Open Access
REVIEW
Recent Advances on Deep Learning for Sign Language Recognition
School of Mathematics and Information Science, Nanjing Normal University of Special Education, Nanjing, 210038, China
* Corresponding Author: Xianwei Jiang. Email:
Computer Modeling in Engineering & Sciences 2024, 139(3), 2399-2450. https://doi.org/10.32604/cmes.2023.045731
Received 06 September 2023; Accepted 21 November 2023; Issue published 11 March 2024
Abstract
Sign language, a visual-gestural language used by the deaf and hard-of-hearing community, plays a crucial role in facilitating communication and promoting inclusivity. Sign language recognition (SLR), the process of automatically recognizing and interpreting sign language gestures, has gained significant attention in recent years due to its potential to bridge the communication gap between the hearing impaired and the hearing world. The emergence and continuous development of deep learning techniques have provided inspiration and momentum for advancing SLR. This paper presents a comprehensive and up-to-date analysis of the advancements, challenges, and opportunities in deep learning-based sign language recognition, focusing on the past five years of research. We explore various aspects of SLR, including sign data acquisition technologies, sign language datasets, evaluation methods, and different types of neural networks. Convolutional Neural Networks (CNN) and Recurrent Neural Networks (RNN) have shown promising results in fingerspelling and isolated sign recognition. However, the continuous nature of sign language poses challenges, leading to the exploration of advanced neural network models such as the Transformer model for continuous sign language recognition (CSLR). Despite significant advancements, several challenges remain in the field of SLR. These challenges include expanding sign language datasets, achieving user independence in recognition systems, exploring different input modalities, effectively fusing features, modeling co-articulation, and improving semantic and syntactic understanding. Additionally, developing lightweight network architectures for mobile applications is crucial for practical implementation. By addressing these challenges, we can further advance the field of deep learning for sign language recognition and improve communication for the hearing-impaired community.Keywords
Cite This Article
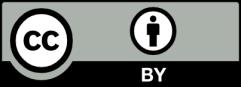
This work is licensed under a Creative Commons Attribution 4.0 International License , which permits unrestricted use, distribution, and reproduction in any medium, provided the original work is properly cited.