Open Access
ARTICLE
A Study on the Transmission Dynamics of the Omicron Variant of COVID-19 Using Nonlinear Mathematical Models
1 Department of Mathematics, Periyar University, Salem, Tamilnadu, 636011, India
2 Faculty of Engineering and Natural Sciences, Istanbul Okan University, Istanbul, Turkey
3 Department of Mathematics, College of Science and Humanities in Alkharj, Prince Sattam Bin Abdulaziz University, Alkharj, 11942, Saudi Arabia
4 Department of Applied Mathematics, Faculty of Mathematics, Statistics and Computer Science, University of Tabriz, Tabriz, Iran
* Corresponding Author: Pushpendra Kumar. Email:
(This article belongs to the Special Issue: Recent Developments on Computational Biology-I)
Computer Modeling in Engineering & Sciences 2024, 139(3), 2265-2287. https://doi.org/10.32604/cmes.2023.030286
Received 29 March 2023; Accepted 26 July 2023; Issue published 11 March 2024
Abstract
This research examines the transmission dynamics of the Omicron variant of COVID-19 using SEIQIcRVW and SQIRV models, considering the delay in converting susceptible individuals into infected ones. The significant delays eventually resulted in the pandemic’s containment. To ensure the safety of the host population, this concept integrates quarantine and the COVID-19 vaccine. We investigate the stability of the proposed models. The fundamental reproduction number influences stability conditions. According to our findings, asymptomatic cases considerably impact the prevalence of Omicron infection in the community. The real data of the Omicron variant from Chennai, Tamil Nadu, India, is used to validate the outputs.Keywords
Since COVID-19 is a newly discovered virus, little is known about how it spreads. As a result, health authorities must thoroughly understand the incubation and recovery periods to implement more efficient quarantine procedures for those suspected of carrying the virus. As of November 24, 2021, Omicron has been found in countries, and it continues to be the most popular variant all over the world. The transmission dynamics and the potential roles of various intervention strategies have been better understood by recent COVID-19 studies [1–7]. These methodologies incorporate relief and concealment to dial back the spread of the pandemic, decreasing pinnacle medical care to safeguard the people who are most in danger from contaminations, lessening the number of infective cases to a low level, implementing lockdown to a district of exceptionally infective cases, confining suspect cases at home, isolating those residing in a similar family at home. Some authors developed an Omicron variant model with variable population size [8–14].
After becoming infected, a strengthening of the immune system may cause a delay in entering the infectious stage, and a significant amount of delay may even result in the disease being stopped at the exposure level. As a result, the effect of time delay on studying the dynamics of disease spread is significant. In addition, the effect of quarantine on preventing disease spread and the transmission of infection from both the exposed and infected groups are taken into consideration. On the one hand, people who were exposed have the virus, but unlike an asymptomatic patient, they do not show any symptoms right away. There is a latency period before an exposed person becomes infected, and it can take up to 14 days for some people to become infected. By developing the integer model, the current study aims to investigate the effects of the latency period. Using the delay differential equations model, newly infected individuals are given some time before contracting the disease.
To prevent COVID-19 infection in the host population, some authors developed delay-type models. Liu et al. proposed a time delay model and utilised the methodology to analyse the COVID-19 pandemic in China [15]. A new form of disease model based on a time delay dynamics was developed in [16]. They fitted model parameters based on the total number of reported cases in Beijing and Wuhan, China. Using mathematical and statistical modelling, Sedighe et al. developed a model to determine the epidemic trend and forecast the number of patients hospitalised due to COVID-19 in Iran [17]. The SEIQR COVID-19 propagation model with two delays was investigated by Fangfung et al. in [18]. Their model took supply chain transmission and hierarchical quarantine rate into account. A modified SIR model which combines suitable delay parameters and generates a more reliable forecasts of COVID-19 real-time data was proposed in [19]. Where the authors compared the predictions of the recently constructed SIR model to actual data collected from Germany, Italy, Kuwait, and Oman. Shidong et al. created a delay SEIR model based on the feedback linearization technique to manage the effects of COVID-19 [20]. The authors in [21] proposed a SIRDV model to investigate the impact of vaccination campaigns during the pandemic in Israel and Great Britain. In [22], the authors introduced a time delay model considering the migration of individuals from susceptible to infected class. The Omicron model can be mathematically modeled in a way that is reasonably accurate to the occurrences that have been observed when delay factors are included in the system of differential equations.
In this paper, two delay mathematical models are proposed. The work is significant, because it contains the mathematical modeling with a real-data of the Omicron variant from Chennai, Tamil Nadu, India. In the form of sections, the delayed SQIRV model is proposed and its stability is examined in Section 2. The delayed SEIQIc RVW model is proposed and steady-state solution existence is tested in Section 3. In order to confirm and strengthen our theoretical findings regarding Omicron B.1.1.529 SARS-Cov-2, computational simulations are carried out from the real data which is collected from Tamilnadu in Section 4. In Section 5, we summarise our findings.
2 Delayed SQIRV Mathematical Model
Here we define the delay-type version of the integer-order SQIRV model proposed in [23]. The disease is considered to have an incubation time of τ>0, because the virus moved from the susceptible phase to the incubation phase. The time between becoming susceptible to the virus and experiencing its symptoms is referred to as the incubation period. Based on the policy decisions made by the government, a set of parameters have been obtained to forecast the pandemic trend. Table 1 lists the non-negative parameters that are used in this model.
Considering the given aspects, the delay SQIRV mathematical model is derived as follows:
dSdt=Γ−η21S−η3S(t−τ)I(t−τ)+η4Q+η5R+η6V,dQdt=η7I−η22Q−η9QV,dIdt=η3S(t−τ)I(t−τ)−(η23)I(t),dRdt=η12I(t)+η8Q−η24R,dVdt=η11R+η2S−η25V+η9QV,(1)
where η21=η1+η2, η22=η1+η4+η8, η23=η1+η7+η10+η12, η24=η1+η5+η11, and η25=η1+η6
Subject to initial conditions S(0)=S0,Q(0)=Q0,I(0)=I0,R(0)=R00,V(0)=V0.
As in the case of Omicron, a susceptible individual is assumed to interact with an infected individual in the equation system but does not enter the infected compartment until after a predetermined incubation period. The incubation period τ is just while moving from the powerless compartment to the contaminated compartment.
There are two steady-state solutions to the model under consideration. Time-independent solutions are obtained when the model system (1) is made static. The steady-state solution, I=0, when there are no infections is given by
E0=(S,Q,I,R,V)=(Γ(η1+η6)η1(η1+η2+η6),0,0,0,Γη2η1(η1+η2+η6))(2)
Also, the steady-state solution when infection is persistent i.e., I≠0 is given by
E∗ =(S∗,Q∗,I∗,R∗,V∗) =(ν33η3,η7I∗η22+η9V∗,η3Γ−η21ν33+η6Cν33−η4A−η5B−η6C∗,η8Q∗+η12I∗η1+η5+η11,η2η11R∗+η2η23η2(η1+η6−η9Q∗))(3)
where A=η7η22+η9V∗, B=η12η1+η5+η11+η7η8(η22+η9V∗)(η1+η5+η11), C=η11Bη1+η6−η9Q∗.
The fundamental reproduction number R0 is calculated by using the next generation operator matrix as folows [24,25]:
R0 is the largest eigenvalue of the spectral radius given by
R0(FV−1)=η3(Γ(η1+η6)η1(η1+η2+η6))(1(η1+η10+η12+η7))(4)
2.1 Stability Analysis of the Delayed SQIRV Model
The following theorem applies Rouche’s theorem [26] to characterise the local stability of the SQIRV system (1) for the infection-free steady state solution (2). The output is determined by the reproduction number R0.
Theorem 2.1. The infection free steady state E0 is locally asymptotically stable if R0<1 and unstable if R0>1 for τ≥0.
Proof. The characteristic equation of system (1), for the equilibrium point E0, is given by
Δ(λ)=|λId5×5−J100−J101e−τλ|(5)
where J100
=(−(η1+η2)η40η5η60−(η1+η4+η8+η9V)η70000−(η1+η7+η10+η12)000η8η12−(η1+η5+η11)0η2η9V0η11−(η1+η6))
and
J101=(00−η3Se−tτ000000000η3Se−tτ000000000000),
C(λ) =(λ+(η1+η4+η7+η8))(λ+(η1+η4+η11))(λ−η3Se−λτ+(η1+η7+η10+η12)) (λ+12((η1+η2)+(η1+η6)±√(η1+η2)2+4η2η6−η1η2(η1+η6)+(η1+η6)2)).(6)
Then from the Jacobian matrix, the eigen values are −(η1+η4+η7+η8),−(η1+η4+η11),η3Se−λτ−(η1+η7+η10+η12), and 12(−(η1+η2)−(η1+η6)±√(η1+η2)2+4η2η6−η1η2(η1+η6)+(η1+η6)2).
When τ=0, the System (1) is stable iff η3S−(η1+η7+η10+η12)<0, and η1(η1+η2+η6)>1.
Then clearly the infection free steady state E0 (2) is locally asymptotically stable if R0<1.
Let τ>0. In this case, we will use Rouches s theorem to prove that all roots of the characteristic Eq. (5) cannot intersect the imaginary axis, i.e., the characteristic equation cannot have pure imaginary roots.
Suppose for the opposite, that is, suppose there exists w∈R such that λ=wi is a solution of (19).
Consider the term η3Se−iwτ−(η1+η7+η10+η12)=0
⟹ wi+(η1+η7+η10+η12)=η3Se−iwτ
⟹ wi+(η1+η7+η10+η12)=η3S(coswτ−isinwτ)
Equating the real and imaginary parts we get
w=−iη3Ssinwτ,(η1+η7+η10+η12)=η3Scoswτ
Squaring and adding we get ⟹ (w)2+(η1+η7+η10+η12)2=μsS2
⟹ w2=μsS2−(η1+η7+η10+η12)2
If R0<1, then w2<0, which is a contradiction.
Thus the infection free consistent state E0 is locally asymptotically stable if R0<1 for τ≥0.
The Ruth-Hurwitz stability theory and Rouche’s theorem are used in the following theorem to characterize the local stability of the SQIRV system (1) for the infectious persistent steady state solution (3). The consequence is determined by the reproduction number R0.
Theorem 2.2. The infection persistent steady state solution E∗ of (1) is locally asymptotically stable if R0>1 for τ≥0.
Proof.
The characteristic equation of system (1), for the equilibrium point E∗, is given by
Δ(λ)=|λId5×5−J110−J111e−τλ|(7)
where J110=
(−(η1+η2)η40η5η60−(η1+η4+η8+η9V∗)η70−η9Q∗00−(η1+η7+η10+η12)000η8η12−(η1+η5+η11)0η2η9V∗0η11−(η1+η6)+η9Q∗)
and
J111=(−η3I∗e−tτ0−η3S∗e−tτ0000000η∗3e−tτ0η3S∗e−tτ000000000000)
The characteristic polynomial is
λ5 +(e−λτη3I∗+F0)λ4+(e−λτη3I∗F1+F2)λ3+(e−λτη3I∗F3+F4)λ2 +(e−λτη3I∗F5+F6)λ+(e−λτη3I∗F7+F8)=0(8)
where F0=η21−η9Q∗+η22+η9V∗+η24+η25, F1=η22+η9V∗+η24+η25−η9Q∗, F2=η21(η22+η24+η25+η9V∗−η9Q∗)−η2η6,
F3=(η24+η25+η3S∗−η9Q∗)(η22+η9V∗)−η4η7−η3S∗η9Q∗−η5η12−η9Q∗η24+η24η25+η9Q∗η9V∗+η3S∗η24+η3S∗η25,
F4=(η21−η9Q∗)(η22+η9V∗)η24−[η9Q∗((η21)(η22+η9V∗)+η21η24)+η2η6((η22+η9V∗)+η24)]+η21((η22+η9V∗)η25+η24η25+η9Q∗η9V∗)+η9Q∗(η2η4+η8η11)+η24((η22+η9V∗)η25+η9Q∗η9V∗),
F5=η5η9Q∗η12−η3S∗η9Q∗(η22+η9V∗+η24)+η4η7(η24+η25)+η5η12(η22+η9V∗+η25)+η5(η7η8+η6η9V∗)+η6η12η11,
F5=(η9Q∗(η8η11+η24η9V∗)+(η22+η9V∗)η24(η25−η9Q∗))−(η3S∗η9Q∗(η22+η9V∗+η24)+η4η7(η24+η25)+η5η12(η22+η9V∗+η25)+η5(η7η8+η6η9V∗)+η6η12η11),
F6=(η9Q∗(η8η11+η24η9V∗)+(η22+η9V∗)η24(η25−η9Q∗))+η4η9Q∗(η5η7+η2η24)+η5η9Q∗(η3I∗η12+η2η8),
F7=η5η9Q∗(η7η8+η12(η22+η9V∗))+η4η9Q∗η12η11,
F8=(η8η9V∗+η24η9V∗)(η3S∗η9Q∗−η6η7)+η24(η9Q∗+η25)(η4η7−η3S∗(η22+η9V∗))−[η12(η22+η9V∗)(η6η24+η5η25) + η5(η7η8η25+η9Q∗η12η11)]
If τ=0, then by using the rule of Descartes of sign, we can get there are no positive real roots.
Also by Routh-Hurwitz stability criterion, the real parts of the complex roots are also negative if η3I∗(Fi)+Fj>0 for i=1,3,5,7;j=0,2,4,6,8, (R0−1)>0,R0>1. Then the infection persistent steady state (S∗,Q∗,I∗,R∗,V∗) is locally stable when R0>1.
If τ>0, then by using Rouch’s theorem, we have to prove that all roots of the characteristic Eq. (6) cannot have pure imaginary roots.
Suppose that there exists w∈R such that λ=wi is a solution of (6).
Now Eq. (22) becomes
iw5 +(e−iwτη3I∗+F0)w4−i(e−iwτη3I∗F1+F2)w3−(e−iwτη3I∗F3+F4)w2+i(e−iwτη3I∗F5+F6)w −(e−iwτη3I∗F7+F8)=0(9)
Then
iw5 +F0w4−iF2w3−F4w2+iF6w+F8 =η3I∗(−w4+iF1w3+F3w2−iF5w−F7)(cosτw−isinτw)(10)
Equating the real and imaginary parts of (10) we get
F0w4−F4w2+F8=η3I∗(−w4+F3w2−F7)cosτw+(F1w3−F5w)sinτw(11)
w5−F2w3+F6w=(F1w3−F5w)cosτw−η3I∗(−w4+F3w2−F7)sinτw(12)
Squaring both Eqs. (12), (12) and adding we get
w10 +(F20−2F2−η3I∗2)w8+[F22+2F6−2F0F4−η3I∗2(F21−2F3)]w6+[F24+2F0F8−2F0F6 −η3I∗2(F23−2F1F5)]w4+[F26−2F7F8−η3I∗2(F25−2F3F7)]w2+(F28−η3I∗2F27)=0.(13)
Let z=w2 in (13)
F(z) =z5+(F20−2F2−η3I∗2)z4+[F22+2F6−2F0F4−η3I∗2(F21−2F3)]z3+[F24+2F0F8−2F0F6 −η3I∗2(F23−2F1F5)]z2+[F26−2F7F8−η3I∗2(F25−2F3F7)]z+(F28−η3I∗2F27)=0.(14)
If R0>1, then from Eq. (14) we can see that F28−η3I∗2F27 is strictly negtive which implies F(0)>0. Thus we can get atleast one positive real root. Hence, if R0>1 all the real parts of the roots of (8) are negative. Thus the equilibrium position E∗ is stable when R0>1 for τ≥0.
3 Delayed SEIQIcRVW Model Formulation
This section is focused on constructing delay SEIQIcRVW model for our problem formulation. The delayed SEIQIcRVW model can be formulated from the integer-order model form given in [27]. It is considered that the disease has an incubation time of the virus τ>0 transferred from susceptible period to an incubation period. The incubation period is the delay time that passes between being susceptible and showing symptoms of the virus. The suitable parameters are used to formulate the Omicron delayed SEIQIcRVW Model, which are described in Table 2.
Considering the given aspects, the SEIQIcRVW delay mathematical model is derived as follows:
dSdt=P−δnS−μsS(t−τ)I(t−τ)+ρrR+ρvV,dEdt=μsS(t−τ)I(t−τ)−(ξ1)E,dIdt=γiE−(ξ2)I,dQdt=kE+ζI+ζqIc−(ξ3)Q,dIcdt=δcE−(ξ4)Ic,dRdt=νrI+γrQ+γcIc−(ξ5)R,dVdt=ηvQ+αvR−(ξ6)VdWdt=ωcI+ζwIc−δnW,(15)
where ξ1=δn+k+γi+δc, ξ2=δn+δe+νr+ζ+ωc, ξ3=γr+ηv+δn, ξ4=ζq+γc+ζw+δn, ξ5=δn+ρr+αv and ξ6=δn+ρv.
Subject to initial conditions: S(0)=S0,E(0)=E0,I(0)=I0,Q(0)=Q0,Ic(0)=Ic0,R(0)=R00,V(0)=V0.
3.1 Steady State Solutions the Delayed SEIQIcRVW Model
The system (15) is found static, i.e., the solutions of time independent are obtained. The steady state solutions in the infection free state, when I=0 is given by
E0q =(S0,E0,I0,Q0,I0c,R0,V0,W0)=(Pδn,0,0,0,0,0,0,0).(16)
Also, when infection is persistent the steady state solutions, i.e., I≠0 is given by
E∗q=(S∗,E∗,I∗,Q∗,I∗c,R∗,V∗,W∗)(17)
where
S∗ =ξ2γi(Pμsγi−δnξ1ξ2μs(ξ1ξ2−ρvγiJ−γiG)),E∗=ξ1ξ2μsγi(Pμsγi−δnξ1ξ2μs(ξ1ξ2−ρvγiJ−γiG))I∗=Pμsγi−δnξ1ξ2μs(ξ1ξ2−ρvγiJ−γiG)=δnξ1ξ2(R0−1)μs(ξ1ξ2−ρvγiJ−γiG)Q∗=ξ2ξ4+ζξ4γi+ζqδcξ2ξ3ξ4γi(Pμsγi−δnξ1ξ2μs(ξ1ξ2−ρvγiJ−γiG))I∗c=δcξ2ξ4γi(Pμsγi−δnξ1ξ2μs(ξ1ξ2−ρvγiJ−γiG))R∗=νrγiξ3ξ4+γr(ξ2ξ4+ζξ4γi+ζqδcξ2)+γiδcξ2ξ3γiξ3ξ4ξ5(Pμsγi−δnξ1ξ2μs(ξ1ξ2−ρvγiJ−γiG))V∗=ηvγiξ5(ξ2ξ4+ζξ4γi+ζqδcξ2)ξ3ξ4γiξ5 +αv[νrγiξ3ξ4+γr(ξ2ξ4+ζξ4γi+ζqδcξ2)+γiδcξ2ξ3]ξ3ξ4γiξ5(Pμsγi−δnξ1ξ2μs(ξ1ξ2−ρvγiJ−γiG))W∗=γiωcξ4+ζwδcξ2δnγiξ4(Pμsγi−δnξ1ξ2μs(ξ1ξ2−ρvγiJ−γiG))
with
J =νrγiξ3ξ4+γr(ξ2ξ4+ζξ4γi+ζqδcξ2)+γiδcξ2ξ3γiξ3ξ4ξ5,G =ηvγiξ5(ξ2ξ4+ζξ4γi+ζqδcξ2)ξ3ξ4γiξ5 +αv[νrγiξ3ξ4+γr(ξ2ξ4+ζξ4γi+ζqδcξ2)+γiδcξ2ξ3]ξ3ξ4γiξ5.
The basic reproduction number R0 is
R0(GV−1)=Pμsγiδn(δn+k+γi+δc)(δn+δe+νr+ζ+ωc).(18)
3.2 Stability Analysis of the Delayed SEIQI cRVW Model
The local stability of the SEIQI cRVW system (15) for the infection-free steady state solution (16) is examined in the next theorem applying Rouche’s theorem. The reproduction number R0 determines the result.
Theorem 3.1. The infection free consistent state E0 (16) is locally asymptotically stable if R0<1 and unstable if R0>1 for the time delay τ≥0.
Proof. The characteristic equation of system 15, for the equilibrium point E0, is given by
Δ(λ)=|λId8×8−J00−J01e−τλ|(19)
where
J00=(−δn0000ρrρv00−ξ10000000γiξ2000000kζ−ξ3ζq0000δc00−ξ400000νrγrγc−ξ500000ηv0αv−ξ6000ωc0ζw00−δn),
and
J01=(00−μsS0000000μsS00000000000000000000000000000000000000000000000000000).
C1(λ) =(λ+δn)(λ+δn)(λ+ξ1+ξ2+√ξ21+4μsSγi−2ξ1ξ2+ξ22)(λ+ξ1+ξ2−√ξ21+4μsSγi−2ξ1ξ2+ξ22)(λ+ξ3)(λ+ξ4)(λ+ξ5)(λ+ξ6).(20)
When τ = 0, the eigenvalues are −δn, −δn, 12(−ξ1−ξ2−√ξ21+4μsSγi−2ξ1ξ2+ξ22), 12(−ξ1−ξ2+√ξ21+4μsSγi−2ξ1ξ2+ξ22), −ξ3, −ξ4, −ξ5, −ξ6.
The given system (15) is stable when −ξ1−ξ2+√ξ21+4μsSγi−2ξ1ξ2+ξ22<0
or √ξ21+4μsSγi−2ξ1ξ2+ξ22<(ξ1+ξ2) or ξ21+4μsSγi−2ξ1ξ2+ξ22<(ξ1+ξ2)2
or μsSγi<ξ1ξ2. i.e., μsSγiξ1ξ2<1.
That is R0<1. Clearly infection free steady state E0 is locally asymptotically stable if R0<1 when τ=0.
Let τ>0. In this case, we will use Rouche’s theorem to prove that all roots of the characteristic Eq. (19) cannot intersect the imaginary axis, i.e., the characteristic equation cannot have pure imaginary roots.
Suppose for the opposite, that is, suppose there exists w∈R such that λ=wi is a solution of (19).
Consider the term wi+ξ1+ξ2−√ξ21+4μsSe−τwiγi−2ξ1ξ2+ξ22=0
⟹ wi+ξ1+ξ2=√ξ21+4μsSe−τwiγi−2ξ1ξ2+ξ22=0
⟹ (wi+ξ1+ξ2)2=ξ21+4μsSe−τwiγi−2ξ1ξ2+ξ22=0
⟹ (wi)2+(ξ1+ξ2)2+wi(ξ1+ξ2)−ξ21+2ξ1ξ2−ξ22=4μsS(cosτw−isinτw)γi
⟹ −w2+wi(ξ1+ξ2)+4ξ1ξ2=4μsS(cosτw−isinτw)γi
By equating the real and imaginary part, we get
4ξ1ξ2−w2=4μsγiScosτw, w(ξ1+ξ2)=−4μsγiSsinτw
If R0<1, then μsSγi−ξ1ξ2>0. Hence w2<0, which is a contradiction.
Thus the infection free consistent state E0 is locally asymptotically stable if R0<1 for τ≥0.
Now suppose that R0>1 from the characteristic polynomial (20), it is enough to consider the term (λ+ξ1+ξ2−√ξ21+4μsSγi−2ξ1ξ2+ξ22). It is easy to see that C1(0)<0. On the other hand, limλ→+∞C1(λ)=+∞. Therefore, by continuity of C1(λ), there is at least one positive root of the characteristic Eq. (20). Hence, we conclude that Σ1 is unstable, for any τ≥0.
The local stability of the SEIQI cRVW system (15) for the infection’s persistent steady state solution (17) is determined using Rouche’s theorem and the Routh-Hurwitz technique in the next theorem. The result is governed by the reproduction number R0.
Theorem 3.2. If R0>1, then the endemic equilibrium point E∗ is locally asymptotically stable for τ≥0.
Proof. The characteristic equation of system 15, for the equilibrium point E∗ 17 is given by
Δ(λ)=|λId8×8−J10−J11e−τλ|.(21)
where the Jacobian matrices of the model at infection persistent steady state solution are
J(10)=(−δn0000ρrρv00−ξ10000000γiξ2000000kζ−ξ3ζq0000δc00−ξ400000νrγrγc−ξ500000ηv0αv−ξ6000ωc0ζw00−δn).
and
J(11)=(−μsI∗0−μsS∗00000μsI∗0μsS∗00000000000000000000000000000000000000000000000000000).
The characteristic equation is
(−δn−λ)(ρvμsI∗e−λτ[(ξ2ξ4kξ5ηv+ξ2ζqδcξ5ηv+ξ4ηγiξ5ηv+ξ3ξ4γiνrs+ξ2ξ4kγrαv+ξ2ζqδcγrαv+ξ4ηγiγrαv+ξ3ξ2δcγcαv)+(ξ2ξ4kηv+ξ2ζqδcηv+ξ4ηγiηv+ξ2kξ5ηv+ξ4kξ5ηv+ζqδcξ5ηv+ηγiξ5ηv+ξ3γiνrαv+ξ4γiνrαv+ξ2kγrαv+ξ4kγrαv+ζqδcγrαv+ηγiγrαv+ξ3δcγcαv+ξ2δcγcαv)λ+(ξ2kηv+ξ4kηv+ζqδcηv+ηγiηv+kξ5ηv+γiνrαv+kγrαv+δcγcαv)λ2+kηvλ3]+(−ξ6−λ)(−ρrμsI∗e−λτ[(ξ3ξ4γiνr+ξ2ξ4kγr+ξ2ζqδcγr+ξ4ηγiγr+ξ3ξ2δcγc)+(ξ3γiνr+ξ4γiνr+ξ2kγr+ξ4kγr+ζqδcγr+ηγiγr+ξ3δcγc+ξ2δcγc)λ+(γiνr+kγr+δcγc)λ2]+(−ξ3−λ)(−ξ4−λ)(−ξ5−λ)(−γiμsS∗e−λτ(−δn−λ)+(−ξ2−λ)(δnξ1+ξ1μsI∗e−λτ+(δn+ξ1+μsI∗e−λτ)x+λ2))))=0.
To check about the stability, consider the second term of the above characteristic equation
λ7 +(e−λτμsI∗+D0)λ6+(e−λτ[μsI∗D1−μsS∗D2]+D3)λ5+(e−λτ[μsI∗D4−μsS∗D5]+D6)λ4 +(e−λτ[μsI∗D7−μsS∗D8]+D9)λ3+(e−λτ[μsI∗D10−μsS∗D11]+D12)λ2 +(e−λτ[μsI∗D13−μsS∗D14]+D15)λ+(e−λτ[μsI∗D16−μsS∗D17]+D18)=0(22)
where D0=δn+ξ1+ξ2+ξ3+ξ4+ξ5+ξ6, D1=ξ1+ξ2+ξ3+ξ4+ξ5+ξ6, D2=γi, D3=δnξ3+δnξ1+ξ3ξ1+δnξ2+ξ3ξ2+ξ1ξ2+δnξ4+ξ3ξ4+ξ1ξ4+ξ2ξ4+δnξ5+ξ3ξ5+ξ1ξ5+ξ2ξ5+ξ4ξ5+δnξ6+ξ3ξ6+ξ1ξ6+ξ2ξ6+ξ4ξ6+ξ5ξ6,
D4=ξ1ξ2+ξ1ξ3+ξ1ξ4+ξ1ξ5+ξ1ξ6+ξ2ξ3+ξ2ξ4+ξ2ξ5+ξ2ξ6+ξ3ξ4+ξ3ξ5+ξ3ξ6+ξ4ξ5+ξ4ξ6+ξ5ξ6, D5=γi[δn+ξ3+ξ4+ξ5+ξ6], D6=δn[ξ3ξ1+ξ3ξ2+ξ1ξ2+ξ3ξ4+ξ1ξ4+ξ2ξ4+ξ3ξ5+ξ1ξ5+ξ2ξ5+ξ4ξ5+ξ2ξ6+ξ3ξ6+ξ1ξ6+ξ4ξ6+ξ5ξ6]+ξ3ξ4ξ5+ξ1ξ4ξ5+ξ2ξ4ξ5+ξ3ξ1ξ2+ξ3ξ1ξ6+ξ3ξ2ξ6+ξ3ξ2ξ4+ξ3ξ2ξ5+ξ1ξ2ξ5+ξ1ξ2ξ4+ξ3ξ1ξ4+ξ1ξ2ξ6+ξ3ξ1ξ5+ξ3ξ4ξ6+ξ1ξ4ξ6+ξ2ξ4ξ6+ξ3ξ5ξ6+ξ1ξ5ξ6+ξ2ξ5ξ6+ξ4ξ5ξ6,
D7=ξ3ξ1ξ2+ξ3ξ1ξ2+ξ3ξ2ξ4+ξ1ξ2ξ4+ξ3ξ1ξ5+ξ3ξ2ξ5+ξ1ξ2ξ5+ξ3ξ4ξ5+ξ1ξ4ξ5+ξ2ξ4ξ5+ξ3ξ1ξ6+ξ3ξ2ξ6+ξ1ξ2ξ6+ξ3ξ4ξ6+ξ1ξ4ξ6+ξ2ξ4ξ6+ξ3ξ5ξ6+ξ1ξ5ξ6+ξ2ξ5ξ6+ξ4ξ5ξ6−ρrγiνr−ρrkγr−ρrδcγc−ρvkηv, D8=γi[ξ3ξ4+δnξ5+ξ3ξ5+δnξ6+ξ3ξ6+ξ4ξ6+ξ5ξ6+δnξ3+ξ4ξ5+δnξ4], D9=ξ3ξ1ξ2ξ4+δnξ3ξ1ξ2+δnξ3ξ1ξ4+δnξ3ξ2ξ4+δnξ1ξ2ξ4+δnξ3ξ1ξ5+δnξ3ξ2ξ5+δnξ1ξ2ξ5+ξ3ξ1ξ2ξ5+δnξ3ξ4ξ5+δnξ1ξ4ξ5+ξ3ξ1ξ4ξ5+δnξ2ξ4ξ5+ξ3ξ2ξ4ξ5+ξ1ξ2ξ4ξ5+δnξ3ξ1ξ6+δnξ3ξ2ξ6+δnξ1ξ2ξ6+ξ3ξ1ξ2ξ6+δnξ3ξ4ξ6+δnξ1ξ4ξ6+ξ3ξ1ξ4ξ6+δnξ2ξ4ξ6+ξ3ξ2ξ4ξ6+ξ1ξ2ξ4ξ6+δnξ3ξ5ξ6+δnξ1ξ5ξ6+ξ3ξ1ξ5ξ6+δnξ2ξ5ξ6+ξ3ξ2ξ5ξ6+ξ1ξ2ξ5ξ6+δnξ4ξ5ξ6+ξ3ξ4ξ5ξ6+ξ1ξ4ξ5ξ6+ξ2ξ4ξ5ξ6,
D10=ξ3ξ1ξ2ξ4+ξ3ξ1ξ2ξ5+ξ3ξ1ξ4ξ5+ξ3ξ2ξ4ξ5+ξ1ξ2ξ4ξ5+ξ3ξ1ξ2ξ6+ξ3ξ1ξ4ξ6+ξ3ξ2ξ4ξ6+ξ1ξ2ξ4ξ6+ξ3ξ1ξ5ξ6+ξ3ξ2ξ5ξ6+ξ1ξ2ξ5ξ6+ξ3ξ4ξ5ξ6+ξ1ξ4ξ5ξ6+ξ2ξ4ξ5ξ6−ξ3ρrγiνr−ρrξ4γiνr−ρrξ2kγr−ρrξ2kγr−ρrζqδcγr−ρrηγiγr−ξ3ρrδcγc−ρrξ2δcγc−ρvξ2kηv−ρvξ4kηv−ρvζqδcηv−ρvηγcηv−ρvkξ5ηv−ρvγiνrαv−ρvkγrαv−ρvδcγcαv−ρrγiνrξ6−ρrkγrξ6−ρrδcγcξ6, D11=γi[δnξ3ξ4+δnξ3ξ5+δnξ4ξ5+ξ3ξ4ξ5+δnξ3ξ6+δnξ4ξ6+ξ3ξ4ξ6+δnξ5ξ6+ξ3ξ5ξ6+ξ4ξ5ξ6], D12=δnξ3ξ1ξ2ξ4+δnξ3ξ1ξ2ξ5+δnξ3ξ1ξ4ξ5+δnξ3ξ2ξ4ξ5+δnξ1ξ2ξ4ξ5+ξ3ξ1ξ2ξ4ξ5+δnξ3ξ1ξ2ξ6+δnξ3ξ1ξ4ξ6+δnξ3ξ2ξ4ξ6+δnξ1ξ2ξ4ξ6+ξ3ξ1ξ2ξ4ξ6+δnξ3ξ1ξ5ξ6+δnξ3ξ2ξ5ξ6+δnξ1ξ2ξ5ξ6+ξ3ξ1ξ2ξ5ξ6+δnξ3ξ4ξ5ξ6+δnξ1ξ4ξ5ξ6+ξ3ξ1ξ4ξ5ξ6+δnξ2ξ4ξ5ξ6+ξ3ξ2ξ4ξ5ξ6+ξ1ξ2ξ4ξ5ξ6,
D13=ξ3ξ1ξ2ξ4ξ5+ξ3ξ1ξ2ξ4ξ6+ξ3ξ1ξ2ξ5ξ6+ξ3ξ1ξ4ξ5ξ6+ξ3ξ2ξ4ξ5ξ6+ξ1ξ2ξ4ξ5ξ6−ξ3ρrξ4γiνr−ρrξ2ξ4kγr−ρrξ2ζqδcγr−ρrξ4ηγiγr−ξ3ρrξ2δcγc−ρvξ2ξ4kηv−ρvξ2ζqδcηv−ρvξ4hγiηv−ρvξ2kξ5ηv−ρvξ4kξ5ηv−ρvζqδcξ5ηv−ρvηγiξ5ηv−ξ3ρvγiνrαv−ρvξ4γiνrαv−ρvξ2kγrαv−ρvξ4kγrαv−ρvζqδcγrαv−ρvηγiγrαv−ξ3ρvδcγcαv−ρvξ2δcγcαv−ξ3ρrγiνrξ6−ρrξ4γiνrξ6−ρrξ2kγrξ6−ρrξ4kγrξ6−ρrζqδcγrξ6−ρrηγiγrξ6−ξ3ρrδcγcξ6−ρrξ2δcγcξ6,
D14=γi[δnξ3ξ4ξ5+δnξ3ξ4ξ6+δnξ3ξ5ξ6+δnξ4ξ5ξ6+ξ3ξ4ξ5ξ6], D15=δnξ3ξ1ξ2ξ4ξ5+δnξ3ξ1ξ2ξ4ξ6+δnξ3ξ1ξ2ξ5ξ6+δnξ3ξ1ξ4ξ5ξ6+δnξ3ξ2ξ4ξ5ξ6+δnξ1ξ2ξ4ξ5ξ6+ξ3ξ1ξ2ξ4ξ5ξ6,
D16=ρvξ2ξ4kξ5ηv+ξ3ξ1ξ2ξ4ξ5ξ6−ρvξ2ζqδcξ5ηv−ρvξ4ηγiξ5ηv−ξ3ρvξ4γiνrαv−ρvξ2ξ4kγrαv−ρvξ2ζqδcγrαv−ρvξ4ηγiγrαv−ξ3ρvξ2δcγcαv−ξ3ρrξ4γiνrξ6−ρrξ2ξ4kγrξ6−ρrξ2ζqδcγrξ6−ρrξ4ηγiγrξ6−ξ3ρrξ2δcγcξ6, D17=γiδnξ3ξ4ξ5ξ6, D18=δnξ1ξ2ξ2ξ4ξ5ξ6.
If τ=0, then by using the rule of Descartes of sign, we can get there are no positive real roots.
Also by Routh-Hurwitz stability criterion, the real parts of the complex roots are also negative if μsI∗(ξ1+ξ2+ξ3+ξ4+ξ5+ξ6)−μsS∗γi+D3>0,(1−R0)<0,R0>1. Then the infection persistent steady state (S∗,E∗,I∗,Q∗,I∗c,R∗,V∗,W∗) is locally stable when R0<1.
If τ>0, then by using Rouche’s theorem, we have to prove that all roots of the characteristic Eq. (22) cannot have pure imaginary roots. Suppose that there exists w∈R such that λ=wi is a solution of (22). Now Eq. (22) becomes
−iw7 −(e−iwτμsI∗+D0)w6+i(e−iwτ[μsI∗D1−μsS∗D2]+D3)w5+(e−iwτ[μsI∗D4−μsS∗D5]+D6)w4 −i(e−iwτ[μsI∗D7−μsS∗D8]+D9)w3−(e−iwτ[μsI∗D10−μsS∗D11]+D12)w2 +i(e−iwτ[μsI∗D13−μsS∗D14]+D15)w+(e−iwτ[μsI∗D16−μsS∗D17]+D18)=0.(23)
Then,
E1+iE2w−E3w2 −iE4w3+E5w4+iE6w5−E7w6−iw7 =(−E∗1−iE∗2w+E∗3w2+iE∗4w3−E∗5w4−iE∗6w5+E∗7w6)(cosτw−isinτw)(24)
where E1=D18,E∗1=μsI∗D16−μsS∗D17, E2=D15,E∗2=μsI∗D13−μsS∗D14, E3=D12,E∗3=μsI∗D10−μsS∗D11, E4=D9,E∗4=μsI∗D7−μsS∗D8, E5=D6,E∗5=μsI∗D4−μsS∗D5, E6=D3,E∗6=μsI∗D1−μsS∗D2, E7=D0,E∗7=μsI∗, Equating the real and imaginary parts of (24), we get
E1−E3w2+E5w4−E7w6 =(−E∗1+E∗3w2−E∗5w4+E∗7w6)cosτw−(−E∗2w+E∗4w3−E∗6w5)sinτw(25)
E2w−E4w3+E6w5−w7 =(−E∗2w+E∗4w3−E∗6w5)cosτw+(−E∗1+E∗3w2−E∗5w4+E∗7w6)sinτw.(26)
Squaring both Eqs.(26), (27) and adding, we get
w14 +(E27−E6−E∗27)w12+(E26+E2E6+E∗5E∗7−E4−E5E7−E∗2E∗6−E∗26)w10+(E25+E3E7+E∗4E∗6 −E2−E4E6−E∗3E∗7−E∗25)w8+(E24+E∗1E∗7+E∗3E∗5−E1E7−E3E5−E∗24)w6 +(E23+E1E5+E∗2E∗4−E∗1E∗5−E2E4−E∗23)w4+(E2−E∗22)w2+(E21−E∗21)=0.(27)
Let z=w2 in (27)
F(z) =z7+(E27−E6−E∗27)z6+(E26+E2E6+E∗5E∗7−E4−E5E7−E∗2E∗6−E∗26)z5+(E25+E3E7 +E∗4E∗6−E2−E4E6−E∗3E∗7−E∗25)z4+(E24+E∗1E∗7+E∗3E∗5−E1E7−E3E5−E∗24)z3 +(E23+E1E5+E∗2E∗4−E∗1E∗5−E2E4−E∗23)z2+(E2−E∗22)z+(E21−E∗21)=0.(28)
If R0>1, then from Eq. (28), we can see that (E∗21−E 21) is strictly positive which implies F(0)<0. Thus, we can get atleast one positive real root. Hence, if R0>1 all the real parts of the roots of (22) are negative. Thus, the equilibrium position E∗ is stable when R0>1 for τ≥0.
During the second wave of the Corona virus, India experienced a high infection rate. We obtained data for this article from Tamilnadu, India. This current Omicron variant pandemic data of Tamilnadu, India is validated with our theoretical findings. The source of the data is specified by [28] and [23]. Tamil Nadu encountered its most memorable instance of the Omicron type of SARS-CoV-2 on December 15, 2021, as indicated by a traveller from another country. Three weeks after the first confirmed Omicron case was reported, Tamil Nadu was infected with the highly transmissible and rapidly spreading form of SARS-Cov-2. The data for this study is gathered from the state of Tamil Nadu (Chennai). As of March 11, 2022, there were 750606 positive cases, 750520 discharged cases, 48 deaths, 499 active cases, 42 positive cases on March 11, 2022, 86 recovered cases, and 3373 vaccinated cases. The state of Tamilnadu achieves a zero-death rate and a safe position against the spread of Omicron on March 11, 2022. We used Mathematica for plotting the solution. The values of the variables and parameters are listed in the Tables 3 and 4 below.
The susceptible individual curves for the systems SEIQIcRVW and SQIRV are depicted in Figs. 1–3, respectively. For the system SEIQIcRVW, we used the delay values (τ) 0.11, 0.14, and 0.16, and for the system SQIRV, we used the delay values (τ) 0.002, 0.003, and 0.004.
Figure 1: Susceptible people S(t) against time t with various τ for SEIQI cRVW
Figure 2: Susceptible people S(t) against time t with various τ for SQIRV
Figure 3: Susceptible people S(t) against time t with τ=0 for SEIQI cRVW and SQIRV
Fig. 4 demonstrates that when the exposed population decreases, the population of other compartments also decreases, while when the exposed population rises, the population of all related compartments rises.
Figure 4: Exposed people E(t) against time t with various τ for SEIQI cRVW
Figs. 5–7 illustrate the possible reduction in the Omicron infection rate. Fig. 5 demonstrates that when the Omicron variant was first discovered, its spread was rapid, and that the variant’s spread was reduced to a safe level when the government implemented quarantine and vaccination at a high rate. By adding more compartments from the models that came before it, the SEIQIcRVW model is able to keep the increase in infected individuals under control at a moderate rate. The state of Tamilnadu discovered on March 11, 2022, that Omicron’s death had not been caused by anyone. People were able to avoid contracting the SARS Cov-2 Omicron variant through vaccination against COVID-19.
Figure 5: Infected people I(t) against time t with various τ for SEIQI cRVW
Figure 6: Infected people I(t) against time t with various τ for SQIRV
Figure 7: Infected people I(t) against time t with τ=0 for SEIQI cRVW and SQIRV
The Quarantined individual level at time t is depicted in Figs. 8–10. When the government implemented the quarantine in Chennai at a high range, the spread of the disease was contained, and the situation in Chennai returned to normal.
Figure 8: Quarantined people Q(t) against time t with various τ for SEIQI cRVW
Figure 9: Quarantined people Q(t) against time t with various τ for SQIRV
Figure 10: Quarantined people Q(t) against time t with τ=0 for SEIQI cRVW and SQIRV
According to Fig. 11, the population of these four districts experiences a high rate of illness during the Omicron period, which begins on December 25 and ends on March 11, 2022. The infection rate gradually decreased to a low level and there were no deaths when people were vaccinated in accordance with government instructions.
Figure 11: Confirmed people S(t) against time t with various τ for SEIQI cRVW
Reproduction numbers of 0.66, 0.92, 0.63, and 0.06 for SEIQIcRVW and 0.02, 0.05, 0.073, and 0.074 for SQIRV are shown in Fig. 12. Contaminations are being eliminated from the host population when R0<1. However, if R0>1, the contaminations cause harm and become endemic, necessitating appropriate clinical treatments to stop the spread of the disease. If the delay value τ=0.014 for the system SEIQIcRVW and τ=0.003 for the system SQIRV, prominent oscillation is observed in the infected population. This could be interpreted as indicating that even though people recover over time, oscillations indicate that the exposed or asymptomatic population has a higher number of active cases than the infected population.
Figure 12: Infected range about various reproduction numbers for the system SEIQIcRVW and SQIRV
The rise in recovered rates for both systems in Chennai is depicted in Figs. 13–15. By balancing the recovered and infected rates with standard rates, the system SEIQIcRVW achieves stability.
Figure 13: Recovered people R(t) against time t with various τ for SEIQI cRVW
Figure 14: Recovered people R(t) against time t with various τ for SQIRV
Figure 15: Recovered people R(t) against time t with τ=0 for SEIQI cRVW and SQIRV
The rapid rise in the number of people being vaccinated is depicted in Figs. 16–18. As a result, the system’s infection rate significantly decreased, and the system became stable. The significance of vaccination to the Omicron virus control strategy is demonstrated by these figures.
Figure 16: Vaccinated people V(t) against time t with various τ for SEIQI cRVW
Figure 17: Vaccinated people V(t) against time t with various τ for SQIRV
Figure 18: Vaccinated people V(t) against time t with τ=0 for SEIQI cRVW and SQIRV
The effect of delayed SEIQIcRVW model construction is depicted in Fig. 19 as a decrease in reservoir individuals over time t. Figs. 20 and 21 show the stability of the Omicron mathematical model for the Chennai district at various delay values. The infection rate decreases for both the SEIQIcRVW and SQIRV systems following a rapid spread over a considerable period, as shown in Fig. 22. These systems control the infection and stop its spread within a few days.
Figure 19: Reservoir people W(t) against time t with various τ for SEIQI cRVW
Figure 20: Stability of the system SEIQI cRVW against time t with various τ
Figure 21: Stability of the system SQIRV against time t with various τ
Figure 22: Stability of the systems with τ=0
Novel delayed mathematical models for the Omicron B.1.1.529 SARS-Cov-2 Variant were developed in this paper. The stability of the two models has been examined and validated, and the principles of reproduction number calculated with this model are an outbreak threshold that determined whether or not the disease would spread further in the district Chennai of Tamilnadu. As the figures show, infection-free steady-state solutions are locally asymptotically stable when R0<1. The derived solutions show that the systems are locally unstable and will never become stable when R0>1 for an infection-free steady state. From all the data, we can say that the host community will be safe from the Omicron variant if more people are isolated, recovered, and vaccinated. We also found that the second wave of SARS Cov-2 Omicron variant spreads less if the intercessions are strictly followed. Based on our mathematical models and the Chennai data, the Omicron variant infection appears to have stabilized after approximately 25 days. This study will be beneficial for scientists who are working in the medical field. This work can be further extended to generalize with different fractional derivative models.
Acknowledgement: This study is supported via funding from Prince Sattam bin Abdulaziz University Project Number (PSAU/2023/R/1444). The first author is partially supported by the University Research Fellowship (PU/AD-3/URF/21F37237/2021 dated 09.11.2021) of Periyar University, Salem. The second author is supported by the fund for improvement of Science and Technology Infrastructure (FIST) of DST (SR/FST/MSI-115/2016).
Funding Statement: This study is supported via funding from Prince Sattam bin Abdulaziz University Project Number (PSAU/2023/R/1444). The first author is partially supported by the University Research Fellowship (PU/AD-3/URF/21F37237/2021 dated 09.11.2021) of PeriyarUniversity, Salem. The second author is supported by the fund for improvement of Science and Technology Infrastructure (FIST) of DST (SR/FST/MSI-115/2016).
Author Contributions: S. Dickson: Conceptualization, Visualization, Software, Resources, Formal analysis, Investigation, Writing-original draft. S. Padmasekaran: Investigation, Supervision, Formal analysis, Writing-review & editing. P. Kumar: Investigation, Supervision, Formal analysis, Writing-review & editing. Kottakkaran Sooppy Nisar: Investigation, Supervision, Formal analysis, Writing-review & editing. Hamidreza Marasi: Investigation, Supervision, Formal analysis, Writing-review & editing.
Availability of Data and Materials: Data sharing not applicable to this article as no datasets were generated or analyzed during the current study.
Conflicts of Interest: The authors declare that they have no conflicts of interest to report regarding the present study.
References
1. Byul Nim, K., Eunjung, K., Sunmi, L., Chunyoung, O. (2020). Mathematical model of COVID-19 transmission dynamics in South Korea: The impacts of travel, social distancing and early detectio. Processes, 8(10), 1304. [Google Scholar]
2. Chatzarakis, G. E., Dickson, S., Padmasekaran, S. (2022). A dynamic SIqIRV mathematical model with non-linear force of isolation, infection and cure. Nonautonomous Dynamical System, 9(1), 56–67. [Google Scholar]
3. Daniel Deborah, O. (2020). Mathematical model for the transmission of COVID-19 with nonlinear forces of infection and the need for prevention measure in nigeria. Journal of Infectious Diseases and Epidemiology, 6(5), 1–12. [Google Scholar]
4. Kiran, R., Madhumita, R., Syed, A., Taraphder, A. (2021). Effect of population migration and punctuated lockdown on the spread of infectious diseases. Nonautonomous Dynamical System, 8(1), 251–266. [Google Scholar]
5. Maski Tomochi, M. K. (2021). A mathematical model for COVID-19 pandemic-SIIR model: Effects of asymptomatic individuals. Journal of General and Family Medicine, 22(1), 5–14. [Google Scholar] [PubMed]
6. Sudhanshu Kumar, B., Jayanta Kumar, G., Susmita, S., Uttam, G. (2020). COVID-19 pandemic in India: A mathematcal model study. Nonlinear Dynamics, 102(1), 1–17. [Google Scholar]
7. Swati, T., Shaifu, G., Syed, A., Krishna, P., Baazaoui, R. (2021). Analysis of infectious disease transmission and prediction through SEIQR epidemic model. Nonautonomous Dynamical Systems, 8(1), 75–86. [Google Scholar]
8. Ashwin, M., Balamuralitharan, S., Poongodi, M., Mounir, H., Kaamran, R. et al. (2022). Stability and numerical solutions of second wave mathematical modeling on COVID-19 and omicron outbreak strategy of pandemic: Analytical and error analysis of approximate series solutions by using HPM. Mathematics, 10(3), 343. [Google Scholar]
9. Wang, B. G., Wang, Z. C., Wu, Y., Xiong, Y. P., Zhang, J. P. et al. (2022). A mathematical model reveals the in the influence of NPIs and vaccination on SARS-CoV-2 omicron variant. Nonlinear Dynamics, 111(4), 3937–3952. [Google Scholar] [PubMed]
10. Dickson, S., Padmasekaran, S., Kumar, P. (2023). Fractional order mathematical model for B.1.1.529 SARS-CoV-2 omicron variant with quarantine and vaccination. International Journal of Dynamics and Control, 11(5), 2215–2231. [Google Scholar]
11. Kumar, P., Suat Erturk, V. (2021). A case study of COVID-19 epidemic in India via new generalised caputo type fractional derivatives. Mathematical Methods in Applied Sciences, 2021, 1–14. [Google Scholar]
12. Kurkina E. S., Koltsova E. M. (2021). Mathematical modeling of the propagation of COVID-19 pandemic waves in the world. Computational Mathematics and Modeling, 32(2), 147–170. [Google Scholar]
13. Pakwan, R., Sherif, E., Arthi, I. (2021). A mathematical model of COVID-19 pandemic: A case study of bangkok, Thailand. Computational and Mathematical Methods in Medicine, 6664483, 1–11. [Google Scholar]
14. Kumar, P., Govindaraj, V., Zareen, A. K. (2022). Some novel mathematical results on the existence and uniqueness of generalized Caputo-type initial value problems with delay. AIMS Mathematics, 7(6), 10483–10494. [Google Scholar]
15. Liu, Z., Magal, P., Seydi, O., Webb, G. (2020). A COVID-19 epidemic model with latency period. Infectious Disease Modelling, 5, 323–337. [Google Scholar] [PubMed]
16. Yang, C., Yang, Y. L., Li, Z. W., Zhang, L. S. (2021). Modeling and analysis of COVID-19 based on a time delay dynamic model. AIMS Mathematical Biosciences and Engineering, 18(1), 154–165. [Google Scholar]
17. Sedighe, R., Noushin, A., Azadeh, S. (2020). Application of distribution-delay models to estimating the hospitalized mortality rate of COVID-19 according to delay effect of hospitalizations counts. Journal of Biostatatistics and Epidemiology, 6(4), 241–250. [Google Scholar]
18. Yang, F. F., Zhang, Z. Z. (2021). A time-delay COVID-19 propagation model considering supply chain transmission and hierarchical quarantine rate. Advances in Difference Equations, 2021, 191. [Google Scholar] [PubMed]
19. Hameed, K. E., Nizar, A., Hussein, B., Ebraheem, S. (2021). Delayed dynamics of SIR model for COVID-19. Open Journal of Modelling and Simulation, 9(2), 146–158. [Google Scholar]
20. Shidong, Z., Guoqiang, L., Tao, H., Xin, W., Junli, T. et al. (2021). Vaccination control of an epidemic model with time delay and its application to COVID-19. Nonlinear Dynamics, 106, 1279–1292. [Google Scholar]
21. Giovanni, N., Carla, P., Salvatore, T., Giorgia, V. (2022). A time-delayed deterministic model for the spread of COVID-19 with calibration on a real dataset. Mathematics, 10(4), 661. [Google Scholar]
22. Mohamed Zaitri, A., Christiana, J., Delfim, F. (2022). Stability analysis of delayed COVID-19 models. Axioms, 11(8), 400. [Google Scholar]
23. Dickson, S., Padmasekaran, S., Chatzarakis, G. E., Panetsos, L. (2022). SQIRV model for Omicron variant with time delay. Australian Journal of Analysis and Applications, 19(2), 1–16. [Google Scholar]
24. Diekmann, O., Heesterbeek, J., Metz, J. (1990). On the definition and computation of the basic reproduction number R0 in models for infectious disease. Journal of Mathematical Biology, 28, 365–382. [Google Scholar] [PubMed]
25. Van den Driessche, P., Watmough, J. (2002). Reproduction number and sub threshold epidemic equilibrium for compartmental models for disease transmission. Mathematical Biosciences, 180(1–2), 29–48. [Google Scholar] [PubMed]
26. Niculescu, S. I. (2001). Delay effects on stability: Lecture notes in control and information sciences, vol. 269. London, UK: Springer. [Google Scholar]
27. Dickson, S., Padmasekaran, S., Chatzarakis, G. E. (2022). Stability analysis of B.1.1.529 SARS-cov-2 omicron variant mathematical model: The impacts of quarantine and vaccination. Nonautonomous Dynamical System, 9(1), 290–306. [Google Scholar]
28. IndiaFightsCorona COVID-19 (2022). https://www.mygov.in/covid-19 (accessed on 11/03/2022). [Google Scholar]
Cite This Article
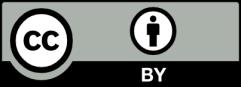
This work is licensed under a Creative Commons Attribution 4.0 International License , which permits unrestricted use, distribution, and reproduction in any medium, provided the original work is properly cited.