally, the last section presents analytical conclusions and outlines potential directions for future studies.
SAM is an effective approximation tool, particularly suited for tackling high-dimensional integration challenges. This section introduces the general process of SAM. Its fundamental logic lies in obtaining the cumulant generating function (CGF) of a random variable, which enables approximations of the associated CDF and PDF. Calculating CDFs and PDFs is a crucial aspect of engineering analysis. Therefore, the primary objective of the general SAM is to facilitate more convenient computations of CDFs and PDFs.
Table 1 presents some common distributions of CGFs [178].
Let f(xR)f(xR) be the PDF of the random variable XRXR; then, the moment-generating function (MGF) of XRXR is denoted as:
MXR(t)=∫+∞−∞etxRf(xR)dxRMXR(t)=∫+∞−∞etxRf(xR)dxR(1)
The CGF of XRXR is expressed as:
KXR(t)=ln[MXR(t)]=∫+∞−∞etxRf(xR)dxRKXR(t)=ln[MXR(t)]=∫+∞−∞etxRf(xR)dxR(2)
For XRXR, the approximation of the PDF of XRXR can be expressed using saddlepoint approximation:
fXR(xR)=[12πK″XR(˜t)]12e[KXR(˜t)−˜txR](3)
where ˜t is the solution of equation K′XR(t)=xR.
Let φ(⋅) and Φ(⋅) be the standard normal distributions of the PDF and CDF, respectively. Generally, the failure probability FXR(xR) can be denoted as:
FXR(xR)=Pr[XR≤xR]=Φ(w)+φ(w)(1w−1v)(4)
or
FXR(xR)=Pr[XR≤xR]=Φ[w+1wlogvw](5)
where w is the solution of Eq. (6):
w=sign(˜t){2[˜txR−KXR(˜t)]}12(6)
and v is the solution of Eq. (7):
v=˜t[K″XR(˜t)]12(7)
sign(˜t) is the sign function:
sign(˜t)={1,˜t>00,˜t=0−1,˜t<0(8)
Under normal circumstances, we choose the maximum-likelihood point X∗R as the analysis point, X∗R=(x∗R1,x∗R2,x∗R3,…,x∗Rm). The first-order expansion of G=G(XR,j) at X∗R can be obtained as Eq. (9) using Taylor’s formula:
G≈ˆG=G(X∗R)+m∑j=1∂G∂XRj|X∗Rj(XRj−x∗Rj)(9)
According to the linear relationship above, the CGFs of KXRj can be expressed in terms of G:
KG(t)≈[G(X∗R)−m∑j=1∂G∂XRj|x∗Rjx∗Rj]t+m∑j=1KXRj[∂G∂XRj|X∗Rjt](10)
The approximation of the CDF of G can be solved using Eqs. (4)–(8). ˜t can be calculated using Eq. (11):
K′G(t)≈(G(X∗R)−m∑j=1∂G∂XRj|x∗Rjx∗Rj)+m∑j=1∂G∂XRj|x∗RjK′XRj[∂G∂XRj|x∗Rjt]=g(11)
This section offers a comprehensive review of the conventional SAM. The resultant equations are highly nonlinear, and the CGFs of XRj are essential to approximate the CDF of G. These limitations may confine the application of SAMs within engineering contexts. Therefore, there exists a pressing need to enhance this method to increase its practical value.
The general SAM features limitations in its application. Utilizing SAM necessitates acquiring the saddlepoint equation, which relies on tractable variables having existing CGFs. In cases in which CGFs are nonexistent, the general SAM cannot be applied. Additionally, for most probability distribution types, the corresponding CGFs tend to be complex, leading to potentially highly nonlinear saddlepoint equations [174]. These factors restrict SAM utilization. To enhance the efficiency and accuracy of SAM and address the shortcomings of the traditional approach, several scholars have introduced improvements and demonstrated the effectiveness of these methods in practical engineering applications. Fig. 8 depicts numerous reliability analysis methods based on saddlepoint approximation. This article introduces four of these methods in detail. They are mean value first-order saddlepoint approximation (MVFOSA), mean value second-order saddlepoint approximation (MVSOSA), saddlepoint approximation and third-moment (SATM) and saddlepoint approximation and fourth-moment (SAFM).
Figure 8: Improved SAMs
MVFOSA demonstrates efficiency and robustness comparable to those of the general SAM but offers superior accuracy. MVFOSA utilizes the first-order Taylor method to linearly expand the performance function G within the original random space [178].
In MVFOSA, d∗i and μXRi are selected as the expansion point. These two variables represent deterministic variables and mean values of random variables, respectively. The first-order approximation function is as follows:
G≈ˆG=ˆg(d,XR)=g(d∗,μXR)+n∑i=1∂G∂di|di(di−d∗i)+n∑i=1∂G∂XRi|μXRi(XRi−μXRi)(12)
The CGF of XR is denoted as KXR(t), and it has two useful properties:
Property 1. When XR=(XR1,XR2,…,XRn) are independent random variables and the CGFs of the variables are KXRi(t)(i=1,2,…,n), the CGF of Y=n∑i=1XRi can be calculated as KY(t)=n∑i=1KRi(t).
Property 2. Let XR be a random variable, its CGF is KXR(t). If Y=cXR+d, where c and d are constants, the CGF of Y can be calculated as KY(t)=KXR(ct)+dt.
For example, if XR follows χ2 distribution with CGF KXR(t)=−110nln(3−4t), then the CGF of Y is KY(t)=−110nln(3−4ct)+dt.
According to the abovementioned two properties, the CGF of G can be calculated using Eq. (13):
KˆG(t)=(g(d∗,µXR)+n∑i=1∂Ω∂di|di(di−d∗i)−n∑i=1∂G∂XRi|μXRiμXRi)t+n∑i=1KXRi(∂G∂XRi|μXRit)(13)
K′(t) is the first-order derivative of CGF; then, ts is the solution of Eq. (14):
K′ˆG(t)=(g(d∗,µXR)+n∑i=1∂Ω∂di|di(di−d∗i)−n∑i=1∂G∂XRi|μXRiμXRi)+∂G∂XRi|μXRin∑i=1K′XRi(∂G∂XRi|μXRit)=0(14)
When both the CGF of G and the saddlepoint ts are obtained, the CDF and PDF can be estimated via MVFOSA.
As previously highlighted, MVFOSA utilizes comprehensive distribution information, necessitating only a saddlepoint identification process. Owing to these characteristics, MVFOSA achieves enhanced accuracy.
MVSOSA represents an alternative method to MVFOSA [179]. The primary distinction lies in MVSOSA’s utilization of the mean values of random information as the point for the second-order Taylor expansion G(XR). It can be denoted as Eq. (15):
G(XR)=G(μ)+n∑i=1∂G∂Xi|μ(Xi−μi)+12n∑i=1n∑j=1∂2G∂Xi∂Xj(Xi−μi)(Xj−μj)(15)
where XR=[X1,X2,X3,…,Xi] are the random variables, and μi is the corresponding mean value of Xi.
Eq. (15) is equivalent to Eq. (16):
G(XR)=(G(μ)−n∑i=1∂G∂Xi|μμi+12n∑i=1n∑j=1∂2G∂Xi∂Xjμiμj)+n∑i=1(∂G∂Xi|μ+n∑j=1∂2G∂Xi∂Xj|μμj)Xi+12n∑i=1n∑j=1∂2G∂Xi∂XjXiXj(16)
Then, Y can be expressed as:
Y=G(XR)=XTDX+hTX+k(17)
where
{Dij=12∂2G∂Xi∂Xj|μhi=∂G∂Xi|μ+n∑j=1∂2G∂Xi∂Xj|μk=G(μ)−n∑i=1∂G∂Xi|μμi+12n∑i=1n∑j=1∂2G∂Xi∂Xj|μμiμj(18)
With the assumption that the random design variables XR follow a Gaussian distribution, μ is the mean value of XR and σ is the covariance of XR. The MGF of Y can be expressed as Eq. (19):
MY(t)=|H|−12et(μTDμ+hTμ+k)+t22dTH−1d(19)
where H=I−2tσ12Dσ12 and d=σ12h+2σ12Dμ.
According to the two properties of CGF mentioned in Section 3.1, the CGF of Y can be calculated using Eq. (20):
KY(t)=−12log|H|+t22dTH−1d+t(μTDμ+hTμ+k)(20)
Furthermore, the first derivative of Y is
K′Y(t)=−12trace(H−1H′)+μTDμ+hTμ+k+tdTH−1d−t22dTH−1H′H−1d(21)
and the second derivative of Y is
K″Y(t)=−12trace(H−1H′H−1H′)+2tdTH−1H′H−1d−t2dTH−1H′H−1H′H−1d.(22)
Then, the PDF of Y can be calculated using Eq. (3).
MVSOSA can also provide higher accuracy than MVFOSA and can be applied in a wide range of practical projects.
SATM is a method developed from general saddlepoint approximation [25]. The basic idea of SATM is introduced below.
When MG(t) exists, MG(0) is equal to 1, and MlG(0) is equal to E(Gl). M(l)G(t) means the lth derivative of MG(t), l=1,2,3,…,n.
Therefore, the first three derivatives of KG(t) are given as:
{K′G(0)=μGK″G(0)=σ2GK‴G(0)=α3G(23)
where μG is the mean, σ2G is the standard deviation, and α3G is the variance of G.
In addition, the standardized form of G can be calculated as GS=G−μGσG. The transformation is linear, ensuring that the nonlinearity of the limit-state function does not increase. This transformation maintains numerical stability in practical engineering. Then, Eq. (24) can be obtained:
{K′GS(0)=0K″GS(0)=1,α3GS=α3Gσ3GK‴GS(0)=α3GS(24)
Considering that the challenges of solving the saddlepoint equation and obtaining the CGF may impact the utilization of the saddlepoint method, a simplified version of the CGF KGS(t) for Gs can be formulated as follows:
KGS(t)=d1t+d2t2−d3ln[(1−et)2](25)
where d1, d2, and d3 are determined constants, and e is a prescribed value, which is usually given as e=α3GS2. The derivatives of KGS can be obtained using Eq. (26):
{K′GS(t)=d1+2d2t+2d3e1−etK″GS(t)=2d2+2d3e21−et2K‴GS(t)=4d3e2(1−et)3(26)
According to Eqs. (23) and (26), the following can be deduced:
{d1+2d3e=02d2+2d3e2=14d3e2=α3GS(27)
If α3GS=0, then d1=0, d2=0.5, and d3e=0. KGS(t) is the CGF of the standard normal variable. If α3GS≠0 and e≠0, then d1=−α3GS2b2, d2=12(1−α3GS2e), and d3e=α3GS4e3. Thus, K′GS(t) in Eq. (26) can be expressed as:
K′GS(t)=−α3GS2e2+1−α3GS2et+2α3GS4e3(1−et)(28)
The saddlepoint equation K′GS(t)=g can be denoted as:
(2e−α3GS)t2−2(1+eg)t+2g=0(29)
If the value of e is given, it will be simpler to obtain ˜t in SATM than in the conventional SAM. The PDF of GS can be approximately expressed in terms of ˜t:
fGS(g)=[2πK″GS(˜t)]−12eKGS(˜t)−˜tg(30)
The CDF of GS is similar to Eq. (4); it can be expressed as:
FG(g)=Pr[GS≤g]=Φ(wS)+φ(wS)(1wS−1vS)(31)
or
FG(g)=Pr[GS≤g]=Φ[wS+1wSlnvSwS](32)
where wS=sign(˜t){2[˜tg−KGS(˜t)]}12, and vS=˜t[K″GS(˜t)]12.
According to Eqs. (25) and (26), KGS(˜t) and K″GS(˜t) can be expressed as KGS(˜t)=d1˜t+d2˜t2−d3ln[(1−e˜t)2] and K″GS(˜t)=2d2+2d3e2(1−e˜t)2, respectively.
Furthermore, the PDF of G can be approximately obtained according to the linear relationship between GS and G as Eq. (33):
fG(g)=1√2πK″GS(˜t)σGeKGS(˜t)−˜t(g−μG)σG(33)
Then, the CDF of G can also be calculated using Eqs. (34) or (35):
FG(g)=Φ(we)+φ(we)(1we−1ve)(34)
or
FG(g)=Φ[we+1welnvewe](35)
where GS=G−μGσG, ge=g−μGσG, we=sign(˜te){2[˜tege−KGS(˜t)]}12, and ve=˜te[K″GS(˜te)]12. Substituting d1=−α3GS2e2, d2=0.5(1−α3GS2e), and d3e=α3GS4e3 into KGS(˜t)=d1˜t+d2˜t2−d3ln[(1−e˜t)2] and K″GS(˜t)=2d2+2d3e2(1−e˜t)2, then KGS(˜te) and K″GS(˜te) can be obtained asKGS(˜te)=−α3GS2b2˜te+12(1−α3GS2e2)˜te2−α3GS4e3ln[(1−e˜te)2](36)
K″GS(˜te)=(1−α3GS2e)+α3GS2e(1−e˜te)2(37)
According to the above equations, the CGF of G can be expressed as:
KG(t)=(μG−σGα3GS2b2)t+σ2G2(1−α3GS2e)t2−α3GS4e3ln[(1−eσGt)2](38)
Then, the CDF of G can be expressed as:
pf=FG(0)=Pr[GS≤−β]=Φ(wr)+φ(wr)(1wr−1vr)(39)
or
pf=FG(0)=Pr[GS≤−β]=Φ[wr+1wrlnvrwr](40)
where β=μGσG, wr=sign(˜tr){2[˜tr(−β)−KGS(˜tr)]}12, and vr=˜tr[K″GS(˜tr)]12.
As mentioned above, KG(t) can be obtained solely using the first three moments. Thus, SATM allows for the derivation of a CGF for a random variable following a specific distribution.
In contrast to SATM, SAFM is an enhanced high-order moment-based SAM [180]. According to Section 3.3 and Eq. (23), the fourth derivative of KG(t) can be expressed as
K(4)G(0)=E(G−E(G))4=η4G−3σ2G(41)
where η4G denotes the fourth central moment of G.
According to SATM, Eq. (25) can be used to express the CGF in terms of GS; then, the fourth derivative of KGS can be obtained using Eq. (42):
K(4)G(t)=12d3e2(1−et)4(42)
Then, Eq. (43) can be obtained using Eqs. (23) and (41):
{d1+2d3e=02d2+2d3e2=14d3e2=α3GS12d3e4=η4GS−3(43)
where η4GS=η4Gσ4G.
If α3GS=0, then d1=0, d2=0.5, d3e=0 and η4GS=3. KGS(t) can be obtained as KGS(t)=0.5t2. If α3GS≠0 and η4GS≠3, then d1=−9α33GS2(η4GS−3)2, d2=−3α33GS+2η4GS−64(η4GS−3), d3=27α43GS4(η4GS−3)3, and e=η4GS−33α3GS.
According to Eqs. (23), (41), and the saddlepoint equation K′GS(t)−g=0, the saddlepoint equation can be expressed as:
d1+2d2t+2d3e1−et−g=0(44)
The saddlepoint ˜t can be calculated by solving Eq. (44):
{˜t1=√(16d2d3+(g−d1)2)e2−4d2(g−d1)e+4d22+2d2+(g−d1)e4ed2˜t2=−√(16d2d3+(g−d1)2)e2−4d2(g−d1)e+4d22+2d2+(g−d1)e4ed2(45)
Then, substituting d1, d2, d3, and e into Eq. (25) and K″GS(t) in Eq. (26), KGS(t) and K″GS(t) can be expressed as:
KGS(˜te)=−3α33GSψ2˜t2e−27α43GSln((ψ˜te−3α3GS)29α23GS)+2ψ3˜t2e−18α33GSψ˜te4ψ3(46)
K″GS(˜te)=−3α33GS+2ψ2ψ+27α43GS2ψ(ψ˜te−3α3GS)2(47)
where ψ=η4GS−3.
According to Section 2, the saddlepoint approximation to the CDF of G can be expressed in the same form as Eq. (35). Consequently, the failure probability of the structure with G can be expressed as:
pf=FG(0)=Pr[GS≤−β2]=Φ[wg+1wglnvgwg](48)
where β2=μGσG, wg=sign(˜tg){2[˜tg(−β2)−KGS(˜tg)]}12 and vg=˜tg[K″GS(˜tg)]12. ˜tg is a saddlepoint determined by Eq. (45); it can be expressed as:
˜tg=[√(16d2d3+(β2+d1)2)e2+4d2(β2+d1)e+4d22−(β2+d1)e+2d24ed2,×−√(16d2d3+(β2+d1)2)e2+4d2(β2+d1)e+4d22−(β2+d1)b+2d24ed2](49)
When g=0, the failure probability of the random structure is estimated at the mean of the distribution of G; the CDF can be expressed as:
FG(0)=Pr[GS≤0]=12+K‴G(0)6√2π(50)
Under the same condition, Eq. (51) can be applied as an alternative method to estimate the failure probability:
Pf=Pr[G≤μG]=Pr[GS≤0]=12+α3GS6√2π(51)
In SAFM, the first four stochastic moments form the foundation for approximating the CGF. Employing the general SAM enables the assessment of the failure probability in a random structure using the approximated CGF. Notably, SAFM offers enhanced accuracy compared with the conventional SAM.
4 Improved SAMs in Application
As previously mentioned, the demand for reliability in modern engineering design is constantly increasing. To provide readers with a better understanding of the specific engineering applications and the value of the aforementioned method, this section presents three engineering-related examples. These examples encompass all of the methods in Section 3, and some related methods are presented for comparison. The comparison within these engineering cases will highlight the effectiveness of the improved SAMs.
4.1 Reliability-Based Optimization for Offshore Structures
The wellhead platform represents an economical solution for offshore oilfield development, providing a provisional structure that safeguards the wellhead situated on the seabed.
In the given context, wave force-induced damage is the primary factor influencing RA. The core principle of RBDO involves constructing a probabilistic model to encapsulate inherent uncertainties in engineering structures. The RBDO methodology allows for addressing prevalent uncertainties in engineering applications, ensuring that the design aligns with the project’s specified reliability criteria. Fig. 9 illustrates the concept diagram of the wellhead platform’s appearance, while Fig. 10 offers a specific schematic. Table 2 presents the random design parameters in this case [179]. According to the optimization results in Table 3, the optimized volume can be calculated. The minimum volume obtained through RBDO-MVSOSA calculation is 15.37 m3, which is 9% smaller than that obtained using the initial scheme, and it is the best result. These results indicate that RBDO with improved SAMs generates acceptable optimization schemes. Comparative data reveals that RBDO-MVSOSA tends to produce more conservative results than RBDO-MVFOSA, aligning closer with the results from RBDO-Monte Carlo simulation (MCS).
Figure 9: Concept diagram of the wellhead platform
Figure 10: Illustration of a wellhead platform
This example demonstrates that RBDO-MVSOSA offers superior accuracy and that enhanced SAMs positively influence reliability-related issues.
4.2 Failure Probability Evaluation of Reinforced Concrete Beam
Reinforced concrete beams, pivotal load-bearing elements in engineering structures such as buildings and bridges, have widespread applications [181]. Therefore, their reliability is paramount. Specific data for this example are available in reference [180]. The schematic of a reinforced concrete beam is depicted in Fig. 11.
Figure 11: Reinforced concrete beam
The performance function of the concrete beam model can be expressed as Eq. (52):
G=Sxσxσpxh−0.59S2xσ2xσpxd−W(52)
where Sx represents the area of the reinforcing cross section, σx represents the steel yield strength, σpx represents the concrete compressive strength, and W represents the total moment produced by the load. The width and height of the beam are d=12in and h=19in, respectively.
The distributions of input random variables are shown in Table 4:
The first four moments of the state variable are shown in Eq. (53):
{μG=816.57σG=397.54α3G=0.059η4G=3.095(53)
The results of the failure probability estimate using the different improved SAMs and MCS are shown in Table 5.
According to the aforementioned results, both SATM and SAFM offer failure probability estimates that closely align with the results of MCS. This suggests that the refined approximation methods employed in this study yield high precision. Fig. 11 presents the comparison of estimated CDF tails derived from different techniques.
According to the results of the comparison, the closer the tail line in the figure is to MCS, the higher the accuracy of the method. According to the data in Fig. 12, the CDF tail estimated by SAFM matches the results of MCS better than the CDF tail estimated by SATM, while SATM can also provide acceptable results. In Table 4, Pf denotes the failure probability calculated by different methods. Compared with SATM, SAFM yields Pf closer to the MCS results.
Figure 12: Comparison of the CDF tails derived from different methods
This example demonstrates the high practicality of both SATM and SAFM in addressing reliability issues in engineering.
4.3 Reliability Evaluation of a Roof Structure
The schematic diagram of a typical roof structure in civil construction is shown in Fig. 13, and its simplified force analysis diagram is shown in Fig. 14.
Figure 13: Roof structure
Figure 14: Simplified force analysis of the roof structure
The upper boom and compression bars are made of concrete, while the lower boom and tension bars are constructed from steel. The vertical deflection of the rooftop’s apex node C can be computed using Eq. (54):
ΔC=(3.81SCEC+1.13SXEX)ql22(54)
where SC and SX are the cross-sectional area of the concrete bars and steel bars, respectively. EC and EX are the elastic moduli of the concrete bars and steel bars, respectively.
There are three limit-state functions generated by different conditions of the structure:
{g1(X)=(3.81SCEC+1.13SXEX)ql22−0.015g2(X)=1.185ql−fCSCg3(X)=0.65ql−fSSX(55)
The first failure mode occurs when the perpendicular deflection ΔC exceeds 1.5 cm. The second failure mode occurs when the internal force of bar AD surpasses its own limiting stress. The third failure mode occurs when the internal force of bar EC exceeds its respective limit stress. fS denotes the tensile strength of the bar. Specific setting parameters can be found in reference [154].
Table 6 outlines the system failure probability estimated by SOSA and other methodologies.
With the MCS solution as the benchmark, SOSA demonstrates superior accuracy and efficiency, emphasizing the applicability of saddlepoint approximation in engineering contexts.
This study comprehensively reviews SAM, explores its enhancement techniques, and provides examples showcasing each augmented SAM in RA. Following these examples, the merits of each method are critically evaluated. The primary goal of SAM is to provide efficient and precise fitting results in RA. Its foundational principles establish a groundwork for effective fitting, adaptable to further refinement based on specific operational needs. RA often involves extensive datasets, posing significant challenges. Remarkably, the results of SAM with minimal sample sizes closely resemble those obtained via MCS with larger sample sizes, indicating SAM’s capability to provide superior approximations even with limited data. Analyzing large-scale samples significantly increases time and financial investments. SAM’s efficiency alleviates substantial operational demands and expedites problem-solving in reliability analysis. However, with the evolving landscape of contemporary engineering, systems requiring RA are growing in size and complexity. An increasing number of uncertainties are being incorporated to ensure the reliability and robustness of extensive mechanical systems. Consequently, achieving a balance between sampling efficiency and reliability is becoming progressively challenging.
In RBDO, challenges arise when the dimensionality of the input information becomes excessively high. Integrating RBDO with saddlepoint approximation removes the need for the spatial transformation of random variables. This approach mitigates potential inaccuracies in reliability assessment caused by amplified nonlinearity in the limit-state function, thereby enhancing the attractiveness of SAM. Presently, the engineering field associated with reliability increasingly tends to adopt machine learning and similar techniques. Utilizing machine learning algorithms enables engineers to construct models derived from data, thereby facilitating predictive analyses and informed decision-making.
The theoretical foundation and validation of SAM remain a dynamic field for future exploration. Its mathematical properties, convergence, and method stability offer significant research value. Additionally, integrating saddlepoint approximation with machine learning models can improve solution accuracy and efficacy. SAM holds potential for utilization in deep neural network (DNN) training. The training processes of DNNs are susceptible to encountering numerous local minima interspersed with saddlepoints, complicating the convergence to a global optimum. SAM can address these saddlepoint challenges during training. SAM involves an effective search in the saddlepoint area to find the saddlepoint and continuous optimization until the global minimum is found. Consequently, SAM can more effectively train DNNs, improving both model accuracy and generalization capability. Integrating saddlepoint approximation into conventional machine learning algorithms, such as support vector machines, logistic regression, and decision trees, enhances model optimization and predictive performance. The amalgamation of SAMs with genetic algorithms is a promising research area. Genetic algorithms, rooted in evolutionary principles, leverage mechanisms such as crossover, mutation, and selection for optimization. Their adeptness at global searching compensates for SAM’s tendency to favor local optimization in high-dimensional spaces. Moreover, genetic algorithms can fine-tune relevant parameters within SAM, enhancing its convergence rate and stability. In essence, combining SAM with these methodologies amplifies its effectiveness in addressing challenges within RA and RBDO.
Acknowledgement: The authors extend their sincere gratitude to the reviewers for their valuable suggestions, which have significantly enhanced the quality of this paper. We also express our heartfelt thanks to the editors for their patience, amiable guidance, and diligent efforts in refining the manuscript.
Funding Statement: This research was funded by the National Natural Science Foundation of China under Grant No. 52175130, the Sichuan Science and Technology Program under Grants Nos. 2022YFQ0087 and 2022JDJQ0024, the Guangdong Basic and Applied Basic Research Foundation under Grant No. 2022A1515240010, and the Students Go Abroad for Scientific Research and Internship Funding Program of University of Electronic Science and Technology of China.
Author Contributions: The authors confirm contribution to the paper as follows: Study conception and design: Debiao Meng, Shiyuan Yang, Yipeng Guo; data collection and analysis: Yihe Xu, Yongqiang Guo; collection and arrangement of references: Lidong Pan, Xinkai Guo; draft manuscript preparation: Debiao Meng, Yipeng Guo. All authors reviewed the results and approved the final version of the manuscript.
Availability of Data and Materials: All data presented in this paper can reasonably be obtained from the relevant references or by contacting the corresponding author of this paper.
Conflicts of Interest: The authors declare that they have no known competing financial interests or personal relationships that could have appeared to influence the work reported in this article.
References
1. Daniels, H. E. (1954). Saddlepoint approximations in statistics. The Annals of Mathematical Statistics, 25(4), 631–650. [Google Scholar]
2. Benzi, M., Golub, G. H. (2004). A preconditioner for generalized saddlepoint problems. SIAM Journal on Matrix Analysis and Applications, 26(1), 20–41. [Google Scholar]
3. Dem’yanov, V. F., Pevnyi, A. B. (1972). Numerical methods for finding saddlepoints. USSR Computational Mathematics and Mathematical Physics, 12(5), 11–52. [Google Scholar]
4. Robert, L., Stephen, R. (1980). Saddlepoint approximation for the distribution of the sum of independent random variables. Advances in Applied Probability, 12(2), 475–490. [Google Scholar]
5. Huang, K., Zhang, J., Zhang, S. (2022). Cubic regularized Newton method for the saddlepoint models: A global and local convergence analysis. Journal of Scientific Computing, 91(2), 60. [Google Scholar]
6. Barndorff-Nielsen, O., Cox, D. R. (1979). Edgeworth and saddlepoint approximations with statistical applications. Journal of the Royal Statistical Society: Series B (Methodological), 41(3), 279–299. [Google Scholar]
7. Goutis, C., Casella, G. (1999). Explaining the saddlepoint approximation. The American Statistician, 53(3), 216–224. [Google Scholar]
8. Benzi, M., Golub, G. H., Liesen, J. (2005). Numerical solution of saddlepoint problems. ACTA Numerical, 14, 1–137. [Google Scholar]
9. Adolphs, L., Daneshmand, H., Lucchi, A., Hofmann, T. (2019). Local saddlepoint optimization: A curvature exploitation approach. International Conference on Artificial Intelligence and Statistics, 89, 486–495. [Google Scholar]
10. Beznosikov, A., Scutari, G., Rogozin, A., Gasnikov, A. (2021). Distributed saddlepoint problems under data similarity. Advances in Neural Information Processing Systems, 34, 8172–8184. [Google Scholar]
11. Zhang, J., Hong, M., Zhang, S. (2022). On lower iteration complexity bounds for the convex concave saddlepoint problems. Mathematical Programming, 194(1–2), 901–935. [Google Scholar]
12. Zhao, R. (2022). Accelerated stochastic algorithms for convex-concave saddlepoint problems. Mathematics of Operations Research, 47(2), 1443–1473. [Google Scholar]
13. Gatica, G. N., Heuer, N., Meddahi, S. (2003). On the numerical analysis of nonlinear twofold saddlepoint problems. IMA Journal of Numerical Analysis, 23(2), 301–330. [Google Scholar]
14. Hu, Z., Du, X. (2018). Saddlepoint approximation reliability method for quadratic functions in normal variables. Structural Safety, 71, 24–32. [Google Scholar]
15. Huang, B., Du, X. (2008). Probabilistic uncertainty analysis by mean-value first order saddlepoint approximation. Reliability Engineering and System Safety, 93(2), 325–336. [Google Scholar]
16. Chen, J., Chen, L., Qian, L., Chen, G., Zhou, S. (2022). Time-dependent kinematic reliability analysis of gear mechanism based on sequential decoupling strategy and saddlepoint approximation. Reliability Engineering & System Safety, 220, 108292. [Google Scholar]
17. Valyi, I. (1987). Approximate saddlepoint theorems in vector optimization. Journal of Optimization Theory and Applications, 55, 435–448. [Google Scholar]
18. Xiao, N., Huang, H., Wang, Z., Liu, Y., Zhang, X. (2012). Unified uncertainty analysis by the mean value first order saddlepoint approximation. Structural and Multidisciplinary Optimization, 46(6), 803–812. [Google Scholar]
19. Zulehner, W. (2002). Analysis of iterative methods for saddlepoint problems: A unified approach. Mathematics of Computation, 71(238), 479–505. [Google Scholar]
20. Sun, X., Teo, K., Zeng, J., Guo, X. (2020). On approximate solutions and saddlepoint theorems for robust convex optimization. Optimization Letters, 14, 1711–1730. [Google Scholar]
21. Chung, S., Butler, R. W., Scharf, L. L., Chapman, P. L., Hoeting, J. A. (2010). Saddlepoint approximation to functional equations in queueing theory and insurance mathematics (Doctoral Dissertation). Colorado State University, USA. [Google Scholar]
22. Na, J. (2016). Saddlepoint approximations for the risk measures of linear portfolios based on generalized hyperbolic distributions. Journal of the Korean Data and Information Science Society, 27(4), 959–967. [Google Scholar]
23. Chen, H., Han, L., Lim, A. (2023). Estimating linear mixed effect models with non-normal random effects through saddlepoint approximation and its application in retail pricing analytics. Journal of Applied Statistics, 1–23. https://doi.org/10.1080/02664763.2023.2260576 [Google Scholar] [CrossRef]
24. Cattaneo, L., Formaggia, L., Iori, G. F., Scotti, A., Zunino, P. (2015). Stabilized extended finite elements for the approximation of saddlepoint problems with unfitted interfaces. Calcolo, 52, 123–152. [Google Scholar]
25. Yuan, R., Meng, D., Li, H. (2016). Multidisciplinary reliability design optimization using an enhanced saddlepoint approximation in the framework of sequential optimization and reliability analysis. Proceedings of the Institution of Mechanical Engineers, Part O: Journal of Risk and Reliability, 230(6), 570–578. [Google Scholar]
26. Apostolakis, G. (1990). The concept of probability in safety assessments of technological systems. Science, 250(4986), 1359–1364. [Google Scholar] [PubMed]
27. Rackwitz, R. (2001). Reliability analysis–A review and some perspectives. Structural Safety, 23(4), 365–395. [Google Scholar]
28. Zio, E. (2009). Reliability engineering: Old problems and new challenges. Reliability Engineering & System Safety, 94(2), 125–141. [Google Scholar]
29. Zhu, S. P., Liu, Q., Lei, Q., Wang, Q. (2018). Probabilistic fatigue life prediction and reliability assessment of a high pressure turbine disc considering load variations. International Journal of Damage Mechanics, 27(10), 1569–1588. [Google Scholar]
30. Liu, X., Gong, M., Zhou, Z., Xie, J., Wu, W. (2021). An improved first order approximate reliability analysis method for uncertain structures based on evidence theory. Mechanics Based Design of Structures and Machines, 51(7), 4137–4154. [Google Scholar]
31. Yu, S., Li, Y. (2021). Active learning kriging model with adaptive uniform design for time-dependent reliability analysis. IEEE Access, 9, 91625–91634. [Google Scholar]
32. Yu, S., Wang, Z., Li, Y. (2022). Time and space-variant system reliability analysis through adaptive Kriging and weighted sampling. Mechanical Systems and Signal Processing, 166, 108443. [Google Scholar]
33. Modibbo, U. M., Arshad, M., Abdalghani, O., Ali, I. (2021). Optimization and estimation in system reliability allocation problem. Reliability Engineering & System Safety, 212, 107620. [Google Scholar]
34. Zhao, D., Yu, S., Wang, Z., Wu, J. (2021). A box moments approach for the time-variant hybrid reliability assessment. Structural and Multidisciplinary Optimization, 64(6), 4045–4063. [Google Scholar]
35. Li, W., Gao, L., Xiao, M. (2020). Multidisciplinary robust design optimization under parameter and model uncertainties. Engineering Optimization, 52(3), 426–445. [Google Scholar]
36. Meng, D., Yang, S., He, C., Wang, H., Lv, Z. et al. (2022). Multidisciplinary design optimization of engineering systems under uncertainty: A review. International Journal of Structural Integrity, 13(4),565–593. [Google Scholar]
37. Afshari, S. S., Enayatollahi, F., Xu, X., Liang, X. (2022). Machine learning-based methods in structural reliability analysis: A review. Reliability Engineering & System Safety, 219, 108223. [Google Scholar]
38. Iannacone, L., Sharma, N., Tabandeh, A., Gardoni, P. (2022). Modeling time-varying reliability and resilience of deteriorating infrastructure. Reliability Engineering & System Safety, 217, 108074. [Google Scholar]
39. Bruton, A., Conway, J. H., Holgate, S. T. (2000). Reliability: What is it, and how is it measured? Physiotherapy, 86(2), 94–99. [Google Scholar]
40. Meng, D., Yang, S., de Jesus, A. M., Zhu, S. P. (2023). A novel Kriging-model-assisted reliability-based multidisciplinary design optimization strategy and its application in the offshore wind turbine tower. Renewable Energy, 203, 407–420. [Google Scholar]
41. Zhang, T. (2017). An improved high-moment method for reliability analysis. Structural and Multidisciplinary Optimization, 56, 1225–1232. [Google Scholar]
42. Zhao, Y. G., Ono, T. (2001). Moment methods for structural reliability. Structural Safety, 23(1), 47–75. [Google Scholar]
43. Melchers, R. E., Beck, A. T. (2018). Structural reliability analysis and prediction. John Wiley & Sons, The Atrium, Southern Gate, Chichester, West Sussex, UK. [Google Scholar]
44. Rahman, S., Wei, D. (2006). A univariate approximation at most probable point for higher-order reliability analysis. International Journal of Solids and Structures, 43(9), 2820–2839. [Google Scholar]
45. Tabandeh, A., Jia, G., Gardoni, P. (2022). A review and assessment of importance sampling methods for reliability analysis. Structural Safety, 97, 102216. [Google Scholar]
46. Cardoso, J. B., de Almeida, J. R., Dias, J. M., Coelho, P. G. (2008). Structural reliability analysis using Monte Carlo simulation and neural networks. Advances in Engineering Software, 39(6), 505–513. [Google Scholar]
47. Zhang, L., Lu, Z., Wang, P. (2015). Efficient structural reliability analysis method based on advanced Kriging model. Applied Mathematical Modelling, 39(2), 781–793. [Google Scholar]
48. Shi, Y., Lu, Z., Chen, S., Xu, L. (2018). A reliability analysis method based on analytical expressions of the first four moments of the surrogate model of the performance function. Mechanical Systems and Signal Processing, 111, 47–67. [Google Scholar]
49. Zhang, C., Song, L., Fei, C., Lu, C., Xie, Y. (2016). Advanced multiple response surface method of sensitivity analysis for turbine blisk reliability with multi-physics coupling. Chinese Journal of Aeronautics, 29(4), 962–971. [Google Scholar]
50. Wei, Y., Bai, G., Song, L., Bai, B. (2019). The estimation of reliability probability of structures based on improved iterative response surface methods. KSCE Journal of Civil Engineering, 23(9), 4063–4074. [Google Scholar]
51. Meng, Z., Zhang, D., Li, G., Yu, B. (2019). An importance learning method for non-probabilistic reliability analysis and optimization. Structural and Multidisciplinary Optimization, 59, 1255–1271. [Google Scholar]
52. Zhang, D., Han, X., Jiang, C., Liu, J., Li, Q. (2017). Time-dependent reliability analysis through response surface method. Journal of Mechanical Design, 139(4), 041404. [Google Scholar]
53. Der Kiureghian, A., Song, J. (2008). Multi-scale reliability analysis and updating of complex systems by use of linear programming. Reliability Engineering & System Safety, 93(2), 288–297. [Google Scholar]
54. Yuan, Y., Jiang, X., Ai, Q. (2017). Probabilistic assessment for concrete spalling in tunnel structures. ASCE-ASME Journal of Risk and Uncertainty in Engineering Systems, Part A: Civil Engineering, 3(4), 04017011. [Google Scholar]
55. Lai, X., Huang, J., Zhang, Y., Wang, C., Zhang, X. (2022). A general methodology for reliability-based robust design optimization of computation-intensive engineering problems. Journal of Computational Design and Engineering, 9(5), 2151–2169. [Google Scholar]
56. Zhang, H., Song, L., Bai, G. (2023). Active kriging-based adaptive importance sampling for reliability and sensitivity analyses of stator blade regulator. Computer Modeling in Engineering & Sciences, 134(3), 1871–1897. https://doi.org/10.32604/cmes.2022.021880 [Google Scholar] [CrossRef]
57. Gao, X., Su, X., Qian, H., Pan, X. (2022). Dependence assessment in human reliability analysis under uncertain and dynamic situations. Nuclear Engineering and Technology, 54(3), 948–958. [Google Scholar]
58. Rajashekhar, M. R., Ellingwood, B. R. (1993). A new look at the response surface approach for reliability analysis. Structural Safety, 12(3), 205–220. [Google Scholar]
59. Yang, S., Meng, D., Wang, H., Yang, C. (2023). A novel learning function for adaptive surrogate-model-based reliability evaluation. Philosophical Transactions of the Royal Society A, 381, 20220395. [Google Scholar]
60. Zhou, X., Deng, X., Deng, Y., Mahadevan, S. (2017). Dependence assessment in human reliability analysis based on D numbers and AHP. Nuclear Engineering and Design, 313, 243–252. [Google Scholar]
61. Pang, Y., Lai, X., Zhang, S., Wang, Y., Yang, L. et al. (2023). A Kriging-assisted global reliability-based design optimization algorithm with a reliability-constrained expected improvement. Applied Mathematical Modelling, 121, 611–630. [Google Scholar]
62. Dong, Z., Sheng, Z., Zhao, Y., Zhi, P. (2023). Robust optimization design method for structural reliability based on active-learning MPA-BP neural network. International Journal of Structural Integrity, 14(2), 248–266. [Google Scholar]
63. Zhang, J., Wang, H., Peng, J. (2012). Initiation time model and its reliability analysis for concrete structures under multi-corrosive factors. Journal of Changsha University of Science & Technology (Natural Science), 9(1), 34–40. [Google Scholar]
64. Liu, X., Zhang, Y. (2021). Reliability-based design optimization for vehicle structural crash worthiness based on hybrid model. Journal of Changsha University of Science & Technology (Natural Science), 18(1), 95–101. [Google Scholar]
65. Chen, J., Zhang, J. (2004). The reliability assessment of existing bridge based on neural network and response surface method. Journal of Changsha University of Science & Technology (Natural Science), 1(3,4), 13–17+22 (In Chinese). [Google Scholar]
66. Yang, S., Meng, D., Wang, H., Chen, Z., Xu, B. (2023). A comparative study for adaptive surrogate-model-based reliability evaluation method of automobile components. International Journal of Structural Integrity, 14(3), 498–519. [Google Scholar]
67. Zhi, P., Xu, Y., Chen, B. (2019). Time-dependent reliability analysis of the motor hanger for EMU based on stochastic process. International Journal of Structural Integrity, 11(3), 453–469. [Google Scholar]
68. Mahadevan, S., Raghothamachar, P. (2000). Adaptive simulation for system reliability analysis of large structures. Computers & Structures, 77(6), 725–734. [Google Scholar]
69. Deng, J., Gu, D., Li, X., Yue, Z. Q. (2005). Structural reliability analysis for implicit performance functions using artificial neural network. Structural Safety, 27(1), 25–48. [Google Scholar]
70. Deng, J. (2006). Structural reliability analysis for implicit performance function using radial basis function network. International Journal of Solids and Structures, 43(11–12), 3255–3291. [Google Scholar]
71. Meng, D., Wang, H., Yang, S., Lv, Z., Hu, Z. et al. (2022). Fault analysis of wind power rolling bearing based on EMD feature extraction. Computer Modeling in Engineering & Sciences, 130(1), 543–558. https://doi.org/10.32604/cmes.2022.018123 [Google Scholar] [CrossRef]
72. Manouchehry Nya, R., Abdullah, S., Singh Karam Singh, S. (2019). Reliability-based fatigue life of vehicle spring under random loading. International Journal of Structural Integrity, 10(5), 737–748. [Google Scholar]
73. Bing, L., Meilin, Z., Kai, X. (2000). A practical engineering method for fuzzy reliability analysis of mechanical structures. Reliability Engineering & System Safety, 67(3), 311–315. [Google Scholar]
74. Macchi, M., Garetti, M., Centrone, D., Fumagalli, L., Pavirani, G. P. (2012). Maintenance management of railway infrastructures based on reliability analysis. Reliability Engineering & System Safety, 104, 71–83. [Google Scholar]
75. Yang, C., Cao, Q., Zeng, N. (2022). Reliability research of service structure based on improved conditional probability method. Journal of Changsha University of Science & Technology (Natural Science), 19(3), 78–86 (In Chinese). [Google Scholar]
76. Tong, D., Farnham, D. J., Duan, L., Zhang, Q., Lewis, N. S. et al. (2021). Geophysical constraints on the reliability of solar and wind power worldwide. Nature Communications, 12(1), 6146. [Google Scholar]
77. Zhao, Y. G., Ono, T. (2000). Third-moment standardization for structural reliability analysis. Journal of Structural Engineering, 126(6), 724–732. [Google Scholar]
78. Blanchard, B. S., Fabrycky, W. J., Fabrycky, W. J. (1990). Systems engineering and analysis, vol. 4. Englewood Cliffs, NJ: Prentice Hall. [Google Scholar]
79. Gertsbakh, I. B. (2000). Reliability theory: With applications to preventive maintenance. Springer-Verlag Berlin Heidelberg, New York: Springer Science & Business Media. [Google Scholar]
80. Li, H., Soares, C. G., Huang, H. Z. (2020). Reliability analysis of a floating offshore wind turbine using Bayesian networks. Ocean Engineering, 217, 107827. [Google Scholar]
81. Förstner, W. (1987). Reliability analysis of parameter estimation in linear models with applications to mensuration problems in computer vision. Computer Vision, Graphics, and Image Processing, 40(3),273–310. [Google Scholar]
82. Ahmed, K. M. U., Bollen, M. H., Alvarez, M. (2021). A review of data centers energy consumption and reliability modeling. IEEE Access, 9, 152536–152563. [Google Scholar]
83. Peyghami, S., Blaabjerg, F., Palensky, P. (2020). Incorporating power electronic converters reliability into modern power system reliability analysis. IEEE Journal of Emerging and Selected Topics in Power Electronics, 9(2), 1668–1681. [Google Scholar]
84. Xing, L., Johnson, B. W. (2022). Reliability theory and practice for unmanned aerial vehicles. IEEE Internet of Things Journal, 10(4), 3548–3566. [Google Scholar]
85. Yang, W., Yang, Y., Jiang, B. (2009). Reliability analysis of RC members subjected to eccentric compression based on random characteristics of eccentricity. Journal of Changsha University of Science & Technology (Natural Science), 6(4), 11–15 (In Chinese). [Google Scholar]
86. Duchesne, L., Karangelos, E., Wehenkel, L. (2020). Recent developments in machine learning for energy systems reliability management. Proceedings of the IEEE, 108(9), 1656–1676. [Google Scholar]
87. Hurtado, J. E. (2007). Filtered importance sampling with support vector margin: A powerful method for structural reliability analysis. Structural Safety, 29(1), 2–15. [Google Scholar]
88. Cheng, J., Li, Q. S. (2008). Reliability analysis of structures using artificial neural network based genetic algorithms. Computer Methods in Applied Mechanics and Engineering, 197(45–48), 3742–3750. [Google Scholar]
89. Guo, Z., Bai, G. (2009). Application of least squares support vector machine for regression to reliability analysis. Chinese Journal of Aeronautics, 22(2), 160–166. [Google Scholar]
90. Nabian, M. A., Meidani, H. (2018). Deep learning for accelerated seismic reliability analysis of transportation networks. Computer-Aided Civil and Infrastructure Engineering, 33(6), 443–458. [Google Scholar]
91. Yang, D. (2010). Chaos control for numerical instability of first order reliability method. Communications in Nonlinear Science and Numerical Simulation, 15(10), 3131–3141. [Google Scholar]
92. Hasofer, A. M., Lind, N. C. (1974). Exact and invariant second-moment code format. Journal of the Engineering Mechanics Division, 100(1), 111–121. [Google Scholar]
93. Lu, Z., Song, J., Song, S., Yue, Z. F., Wang, J. (2010). Reliability sensitivity by method of moments. Applied Mathematical Modelling, 34(10), 2860–2871. [Google Scholar]
94. Zhong, C., Wang, M., Dang, C., Ke, W., Guo, S. (2020). First-order reliability method based on Harris Hawks Optimization for high-dimensional reliability analysis. Structural and Multidisciplinary Optimization, 62, 1951–1968. [Google Scholar]
95. Periçaro, G. A., Santos, S. R., Ribeiro, A. A., Matioli, L. C. (2015). HLRF-BFGS optimization algorithm for structural reliability. Applied Mathematical Modelling, 39(7), 2025–2035. [Google Scholar]
96. Shin, J., Lee, I. (2015). Reliability analysis and reliability-based design optimization of roadway horizontal curves using a first-order reliability method. Engineering Optimization, 47(5), 622–641. [Google Scholar]
97. Sankararaman, S., Daigle, M. J., Goebel, K. (2014). Uncertainty quantification in remaining useful life prediction using first-order reliability methods. IEEE Transactions on Reliability, 63(2), 603–619. [Google Scholar]
98. Alibrandi, U., Koh, C. G. (2015). First-order reliability method for structural reliability analysis in the presence of random and interval variables. ASCE-ASME Journal of Risk and Uncertainty in Engineering Systems, Part B: Mechanical Engineering, 1(4), 041006. [Google Scholar]
99. Choi, C. K., Yoo, H. H. (2012). Uncertainty analysis of nonlinear systems employing the first-order reliability method. Journal of Mechanical Science and Technology, 26, 39–44. [Google Scholar]
100. Du, X. (2008). Unified uncertainty analysis by the first order reliability method. Journal of Mechanical Design, 130(9), 1404–1404. [Google Scholar]
101. Alibrandi, U., Koh, C. G. (2017). Stochastic dynamic analysis of floating production systems using the first order reliability method and the secant hyperplane method. Ocean Engineering, 137, 68–77. [Google Scholar]
102. Fu, X., Guo, Q., Sun, H., Zhang, X., Wang, L. (2017). Estimation of the failure probability of an integrated energy system based on the first order reliability method. Energy, 134, 1068–1078. [Google Scholar]
103. Xiang, Y., Liu, Y. (2011). Application of inverse first-order reliability method for probabilistic fatigue life prediction. Probabilistic Engineering Mechanics, 26(2), 148–156. [Google Scholar]
104. Hao, P., Wang, Y., Ma, R., Liu, H., Wang, B. et al. (2019). A new reliability-based design optimization framework using isogeometric analysis. Computer Methods in Applied Mechanics and Engineering, 345, 476–501. [Google Scholar]
105. Huang, H. Z., Yu, H., Zhang, X., Zeng, S., Wang, Z. (2010). Collaborative optimization with inverse reliability for multidisciplinary systems uncertainty analysis. Engineering Optimization, 42(8), 763–773. [Google Scholar]
106. Chen, Z., Wu, Z., Li, X., Chen, G., Chen, G. et al. (2019). An accuracy analysis method for first-order reliability method. Proceedings of the Institution of Mechanical Engineers, Part C: Journal of Mechanical Engineering Science, 233(12), 4319–4327. [Google Scholar]
107. Kang, B., Mun, J., Lim, J., Choi, K. K., Kim, D. H. (2019). Most probable failure point update method for accurate first-order reliability-based electromagnetic designs. Journal of Magnetics, 24(3), 408–412. [Google Scholar]
108. He, R., Xu, Z., Yuan, F., Xiao, Z. (2013). First order reliability method of plane strain based on Mohr-Coulomb criterion. Electronic Journal of Geotechnical Engineering, 18, 1817–1830. [Google Scholar]
109. Keshtegar, B., Meng, Z. (2017). A hybrid relaxed first-order reliability method for efficient structural reliability analysis. Structural Safety, 66, 84–93. [Google Scholar]
110. Meng, Z., Li, G., Yang, D., Zhan, L. (2017). A new directional stability transformation method of chaos control for first order reliability analysis. Structural and Multidisciplinary Optimization, 55, 601–612. [Google Scholar]
111. Sudret, B., Der Kiureghian, A. (2002). Comparison of finite element reliability methods. Probabilistic Engineering Mechanics, 17(4), 337–348. [Google Scholar]
112. Sundararajan, C. (1995). Probabilistic structural mechanics handbook: Theory and industrial applications. New York: Springer. [Google Scholar]
113. Lopez, R. H., Beck, A. T. (2012). Reliability-based design optimization strategies based on FORM: A review. Journal of the Brazilian Society of Mechanical Sciences and Engineering, 34, 506–514. [Google Scholar]
114. Hohenbichler, M., Rackwitz, R. (1982). First-order concepts in system reliability. Structural Safety, 1(3), 177–188. [Google Scholar]
115. Pereda, L. A. (2015). Reliability and sensitivity analysis for micro-meteoroids and orbital debris protection models using first-order reliability methods (FORM). El Paso, Texas, USA: The University of Texas at El Paso. [Google Scholar]
116. Zhu, Z., Du, X. (2016). Reliability analysis with Monte Carlo simulation and dependent Kriging predictions. Journal of Mechanical Design, 138(12), 121403. [Google Scholar]
117. Li, M., Wang, Z. (2019). Surrogate model uncertainty quantification for reliability-based design optimization. Reliability Engineering & System Safety, 192, 106432. [Google Scholar]
118. Mínguez, R., Castillo, E. (2009). Reliability-based optimization in engineering using decomposition techniques and FORMS. Structural Safety, 31(3), 214–223. [Google Scholar]
119. Zhang, J., Du, X. (2010). A Second-order reliability method with first-order efficiency. Journal of Mechanical Design, 132(10), 101006. [Google Scholar]
120. Breitung, K. (1984). Asymptotic approximations for multinormal integrals. Journal of Engineering Mechanics, 110(3), 357–366. [Google Scholar]
121. Tvedt, L. (1990). Distribution of quadratic forms in normal space-application to structural reliability. Journal of Engineering Mechanics, 116(6), 1183–1197. [Google Scholar]
122. Der Kiureghian, A., Lin, H. Z., Hwang, S. J. (1987). Second-order reliability approximations. Journal of Engineering Mechanics, 113(8), 1208–1225. [Google Scholar]
123. Hohenbichler, M., Rackwitz, R. (1988). Improvement of second-order reliability estimates by importance sampling. Journal of Engineering Mechanics, 114(12), 2195–2199. [Google Scholar]
124. Kiureghian, A. D., Stefano, M. D. (1991). Efficient algorithm for second-order reliability analysis. Journal of Engineering Mechanics, 117(12), 2904–2923. [Google Scholar]
125. Köylüoǧlu, H. U., Søren, R. K. (1994). New approximations for SORM integrals. Structural Safety, 13(4), 235–246. [Google Scholar]
126. Cai, G. Q., Elishakoff, I. (1994). Refined second-order reliability analysis. Structural Safety, 14(4),267–276. [Google Scholar]
127. Su, Y., Li, S., Liu, S., Fang, Y. (2019). Extensive second-order method for reliability analysis of complicated geotechnical structures. European Journal of Environmental and Civil Engineering, 23(10), 1203–1221. [Google Scholar]
128. Adhikari, S. (2004). Reliability analysis using parabolic failure surface approximation. Journal of Engineering Mechanics, 130(12), 1407–1427. [Google Scholar]
129. Zhao, Y. G. (1999). New approximations for SORM: Part 2. Journal of Engineering Mechanics, 125(1), 86–93. [Google Scholar]
130. Lu, Z. H., Hu, D. Z., Zhao, Y. G. (2017). Second-order fourth-moment method for structural reliability. Journal of Engineering Mechanics, 143(4), 06016010. [Google Scholar]
131. Huang, X., Li, Y., Zhang, Y., Zhang, X. (2018). A new direct second-order reliability analysis method. Applied Mathematical Modelling, 55, 68–80. [Google Scholar]
132. Zhao, Y. G., Ono, T., Kato, M. (2002). Second-order third-moment reliability method. Journal of Structural Engineering, 128(8), 1087–1090. [Google Scholar]
133. Lü, Q., Low, B. K. (2011). Probabilistic analysis of underground rock excavations using response surface method and SORM. Computers and Geotechnics, 38(8), 1008–1021. [Google Scholar]
134. Low, B. K. (2014). FORM, SORM, and spatial modeling in geotechnical engineering. Structural Safety, 49, 56–64. [Google Scholar]
135. Zeng, P., Jimenez, R., Li, T. (2016). An efficient quasi-Newton approximation-based SORM to estimate the reliability of geotechnical problems. Computers and Geotechnics, 76, 33–42. [Google Scholar]
136. Zeng, P., Li, T., Jimenez, R., Feng, X., Chen, Y. (2018). Extension of quasi-Newton approximation-based SORM for series system reliability analysis of geotechnical problems. Engineering with Computers, 34, 215–224. [Google Scholar]
137. Lee, I., Noh, Y., Yoo, D. (2012). A novel second-order reliability method (SORM) using noncentral or generalized chi-squared distributions. Journal of Mechanical Design, 134(10), 100912. [Google Scholar]
138. Yoo, D., Lee, I., Cho, H. (2014). Probabilistic sensitivity analysis for novel second-order reliability method (SORM) using generalized chi-squared distribution. Structural and Multidisciplinary Optimization, 50, 787–797. [Google Scholar]
139. Park, J. W., Lee, I. (2018). A study on computational efficiency improvement of novel SORM using the convolution integration. Journal of Mechanical Design, 140(2), 024501. [Google Scholar]
140. Mansour, R., Olsson, M. (2014). A closed-form second-order reliability method using noncentral chi-squared distributions. Journal of Mechanical Design, 136(10), 101402. [Google Scholar]
141. Strömberg, N. (2017). Reliability-based design optimization using SORM and SQP. Structural and Multidisciplinary Optimization, 56(3), 631–645. [Google Scholar]
142. Tapankov, M., Strömberg, N. (2012). Sampling-and SORM-based RBDO of a knuckle component by using optimal regression models. 12th AIAA Aviation Technology, Integration, and Operations (ATIO) Conference and 14th AIAA/ISSMO Multidisciplinary Analysis and Optimization Conference, Indianapolis, Indiana. [Google Scholar]
143. Lin, W., Huang, W., Guo, J., Zhang, M. (2016). Reliability-based robust design for flange based on OSAM and SORM. Journal of Computational Methods in Sciences and Engineering, 16(4), 943–953. [Google Scholar]
144. Loc, N. H., van THUY, T., Trung, P. Q. (2019). Reliability-based analysis of machine structures using second-order reliability method. Journal of Advanced Mechanical Design, Systems, and Manufacturing, 13(3), JAMDSM0063. [Google Scholar]
145. Lee, O. S., Kim, D. H. (2007). Reliability of fatigue damaged structure using FORM, SORM and fatigue model. World Congress on Engineering, pp. 1322–1328. London, UK. [Google Scholar]
146. Lee, C. H., Kim, Y. (2017). Reliability-based flaw assessment of a mooring chain using FORM and SORM. 27th International Ocean and Polar Engineering Conference, San Francisco, California, USA. [Google Scholar]
147. Lee, O. S., Hur, M. J., Park, Y. C., Kim, D. H. (2007). Vibration fatigue reliability of lead and lead-free solder joint by FORM/SORM. Key Engineering Materials, 345, 1393–1396. [Google Scholar]
148. Ditlevsen, O. (2005). SORM correction of FORM results for the FBC load combination problem. 9th International Conference on Structural Safety and Reliability, Rome, Italy. [Google Scholar]
149. Lee, O. S., Kim, D. H. (2006). Reliability estimation of buried pipeline using FORM, SORM and monte carlo simulation. Key Engineering Materials, 326, 597–600. [Google Scholar]
150. Zhu, L. S., Lu, H., Zhang, Y. M. (2021). A system reliability estimation method by the fourth moment saddlepoint approximation and copula functions. Quality and Reliability Engineering International, 37(6), 2950–2969. [Google Scholar]
151. Du, X. (2008). Saddlepoint approximation for sequential optimization and reliability analysis. Journal of Mechanical Design, 130(1), 011011. [Google Scholar]
152. Zhao, Q., Duan, J., Wu, T., Hong, J. (2023). Time-dependent reliability analysis under random and interval uncertainties based on Kriging modeling and saddlepoint approximation. Computers & Industrial Engineering, 182, 109391. [Google Scholar]
153. Du, X. (2010). System reliability analysis with saddlepoint approximation. Structural and Multidisciplinary Optimization, 42, 193–208. [Google Scholar]
154. Wu, H., Du, X. (2020). System reliability analysis with second-order saddlepoint approximation. ASCE-ASME Journal of Risk and Uncertainty in Engineering Systems, Part B: Mechanical Engineering, 6(4), 041001. [Google Scholar]
155. Yu, S., Wang, Z. (2019). A general decoupling approach for time-and space-variant system reliability-based design optimization. Computer Methods in Applied Mechanics and Engineering, 357, 112608. [Google Scholar]
156. Huang, Z. L., Jiang, C., Zhou, Y. S., Zheng, J., Long, X. Y. (2017). Reliability-based design optimization for problems with interval distribution parameters. Structural and Multidisciplinary Optimization, 55,513–528. [Google Scholar]
157. Cho, T. M., Lee, B. C. (2011). Reliability-based design optimization using convex linearization and sequential optimization and reliability assessment method. Structural Safety, 33(1), 42–50. [Google Scholar]
158. Li, X., Yang, Q., Wang, Y., Han, X., Cao, Y. et al. (2021). Development of surrogate models in reliability-based design optimization: A review. Mathematical Biosciences and Engineering, 18(5), 6386–6409. [Google Scholar] [PubMed]
159. Dubourg, V., Sudret, B., Bourinet, J. M. (2011). Reliability-based design optimization using kriging surrogates and subset simulation. Structural and Multidisciplinary Optimization, 44, 673–690. [Google Scholar]
160. Meng, D., Yang, S., de Jesus, A. M., Fazeres-Ferradosa, T., Zhu, S. P. (2023). A novel hybrid adaptive Kriging and water cycle algorithm for reliability-based design and optimization strategy: Application in offshore wind turbine monopile. Computer Methods in Applied Mechanics and Engineering, 412, 116083. [Google Scholar]
161. Meng, Z., Li, G., Wang, B. P., Hao, P. (2015). A hybrid chaos control approach of the performance measure functions for reliability-based design optimization. Computers & Structures, 146, 32–43. [Google Scholar]
162. Zhu, S. P., Keshtegar, B., Trung, N. T., Yaseen, Z. M., Bui, D. T. (2021). Reliability-based structural design optimization: Hybridized conjugate mean value approach. Engineering with Computers, 37, 381–394. [Google Scholar]
163. Yang, S., Meng, D., Guo, Y., Nie, P., Jesus, A. M. D. (2023). A reliability-based design and optimization strategy using a novel MPP searching method for maritime engineering structures. International Journal of Structural Integrity, 14(5), 809–826. [Google Scholar]
164. Liu, X., Li, T., Zhou, Z., Hu, L. (2022). An efficient multi-objective reliability-based design optimization method for structure based on probability and interval hybrid model. Computer Methods in Applied Mechanics and Engineering, 392, 114682. [Google Scholar]
165. Meng, Z., Guo, L., Wang, X. (2022). A general fidelity transformation framework for reliability-based design optimization with arbitrary precision. Structural and Multidisciplinary Optimization, 65, 1–16. [Google Scholar]
166. Chiralaksanakul, A., Mahadevan, S. (2005). First-order approximation methods in reliability-based design optimization. Journal of Mechanical Design, 127(5), 851–857. [Google Scholar]
167. Clark, C. E., DuPont, B. (2018). Reliability-based design optimization in offshore renewable energy systems. Renewable and Sustainable Energy Reviews, 97, 390–400. [Google Scholar]
168. Noh, Y., Choi, K. K., Du, L. (2009). Reliability-based design optimization of problems with correlated input variables using a Gaussian Copula. Structural and Multidisciplinary Optimization, 38, 1–16. [Google Scholar]
169. Du, X. (2012). Reliability-based design optimization with dependent interval variables. International Journal for Numerical Methods in Engineering, 91(2), 218–228. [Google Scholar]
170. Shan, S., Wang, G. G. (2008). Reliable design space and complete single-loop reliability-based design optimization. Reliability Engineering & System Safety, 93(8), 1218–1230. [Google Scholar]
171. Yang, M., Zhang, D., Han, X. (2020). New efficient and robust method for structural reliability analysis and its application in reliability-based design optimization. Computer Methods in Applied Mechanics and Engineering, 366, 113018. [Google Scholar]
172. Kaveh, A., Zaerreza, A. (2022). A new framework for reliability-based design optimization using metaheuristic algorithms. Structures, 38, 1210–1225. [Google Scholar]
173. Meng, D., Yang, S., Lin, T., Wang, J., Yang, H. et al. (2022). RBMDO using gaussian mixture model-based second-order mean-value saddlepoint approximation. Computer Modeling in Engineering & Sciences, 132(2), 553–568. https://doi.org/10.32604/cmes.2022.020756 [Google Scholar] [CrossRef]
174. Guo, S. (2014). An efficient third-moment saddlepoint approximation for probabilistic uncertainty analysis and reliability evaluation of structures. Applied Mathematical Modelling, 38(1), 221–232. [Google Scholar]
175. Liu, T. W., Bai, J. B., Fantuzzi, N., Bu, G. Y., Li, D. (2022). Multi-objective optimisation designs for thin-walled deployable composite hinges using surrogate models and genetic algorithms. Composite Structures, 280, 114757. [Google Scholar]
176. Wang, Z., Xiao, F., Cao, Z. (2022). Uncertainty measurements for Pythagorean fuzzy set and their applications in multiple-criteria decision making. Soft Computing, 26(19), 9937–9952. [Google Scholar]
177. Wu, K., Xiao, F. (2023). A novel quantum belief entropy for uncertainty measure in complex evidence theory. Information Sciences, 652, 119744. [Google Scholar]
178. Meng, D., Huang, H. Z., Wang, Z., Xiao, N. C., Zhang, X. L. (2014). Mean-value first-order saddlepoint approximation based collaborative optimization for multidisciplinary problems under aleatory uncertainty. Journal of Mechanical Science and Technology, 28, 3925–3935. [Google Scholar]
179. Meng, D., Hu, Z., Wu, P., Zhu, S. P., Correia, J. A. et al. (2020). Reliability-based optimisation for offshore structures using saddlepoint approximation. Proceedings of the Institution of Civil Engineers-Maritime Engineering, 173(2), 33–42. [Google Scholar]
180. Lu, H., Cao, S., Zhu, Z., Zhang, Y. (2020). An improved high order moment-based saddlepoint approximation method for reliability analysis. Applied Mathematical Modelling, 82, 836–847. [Google Scholar]
181. Wang, H., Yuan, Y., Mang, H. A., Ai, Q., Huang, X. et al. (2022). Thermal stresses in rectangular concrete beams, resulting from constraints at microstructure, cross-section, and supports. European Journal of Mechanics-A/Solids, 93, 104495. [Google Scholar]
Cite This Article
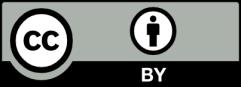
This work is licensed under a Creative Commons Attribution 4.0 International License , which permits unrestricted use, distribution, and reproduction in any medium, provided the original work is properly cited.