Open Access
ARTICLE
Quick Weighing of Passing Vehicles Using the Transfer-Learning-Enhanced Convolutional Neural Network
1 College of Civil Engineering, Xiangtan University, Xiangtan, 411105, China
2 Energy & Environmental Engineering Department, China CEC Engineering Corporation, Changsha, 410114, China
* Corresponding Author: Wangchen Yan. Email:
Computer Modeling in Engineering & Sciences 2024, 139(3), 2507-2524. https://doi.org/10.32604/cmes.2023.044709
Received 06 August 2023; Accepted 28 September 2023; Issue published 11 March 2024
Abstract
Transfer learning could reduce the time and resources required by the training of new models and be therefore important for generalized applications of the trained machine learning algorithms. In this study, a transfer learning-enhanced convolutional neural network (CNN) was proposed to identify the gross weight and the axle weight of moving vehicles on the bridge. The proposed transfer learning-enhanced CNN model was expected to weigh different bridges based on a small amount of training datasets and provide high identification accuracy. First of all, a CNN algorithm for bridge weigh-in-motion (B-WIM) technology was proposed to identify the axle weight and the gross weight of the typical two-axle, three-axle, and five-axle vehicles as they crossed the bridge with different loading routes and speeds. Then, the pre-trained CNN model was transferred by fine-tuning to weigh the moving vehicle on another bridge. Finally, the identification accuracy and the amount of training data required were compared between the two CNN models. Results showed that the pre-trained CNN model using transfer learning for B-WIM technology could be successfully used for the identification of the axle weight and the gross weight for moving vehicles on another bridge while reducing the training data by 63%. Moreover, the recognition accuracy of the pre-trained CNN model using transfer learning was comparable to that of the original model, showing its promising potentials in the actual applications.Keywords
Cite This Article
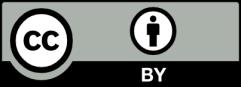
This work is licensed under a Creative Commons Attribution 4.0 International License , which permits unrestricted use, distribution, and reproduction in any medium, provided the original work is properly cited.