Open Access
ARTICLE
Japanese Sign Language Recognition by Combining Joint Skeleton-Based Handcrafted and Pixel-Based Deep Learning Features with Machine Learning Classification
1 School of Computer Science and Engineering, The University of Aizu, Aizuwakamatsu, 965-8580, Japan
2 Department of Computer Science & Engineering, Rajshahi University of Engineering & Technology, Rajshahi, 6204, Bangladesh
* Corresponding Author: Jungpil Shin. Email:
Computer Modeling in Engineering & Sciences 2024, 139(3), 2605-2625. https://doi.org/10.32604/cmes.2023.046334
Received 27 September 2023; Accepted 22 November 2023; Issue published 11 March 2024
Abstract
Sign language recognition is vital for enhancing communication accessibility among the Deaf and hard-of-hearing communities. In Japan, approximately 360,000 individuals with hearing and speech disabilities rely on Japanese Sign Language (JSL) for communication. However, existing JSL recognition systems have faced significant performance limitations due to inherent complexities. In response to these challenges, we present a novel JSL recognition system that employs a strategic fusion approach, combining joint skeleton-based handcrafted features and pixel-based deep learning features. Our system incorporates two distinct streams: the first stream extracts crucial handcrafted features, emphasizing the capture of hand and body movements within JSL gestures. Simultaneously, a deep learning-based transfer learning stream captures hierarchical representations of JSL gestures in the second stream. Then, we concatenated the critical information of the first stream and the hierarchy of the second stream features to produce the multiple levels of the fusion features, aiming to create a comprehensive representation of the JSL gestures. After reducing the dimensionality of the feature, a feature selection approach and a kernel-based support vector machine (SVM) were used for the classification. To assess the effectiveness of our approach, we conducted extensive experiments on our Lab JSL dataset and a publicly available Arabic sign language (ArSL) dataset. Our results unequivocally demonstrate that our fusion approach significantly enhances JSL recognition accuracy and robustness compared to individual feature sets or traditional recognition methods.Keywords
Cite This Article
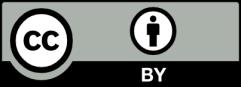
This work is licensed under a Creative Commons Attribution 4.0 International License , which permits unrestricted use, distribution, and reproduction in any medium, provided the original work is properly cited.