Open Access
ARTICLE
Gyroscope Dynamic Balance Counterweight Prediction Based on Multi-Head ResGAT Networks
School of Mechanical Engineering, Harbin Institute of Technology, Harbin, 150000, China
* Corresponding Author: Shisheng Zhong. Email:
Computer Modeling in Engineering & Sciences 2024, 139(3), 2525-2555. https://doi.org/10.32604/cmes.2023.046951
Received 20 October 2023; Accepted 17 November 2023; Issue published 11 March 2024
Abstract
The dynamic balance assessment during the assembly of the coordinator gyroscope significantly impacts the guidance accuracy of precision-guided equipment. In dynamic balance debugging, reliance on rudimentary counterweight empirical formulas persists, resulting in suboptimal debugging accuracy and an increased repetition rate. To mitigate this challenge, we present a multi-head residual graph attention network (ResGAT) model, designed to predict dynamic balance counterweights with high precision. In this research, we employ graph neural networks for interaction feature extraction from assembly graph data. An SDAE-GPC model is designed for the assembly condition classification to derive graph data inputs for the ResGAT regression model, which is capable of predicting gyroscope counterweights under small-sample conditions. The results of our experiments demonstrate the effectiveness of the proposed approach in predicting dynamic gyroscope counterweight in its assembly process. Our approach surpasses current methods in mitigating repetition rates and enhancing the assembly efficiency of gyroscopes.Keywords
Cite This Article
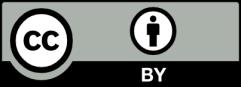