Open Access
ARTICLE
KSKV: Key-Strategy for Key-Value Data Collection with Local Differential Privacy
1 Artificial Intelligence Development Research Center, Institute of Scientific and Technical Information of China, Beijing, 100038, China
2 Industry Development Department, NSFOCUS Inc., Beijing, China
3 School of Information Science and Technology, Beijing Forestry University, Beijing, 100083, China
4 School of Computer Science and Engineering, University of Electronic Science and Technology of China, Chengdu, 610054, China
5 Institute of Computing Technology, China Academy of Railway Sciences Corporation Limited, Beijing, 10081, China
* Corresponding Authors: Chuanwen Luo. Email: ; Ting Chen. Email:
(This article belongs to the Special Issue: Privacy-Preserving Technologies for Large-scale Artificial Intelligence)
Computer Modeling in Engineering & Sciences 2024, 139(3), 3063-3083. https://doi.org/10.32604/cmes.2023.045400
Received 25 August 2023; Accepted 08 November 2023; Issue published 11 March 2024
Abstract
In recent years, the research field of data collection under local differential privacy (LDP) has expanded its focus from elementary data types to include more complex structural data, such as set-value and graph data. However, our comprehensive review of existing literature reveals that there needs to be more studies that engage with key-value data collection. Such studies would simultaneously collect the frequencies of keys and the mean of values associated with each key. Additionally, the allocation of the privacy budget between the frequencies of keys and the means of values for each key does not yield an optimal utility tradeoff. Recognizing the importance of obtaining accurate key frequencies and mean estimations for key-value data collection, this paper presents a novel framework: the Key-Strategy Framework for Key-Value Data Collection under LDP. Initially, the Key-Strategy Unary Encoding (KS-UE) strategy is proposed within non-interactive frameworks for the purpose of privacy budget allocation to achieve precise key frequencies; subsequently, the Key-Strategy Generalized Randomized Response (KS-GRR) strategy is introduced for interactive frameworks to enhance the efficiency of collecting frequent keys through group-and-iteration methods. Both strategies are adapted for scenarios in which users possess either a single or multiple key-value pairs. Theoretically, we demonstrate that the variance of KS-UE is lower than that of existing methods. These claims are substantiated through extensive experimental evaluation on real-world datasets, confirming the effectiveness and efficiency of the KS-UE and KS-GRR strategies.Keywords
Cite This Article
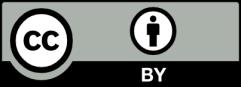