Open Access
ARTICLE
Improving Prediction of Chronic Kidney Disease Using KNN Imputed SMOTE Features and TrioNet Model
1 Department of Information Systems, College of Computer and Information Sciences, Princess Nourah bint Abdulrahman University, P.O. Box 84428, Riyadh, 11671, Saudi Arabia
2 Department College of Computer Science and Engineering, University of Hafr Al-Batin, Hafar, Al-Batin, 39524, Saudi Arabia
3 Department of Computer Science & Information Technology, The Islamia University of Bahawalpur, P.O. Box 63100, Bahawalpur, Pakistan
4 Department of Computer Science, College of Computer Engineering and Sciences, Prince Sattam bin Abdulaziz University, P.O. Box 151, Al-Kharj, 11942, Saudi Arabia
5 Information Technology and Management, Illinois Institute of Technology, Chicago, IL 60616-3793, USA
6 Information and Communication Engineering, Yeungnam University, Gyeongsan, 38541, Korea
* Corresponding Authors: Muhammad Umer. Email: ; Imran Ashraf. Email:
(This article belongs to the Special Issue: AI-Based Tools for Precision Medicine Solutions)
Computer Modeling in Engineering & Sciences 2024, 139(3), 3513-3534. https://doi.org/10.32604/cmes.2023.045868
Received 10 September 2023; Accepted 29 November 2023; Issue published 11 March 2024
Abstract
Chronic kidney disease (CKD) is a major health concern today, requiring early and accurate diagnosis. Machine learning has emerged as a powerful tool for disease detection, and medical professionals are increasingly using ML classifier algorithms to identify CKD early. This study explores the application of advanced machine learning techniques on a CKD dataset obtained from the University of California, UC Irvine Machine Learning repository. The research introduces TrioNet, an ensemble model combining extreme gradient boosting, random forest, and extra tree classifier, which excels in providing highly accurate predictions for CKD. Furthermore, K nearest neighbor (KNN) imputer is utilized to deal with missing values while synthetic minority oversampling (SMOTE) is used for class-imbalance problems. To ascertain the efficacy of the proposed model, a comprehensive comparative analysis is conducted with various machine learning models. The proposed TrioNet using KNN imputer and SMOTE outperformed other models with 98.97% accuracy for detecting CKD. This in-depth analysis demonstrates the model’s capabilities and underscores its potential as a valuable tool in the diagnosis of CKD.Keywords
Cite This Article
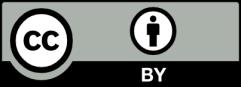