Open Access
ARTICLE
PAL-BERT: An Improved Question Answering Model
1 School of Automation, University of Electronic Science and Technology of China, Chengdu, 610054, China
2 Department of Geography and Anthropology, Louisiana State University, Baton Rouge, LA, 70803, USA
* Corresponding Author: Lirong Yin. Email:
Computer Modeling in Engineering & Sciences 2024, 139(3), 2729-2745. https://doi.org/10.32604/cmes.2023.046692
Received 11 October 2023; Accepted 14 December 2023; Issue published 11 March 2024
Abstract
In the field of natural language processing (NLP), there have been various pre-training language models in recent years, with question answering systems gaining significant attention. However, as algorithms, data, and computing power advance, the issue of increasingly larger models and a growing number of parameters has surfaced. Consequently, model training has become more costly and less efficient. To enhance the efficiency and accuracy of the training process while reducing the model volume, this paper proposes a first-order pruning model PAL-BERT based on the ALBERT model according to the characteristics of question-answering (QA) system and language model. Firstly, a first-order network pruning method based on the ALBERT model is designed, and the PAL-BERT model is formed. Then, the parameter optimization strategy of the PAL-BERT model is formulated, and the Mish function was used as an activation function instead of ReLU to improve the performance. Finally, after comparison experiments with traditional deep learning models TextCNN and BiLSTM, it is confirmed that PAL-BERT is a pruning model compression method that can significantly reduce training time and optimize training efficiency. Compared with traditional models, PAL-BERT significantly improves the NLP task’s performance.Keywords
Cite This Article
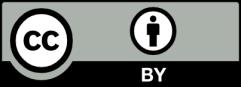
This work is licensed under a Creative Commons Attribution 4.0 International License , which permits unrestricted use, distribution, and reproduction in any medium, provided the original work is properly cited.