Open Access
ARTICLE
A Deep Learning Approach to Shape Optimization Problems for Flexoelectric Materials Using the Isogeometric Finite Element Method
1 College of Architecture and Civil Engineering, Xinyang Normal University, Xinyang, 464000, China
2 Henan Unsaturated Soil and Special Soil Engineering Technology Research Center, Xinyang Normal University, Xinyang, 464000, China
3 College of Intelligent Construction, Wuchang University of Technology, Wuhan, 430223, China
4 College of Civil Engineering and Architecture, Dalian University, Dalian, 116622, China
5 Henan International Joint Laboratory of Structural Mechanics and Computational Simulation, School of Architecture and Civil Engineering, Huanghuai University, Zhumadian, 463000, China
* Corresponding Authors: Xiaohui Yuan. Email: ; Yanming Xu. Email:
(This article belongs to the Special Issue: Structural Design and Optimization)
Computer Modeling in Engineering & Sciences 2024, 139(2), 1935-1960. https://doi.org/10.32604/cmes.2023.045668
Received 04 September 2023; Accepted 27 October 2023; Issue published 29 January 2024
Abstract
A new approach for flexoelectric material shape optimization is proposed in this study. In this work, a proxy model based on artificial neural network (ANN) is used to solve the parameter optimization and shape optimization problems. To improve the fitting ability of the neural network, we use the idea of pre-training to determine the structure of the neural network and combine different optimizers for training. The isogeometric analysis-finite element method (IGA-FEM) is used to discretize the flexural theoretical formulas and obtain samples, which helps ANN to build a proxy model from the model shape to the target value. The effectiveness of the proposed method is verified through two numerical examples of parameter optimization and one numerical example of shape optimization.Keywords
Optimization plays a vital role in the analysis of physical systems and in the science of decision making, aiming to maximize utility within resource constraints. Structural optimization problems can be broadly classified into three categories: size optimization [1–3], shape optimization [4–6], and topology optimization [7–10]. Size optimization [11–13] focuses on minimizing the thickness (e.g., cross-sectional area) of a specific structure type. Shape optimization [14–16] focuses on optimizing specific contours or shapes of designated domain boundary segments. Topology optimization [17–19] aims to minimize the material layout of the entire structure. Several approaches have been proposed to tackle optimization problems, including Newton’s method, Gauss-Newton method and some other methods [20–24]. Moreover, researchers have developed metaheuristic optimization algorithms like Particle Swarm Optimization [25], Differential Evolution [26], and other similar techniques [27–30].
Machine learning has gained significant attention in recent years as an alternative to classical optimization methods [31,32]. Since the 1980s, artificial neural network (ANN) [33–36] has become a prominent research topic in the field of artificial intelligence. ANN aims to simulate the information processing mechanism of neuronal networks in the human brain by constructing simplified models through various connection methods. ANN is composed of interconnected nodes, known as neurons, which represent specific output functions or activation functions. The connections between these nodes are represented by weighted values called weights, which act as the neural network’s memory. The output of the network is determined by the specific connection method, weight values, and activation functions utilized. Neural networks are often used to approximate algorithms or natural functions and to express logical strategies.
Kien et al. [37,38] proposed the Deep Lagrangian Method (DLM) as a new approach for solving size and shape optimization problems. This method cleverly combines Lagrange duality with deep learning techniques. Lagrangian duality theory provides a framework for solving the dual problems associated with primal constrained optimization problems. In the DLM, input data is utilized to train a deep neural network, with the parameters fine-tuned until the output closely aligns with the predicted values. By leveraging the interpolation capabilities of deep learning, the method effectively identifies the minimum input value. Consequently, this deep learning-based method enhances sensitivity analysis by making efficient use of a substantial amount of input data for neural network training.
Flexoelectricity was first introduced by Mashkevich and Tolpygo in 1957 [39], but its significance in bulk crystal materials was found to be weak, resulting in limited attention during the early stages. However, with the advancements in nanotechnology, significant strain gradients can now be observed at small scales, leading to the emergence of flexible electronics as a new avenue for studying size-related phenomena [40,41]. Unlike piezoelectric materials, where linear polarization is observed, different piezoelectric materials in flexible structures exhibit polarization that is dependent on the gradient. This makes flexoelectricity a more prevalent electromechanical coupling mechanism [42], as it can occur in any dielectric material, including those with centrally symmetric crystal structures [43–45]. Chen et al. [46] used a generalized n th-order perturbation and other isogeometry stochastic finite element method to quantitatively analyze the uncertainty of the mechanical properties of piezoelectric materials.
In the realm of flexoelectric effect analysis, numerous scholars have made remarkable contributions. El Dhaba et al. [47] and Awad et al. [48] examined the flexoelectric effects of materials with anisotropy and isotropy, correspondingly. Ghasemi et al. [49] introduced an isogeometric formula to calculate the flexoelectric effect based on the strain gradient expression of flexoelectricity, and presented 2D cantilever and 3D truncated pyramid models. Qu et al. [50] conducted a study on the buckling of piezoelectric semiconductor Reissner Mindlin plates. They determined the buckling load and mode, and also examined the wave particle resistance effect of flexible semiconductor materials [51]. Nguyen et al. [52] developed an isogeometric numerical model for the Maxwell-Wagner polarization effect in bilayer structures consisting of piezoelectric or flexoelectric materials. Liu et al. [53] constructed a real spatial phase field model using isogeometric analysis (IGA) to investigate the flexoelectric effect of ferroelectric materials at the nanoscale. Yin et al. [54] derived a curvature-based Euler-Bernoulli and Timoshenko beam model for flexoelectricity based on the coupled stress and flexoelectricity theory. They analyzed the impacts of flexoelectric effects, microstructure effects, and boundary conditions on the mechanical behavior of nanobeams using IGA. Gupta et al. [55] explored the effective piezoelectric and dielectric properties of boron nitride (BN) reinforced nanocomposites (BNRC), along with the surface flexoelectric effect. Their findings indicated that size-dependent flexoelectric and surface effects should be taken into account for accurate modeling of active nanostructures.
IGA represents substantial progress in computational mechanics [56], functioning as an expansion of the finite element technique. One of the key advantages of isogeometric analysis (IGA) is its ability to discretize partial differential equations using non-uniform rational B-spline basis functions. This feature allows engineers to directly perform numerical analysis from computer-aided design (CAD) models [57–61], ensuring both geometric accuracy [62–65] and eliminating the need for mesh generation. It is worth mentioning the contributions made by Jahanbin and Rahman [66] in developing engineering applications for uncertainty quantification through Stochastic Isogeometric Analysis (SIGA) in high-dimensional linear elasticity. Furthermore, Liu et al. [67] proposed a novel technique based on reduced basis vectors in SIGA for solving practical engineering problems. Chen et al. [68] utilized the radial integration technique for solving 2-D transient heat conduction problems via isogeometric boundary element analysis. Due to IGA’s ability to meet the continuity requirements of fourth-order partial differential equations (PDEs), it becomes possible to consider the flexible electrical properties by ensuring the necessary
Based on the aforementioned inspiration, we propose a method that combines ANN and IGA-FEM for solving shape optimization problems. In this method, the bending theoretical formulas are discretized using IGA-FEM to generate samples. These samples are then used to train the artificial neural network, which establishes a proxy model linking the model shape to the target value. Subsequently, this proxy model is utilized to solve shape optimization problems.
The content structure of this paper is set as follows: In the second section, the steps and improvements of ANN for optimization problems are introduced. In the third section, the theoretical formulas of flexographic problems and how to obtain initial samples by IGA-FEM method are expounded. Finally, in the fourth section, the effectiveness and accuracy of the method are verified by numerical examples.
2 Artificial Neural Network Methods for Optimization Problems
In order to make it easier for readers to understand, we first give a brief introduction to the composition and working principle of ANN. Please see [70–72] for detailed information.
ANN typically consist of three layers: input, hidden, and output. Among them, the input layer receives data, while the output layer produces the final result. Multiple hidden layers can exist. Each layer contains multiple neurons, with the number depending on specific requirements. The number of hidden layers in ANN can be customized, often involving multiple layers. These layers are fully connected, meaning that there are connections between adjacent layers, but no connections within the same layer.
Neural networks utilize two main processes, known as forward propagation and back propagation, to achieve self-learning. Forward propagation involves inputting samples into the neural network, which then passes through hidden layers until the output layer generates the desired output. The quality of the model’s fit is evaluated by assessing the loss function. Typically, the mean square error between the output layer results and sample labels is commonly utilized as the loss function for ANN, Fig. 1 illustrates an ANN network structure and neuronal node calculation process.
Figure 1: Neural network structure and neuron parameters
The input and output vectors are denoted as x =
also written as
where i = 1, 2,
where i = 1, 2,
where i = 1, 2,
In this study, we employed ANN to address the optimization problem. The methodology involved constructing a surrogate model of the optimization problem by training an artificial neural network, in order to find the optimal solution to the optimization problem. Notably, the ANN method is well-suited to address nonlinear optimization problems, thus enhancing its capability in handling complex systems. For instance,
where
Then, the mapping model of x= {
where
Using the mapping capabilities of neural networks, the initial sample can be expanded to
where
2.2 Dual Optimization Neural Network (DONN)
The accuracy of models in artificial neural networks during training and prediction is influenced by the neural network structure, including hidden layers and neurons. However, the optimal neural network structures vary for different problems. To address this, we propose a data-driven approach, referred to as Algorithm 1. This approach efficiently discovers a neural network structure that is better suited to the specific model, reducing the required time. The implementation concept of the algorithm is provided in the appendix in Fig. A1.
The DONN method first determines the optimal combination of a set of neural network layers and neurons through Algorithm 1 to establish a neural network structure, and then inputs the training data sets
The sigmoid function serves as the activation function for neuron nodes. Let us assume that the predicted value of the
Thus, as the value of the loss function approaches zero, the neural network’s predicted result and the actual result exhibit a smaller error. The role of a neural network optimizer is to continuously minimize the loss function through training on the data set. Different optimizers possess varying capabilities in reducing the loss function’s value. Furthermore, the choice of optimizer depends on the specific problem at hand, as different problems may require different optimizers. An appropriate optimizer not only enhances model accuracy but also minimizes the required training iterations and mitigates the risk of overfitting the neural network. In practice, it is possible to employ multiple optimizers for combined training within a neural network. Selecting the appropriate number of iterations further enhances training efficiency.
We divide the process of stochastic analysis with DONN into three stages:
(1) Preparation stage: Obtain a batch of training data sets through Latin-Hypercube sampling, leave samples that meet the constraints, obtain the initial data set
A small part of the normalized dataset is selected, and an artificial neural network is established through Algorithm 1.
(2) Model training and validation phase: the dataset is divided into training dataset and testing dataset. The former is utilized to construct the approximate function f that captures the relationship between the input and output. The latter is employed to assess the model’s generalization capability. The training process of the machine learning model involves searching for optimal parameters that minimize the loss function, while the testing process is conducted to validate the accuracy of the fitted function. Furthermore, it is necessary to post-process the predicted value to ensure its alignment with the input
where
(3) Application stage: The correctness of the mapping relationship
For the input variable
Figure 2: DONN flow charts for stochastic analysis
In the upcoming section, we will present an overview of the flexoelectric problem theory and provide guidance on obtaining the initial sample.
3 Initial Sample Acquisition for Flexoelectric Problems
In this section, we provide a comprehensive introduction to the theory of the flexoelectric problem and outline the process of obtaining initial samples using the IGA-FEM method.
3.1 Theory and Formulations of Flexoelectricity
The enthalpy density
Using the symbol
Consider the terms in Eq. (14)’s bracketed sections, which cover the direct and opposite flexoelectric effects. We reach the following results by integrating these terms across the physical domain
where the single material tensor
For purely piezoelectric dielectrics, we have
The normal electromechanical stresses
After substituting Eqs. (18) and (19) into Eq. (20), we obtain
The electrical enthalpy of a flexoelectric dielectric is given by
The work done by external forces, such as mechanical traction
where the sign
The kinetic energy of a system is defined as
where the
Upon substituting Eqs. (23)–(25) into Eq. (26), we obtain
By adding the variation operation within the integral operations, we obtain
Using the chain rule of variation and reordering the operations, we get
Now we can rewrite Eq. (28) as
To fulfill Eq. (31) for all possible values of
The inertia element is ignored in the case of a static situation, resulting in the following:
We may derive the weak version of the governing equation for flexoelectricity by putting Eqs. (18)–(22) into Eq. (33), as illustrated as
3.2 IGA-FEM Used to Discretize the Fourth-Order Partial Differential Equation of Flexoelectricity
In this section, we use B-spline basis functions to discretize the controlling Eq. (14). The B-spline basis function H is defined recursively by the Cox-de-Boor formula [74–77], and its expression is
when
Fig. 3 shows a particular multidimensional B-spline form function that is distinguished by knot vectors
and
Figure 3: The schematic diagram in illustrates the B-spline shape functions with a specific configuration of knot vectors
In Eqs. (38)–(43), the subscript
In Eqs. (44)–(46), the subscript
where the Poisson’s ratio is denoted by the symbol
In this section, we use three models to analyze the optimization problem, the first two models to analyze the parameter optimization problem, and the last model to analyze the shape optimization problem using the flexoelectric effect as an example.
4.1 Example of Structural Parameter Optimization
First example, we examine a truss structure consisting of bars with Young’s modulus E and density
Figure 4: A two-bar truss constrained by stress and displacement at the top
The weight of the truss is given by
In addition, the following constraints were applied to the stress:
and the displacement
with
where
We use the Latin hypercube sampling method for initial sampling, and remove the sample points that do not meet the constraints to obtain S1, which is substituted into the neural network as a training set for model training. The number of hidden layers of the neural network and the number of nodes per layer are determined by Algorithm 1. Fig. 5 shows the neural network structure determined by Algorithm 1. During training, we set up a two-layer optimizer, first using the Adam optimizer for fast fitting of the pre-model, and then using the gradient descent optimizer for fine-tuning the model. The gradient descent optimizer has a learning rate of
Figure 5: The first neural network structure built
In the neural network, the size of the data set directly affects the time spent in model training and the training effect. If the data set is too large, more iterations are required in the neural network training stage, and the time consumption increases significantly. At the same time, if the dataset is too small, the neural network will not be able to learn all the features, resulting in poor prediction effect after model training. Therefore, choosing a dataset of suitable size can effectively solve the above two problems. Fig. 6a shows the relationship between the loss function value of different optimizers and the number of iterations, and Fig. 6b shows the error curve under different sampling intervals, that is, different data sets of different sizes.
Figure 6: Decline curve of loss function and error analysis of data sets of different sizes
As can be seen from Fig. 6b, when
Figure 7: Error of DONN optimization results
After observing the decreasing law of the loss function and the relationship between the relative error and the number of iterations under different data and sizes, we make Adam optimizer perform 20,000 iterations, and GD optimizer perform 20,000 iterations. We restrict the range of
Using 1957 constrained samples as the training set, the solutions for DONN are given in Table 1, which are in good agreement with the exact solutions.
4.2 Weight Minimization of a Cantilever
Second example, we shift our focus to the cantilever beam illustrated in Fig. 8. The beam possesses a thin-walled cross-section depicted in Fig. 8b, with a thickness labeled as t. Each segment of the cross-section has a side length denoted as
Figure 8: Cantilever structure (a) and hollow square section (b)
In this case, we make an assumption that the thickness of the segment is significantly smaller in comparison to the side length, denoted as
Then, the weight of the beam can be calculated using the following equation:
The calculation of tip displacement can be expressed as [78]
The optimization problem of nested formulas can be expressed as follows:
with
In Table 2, the results of the DLM methods can be found.
Similar to the previous example, we set the range for
Figure 9: The second neural network structure built
Figure 10: Decline curve of loss function and error analysis of data sets of different sizes
Figure 11: The relationship between the error of the predicted result and the true value and the number of training times of the neural network
4.3 Shape Optimization of Flexoelectric Materials
The shape of flexoelectric materials that is most frequently researched is the truncated pyramid. In Fig. 12, the truncated pyramid model is displayed. While the bottom edge is immovable, the top edge is subject to evenly distributed forces of F magnitude. Tables 3 and 4 provide the dimensions of the model and the characteristics of the
Figure 12: The size of the truncated cone model structure and the uniformly distributed force on the upper edge
Figure 13: The distribution of total potential (a) and the distribution of strain in the
Firstly, we add control points to the truncated pyramid shape in Fig. 14. Then, we use the control points on the two waists of the trapezoid as input parameters for ANN and change them within a range of 20 percent, the maximum potential corresponding to the initial truncated pyramid is 0.374193. Using ANN, we find a shape with a larger potential than before.
Figure 14: The truncated pyramid shape control point and its movement direction, with points within the dashed line as random variables, vary within a 20 percent range
In terms of sample calculation, we select three control points on each waist, so that their x-direction coordinates fluctuate within a 20 percent range. 5000 sets of samples were calculated using IGA-FEM, and an ANN network was trained 50,000 times to obtain a mapping model of the maximum potential corresponding to the shape from the control point coordinates. The loss values of ANN are given in Fig. 15a, and the prediction error is shown in Fig. 15b.
Figure 15: Loss function decline curve (a) and relative error curve (b) between predicted value and true value during ANN training
It can be seen from the prediction error chart of loss value that the prediction error of neural network reaches a very low degree. Therefore, it can be used for the shape optimization of this problem. The shape change in the optimization process is shown in Fig. 16, The corresponding potential and strain distributions are given in Figs. 17 and 18, respectively. In addition, Fig. 19 shows the optimization effect curve under different training times.
Figure 16: The shape obtained by optimization under different iterations
Figure 17: The potential distribution corresponding to the shape is optimized under different iterations
Figure 18: The displacement distribution corresponding to the shape is optimized under different iterations
Figure 19: The maximum potential value corresponding to the optimized shape under different iterations (a) and the percentage increase of the optimized maximum potential value compared with the original model (b)
With the increase of the number of iterations, the prediction accuracy and optimization effect of the neural network gradually increased, and became stable after the number of iterations reached 32,000.
In this paper, ANN is used to optimize the structural parameters and shapes, and some techniques to improve the fitting effect are proposed. ANN only needs the samples of the corresponding problem for optimization, and then a proxy model could be built to deal with the optimization problem. Since the reliability of the optimization in this method depends on the accuracy of ANN, the idea of pre-training is used to find a suitable network structure, and the method of combining multiple optimizers is used to improve the prediction accuracy of ANN. To ensure the accuracy of samples, IGA-FEM is used to obtain high-precision samples, and the mapping model from shape control points to maximum potentials is established to solve the shape optimization problem. In the future, we will introduce deeper neural networks and meta-heuristic optimization algorithms, and study the extension of this method to shape optimization problems of more complex models.
Acknowledgement: The authors wish to express sincere appreciation to the reviewers for their valuable comments, which significantly improved this paper.
Funding Statement: The research in this article has been supported by a Major Research Project in Higher Education Institutions in Henan Province, with Project Number 23A560015.
Author Contributions: The authors confirm contribution to the paper as follows: study conception and design: Yu Cheng, Yajun Huang, Xiaohui Yuan; data collection: Yu Cheng, Shuai Li, Zhongbin Zhou; analysis and interpretation of results: Yu Cheng, Yajun Huang, Yanming Xu; draft manuscript preparation: Yu Cheng, Xiaohui Yuan, Yanming Xu. All authors reviewed the results and approved the final version of the manuscript.
Availability of Data and Materials: Data is available on request.
Conflicts of Interest: The authors declare that they have no conflicts of interest to report regarding the present study.
References
1. Svanberg, K. (1987). The method of moving asymptotes–A new method for structural optimization. International Journal for Numerical Methods in Engineering, 24(2), 359–373. [Google Scholar]
2. Schittkowski, K., Zillober, C., Zotemantel, R. (1994). Numerical comparison of nonlinear programming algorithms for structural optimization. Structural Optimization, 7(1–2), 1–19. [Google Scholar]
3. Fritze, D., Marburg, S., Hardtke, H. J. (2005). FEM-BEM-coupling and structural-acoustic sensitivity analysis for shell geometries. Advances in Analysis of Fluid Structure Interaction, 83(2), 143–154. [Google Scholar]
4. Marburg, S., Hardtke, H. J. (2001). Shape optimization of a vehicle hat-shelf: Improving acoustic properties for different load cases by maximizing first eigenfrequency. Computers & Structures, 79(20), 1943–1957. [Google Scholar]
5. Kostas, K., Ginnis, A., Politis, C., Kaklis, P. (2015). Ship-hull shape optimization with a T-spline based BEM-isogeometric solver. Computer Methods in Applied Mechanics and Engineering, 284, 611–622. [Google Scholar]
6. Lian, H., Wu, P. (2020). The shape optimization of underground caverns based on strain energy criterion via explicit interface tracking methods. International Journal of Advances in Engineering Sciences and Applied Mathematics, 12, 183–192. [Google Scholar]
7. Yuan, B., Ye, H., Li, J., Wei, N., Sui, Y. (2023). Topology optimization of geometrically nonlinear structures under thermal-mechanical coupling. Acta Mechanica Solida Sinica, 36(1), 22–33. [Google Scholar]
8. Ye, H., Yuan, B., Li, J., Zhang, X., Sui, Y. (2021). Geometrically nonlinear topology optimization of continuum structures based on an independent continuous mapping method. Acta Mechanica Solida Sinica, 34, 658–672. [Google Scholar]
9. Ye, H., Li, Z., Wei, N., Sui, Y. (2021). Fatigue topology optimization design based on distortion energy theory and independent continuous mapping method. Computer Modeling in Engineering & Sciences, 128(1), 297–314. https://doi.org/10.32604/cmes.2021.016133 [Google Scholar] [CrossRef]
10. Ye, H., Li, B., Shi, X., Zhang, Y. (2020). Quasi-static folding mechanical behavior analysis and optimization design for composite tube hinge. Journal of Mechanical Engineering, 56(5), 172. [Google Scholar]
11. Marburg, S., Schneider, S. (2003). Performance of iterative solvers for acoustic problems. Part I. Solvers and effect of diagonal preconditioning. Engineering Analysis with Boundary Elements, 27(7), 727–750. [Google Scholar]
12. Wen, P., Takano, N., Akimoto, S. (2018). General formulation of the first-order perturbation-based stochastic homogenization method using many random physical parameters for multi-phase composite materials. Acta Mechanica, 229(5), 2133–2147. [Google Scholar]
13. Marburg, S. (2002). Developments in structural-acoustic optimization for passive noise control. Archives of Computational Methods in Engineering, 9(4), 291–370. [Google Scholar]
14. Li, S., Trevelyan, J., Wu, Z., Lian, H., Wang, D. et al. (2019). An adaptive SVD-Krylov reduced order model for surrogate based structural shape optimization through isogeometric boundary element method. Computer Methods in Applied Mechanics and Engineering, 349, 312–338. [Google Scholar]
15. Chen, L., Lian, H., Liu, Z., Chen, H., Atroshchenko, E. et al. (2019). Structural shape optimization of three dimensional acoustic problems with isogeometric boundary element methods. Computer Methods in Applied Mechanics and Engineering, 355, 926–951. [Google Scholar]
16. Lian, H., Kerfriden, P., Bordas, S. (2016). Implementation of regularized isogeometric boundary element methods for gradient-based shape optimization in two-dimensional linear elasticity. International Journal for Numerical Methods in Engineering, 106(12), 972–1017. [Google Scholar]
17. Zhang, J., Zhang, W., Zhu, J., Xia, L. (2012). Integrated layout design of multi-component systems using xfem and analytical sensitivity analysis. Computer Methods in Applied Mechanics and Engineering, 245, 75–89. [Google Scholar]
18. Dühring, M. B., Jensen, J. S., Sigmund, O. (2008). Acoustic design by topology optimization. Journal of Sound and Vibration, 317(3), 557–575. [Google Scholar]
19. Chen, L., Lu, C., Lian, H., Liu, Z., Zhao, W. et al. (2020). Acoustic topology optimization of sound absorbing materials directly from subdivision surfaces with isogeometric boundary element methods. Computer Methods in Applied Mechanics and Engineering, 362, 112806. [Google Scholar]
20. Ye, H., Zhang, Y., Yang, Q., Zhang, B. (2019). Quasi-static analysis and multi-objective optimization for tape spring hinge. Structural and Multidisciplinary Optimization, 60(3–6), 2417–2430. [Google Scholar]
21. Ye, H., Dai, Z., Wang, W., Sui, Y. (2019). ICM method for topology optimization of multimaterial continuum structure with displacement constraint. Acta Mechanica Sinica, 35, 552–562. [Google Scholar]
22. Wang, W., Ye, H., Li, Z., Sui, Y. (2022). Stiffness and strength topology optimization for bi-disc systems based on dual sequential quadratic programming. International Journal for Numerical Methods in Engineering, 123, 4073–4093. [Google Scholar]
23. Wang, W., Liu, K., Wu, M., Li, H., Lv, P. et al. (2023). Instability-induced origami design by topology optimization. Acta Mechanica Solida Sinica, 36, 1–8. [Google Scholar]
24. Ho, C., Basdogan, C., Srinivasan, M. (2006). Numerical optimization. New York, NY, USA: Springer. [Google Scholar]
25. Hu, X., Eberhart, R. (2002). Solving constrained nonlinear optimization problems with particle swarm optimization. Proceedings of the Sixth World Multiconference on Systemics, Cybernetics and Informatics, vol. 5. Orlando. [Google Scholar]
26. Storn, R., Price, K. (1997). Differential evolution–A simple and efficient heuristic for global optimization over continuous spaces. Journal of Global Optimization, 11(4), 341–359. [Google Scholar]
27. Chen, L., Lian, H., Liu, Z., Gong, Y., Zheng, C. et al. (2022). Bi-material topology optimization for fully coupled structural-acoustic systems with isogeometric FEM-BEM. Engineering Analysis with Boundary Elements, 135, 182–195. [Google Scholar]
28. Lian, H., Chen, L., Lin, X., Zhao, W., Bordas, S. P. A. et al. (2022). Noise pollution reduction through a novel optimization procedure in passive control methods. Computer Modeling in Engineering & Sciences, 131(1), 1–18. https://doi.org/10.32604/cmes.2022.019705 [Google Scholar] [CrossRef]
29. Ghasemi, H., Park, H. S., Rabczuk, T. (2018). A multi-material level set-based topology optimization of flexoelectric composites. Computer Methods in Applied Mechanics and Engineering, 332, 47–62. [Google Scholar]
30. Kaveh, A. (2014). Advances in metaheuristic algorithms for optimal design of structures. Switzerland: Springer International Publishing. [Google Scholar]
31. Papadrakakis, M., Lagaros, N. D. (2002). Reliability-based structural optimization using neural networks and Monte Carlo simulation. Computer Methods in Applied Mechanics and Engineering, 191(32), 3491–3507. [Google Scholar]
32. Papadrakakis, M., Lagaros, N. D., Tsompanakis, Y. (1998). Structural optimization using evolution strategies and neural networks. Computer Methods in Applied Mechanics and Engineering, 156(1–4), 309–333. [Google Scholar]
33. Chen, L., Cheng, R., Li, S., Lian, H., Zheng, C. et al. (2022). A sample-efficient deep learning method for multivariate uncertainty qualification of acoustic-vibration interaction problems. Computer Methods in Applied Mechanics and Engineering, 393, 114784. [Google Scholar]
34. LeCun, Y., Bengio, Y., Hinton, G. (2015). Deep learning. Nature, 521(7553), 436–444. [Google Scholar] [PubMed]
35. Shen, X., Du, C., Jiang, S., Sun, L., Chen, L. (2023). Enhancing deep neural networks for multivariate uncertainty analysis of cracked structures by POD-RBF. Theoretical and Applied Fracture Mechanics, 125, 103925. [Google Scholar]
36. Atluri, S., Han, Z., Shen, S. (2003). Meshless local petrov-galerkin (MLPG) approaches for solving the weakly-singular traction & displacement boundary integral equations. Computer Modeling in Engineering & Sciences, 4(5), 507–518. https://doi.org/10.3970/cmes.2003.004.507 [Google Scholar] [CrossRef]
37. Kien, D. N., Zhuang, X. (2021). A deep neural network-based algorithm for solving structural optimization. Journal of Zhejiang University–SCIENCE A, 22(8), 609–620 (In Chinese). [Google Scholar]
38. Ye, H. L., Li, J. C., Yuan, B. S., Wei, N., Sui, Y. K. (2021). Acceleration design for continuum topology optimization by using Pix2pix neural network. International Journal of Applied Mechanics, 13(4), 2150042. [Google Scholar]
39. Mashkevich, V., Tolpygo, K. (1957). Electrical, optical and elastic properties of diamond type crystals. Soviet Physics JETP, 5(3), 435–439. [Google Scholar]
40. Ahmadpoor, F., Sharma, P. (2015). Flexoelectricity in two-dimensional crystalline and biological membranes. Nanoscale, 7(40), 16555–16570. [Google Scholar] [PubMed]
41. Qu, Y., Guo, Z., Zhang, G., Gao, X. L., Jin, F. (2022). A new model for circular cylindrical Kirchhoff–Love Shells incorporating microstructure and flexoelectric effects. Journal of Applied Mechanics, 89(12), 121010. [Google Scholar]
42. Qu, Y., Pan, E., Zhu, F., Jin, F., Roy, A. K. (2023). Modeling thermoelectric effects in piezoelectric semiconductors: New fully coupled mechanisms for mechanically manipulated heat flux and refrigeration. International Journal of Engineering Science, 182, 103775. [Google Scholar]
43. Yudin, P., Tagantsev, A. (2013). Fundamentals of flexoelectricity in solids. Nanotechnology, 24(43), 432001. [Google Scholar] [PubMed]
44. Nguyen, T. D., Mao, S., Yeh, Y. W., Purohit, P. K., McAlpine, M. C. (2013). Nanoscale flexoelectricity. Advanced Materials, 25(7), 946–974. [Google Scholar] [PubMed]
45. Zubko, P., Catalan, G., Tagantsev, A. K. (2013). Flexoelectric effect in solids. Annual Review of Materials Research, 43, 387–421. [Google Scholar]
46. Chen, L., Li, H., Guo, Y., Chen, P., Atroshchenko, E. et al. (2023). Uncertainty quantification of mechanical property of piezoelectric materials based on isogeometric stochastic FEM with generalized nth-order perturbation. Engineering with Computers, 1–21. [Google Scholar]
47. El Dhaba, A. (2019). A model for an anisotropic flexoelectric material with cubic symmetry. International Journal of Applied Mechanics, 11(3), 1950026. [Google Scholar]
48. Awad, E., El Dhaba, A. R., Fayik, M. (2022). A unified model for the dynamical flexoelectric effect in isotropic dielectric materials. European Journal of Mechanics–A/Solids, 95, 104618. [Google Scholar]
49. Ghasemi, H., Park, H. S., Alajlan, N., Rabczuk, T. (2020). A computational framework for design and optimization of flexoelectric materials. International Journal of Computational Methods, 17(1), 1850097. [Google Scholar]
50. Qu, Y., Jin, F., Yang, J. (2022). Buckling of a Reissner–Mindlin plate of piezoelectric semiconductors. Meccanica, 57(11), 2797–2807. [Google Scholar]
51. Qu, Y., Zhu, F., Pan, E., Jin, F., Hirakata, H. (2023). Analysis of wave-particle drag effect in flexoelectric semiconductor plates via Mindlin method. Applied Mathematical Modelling, 118, 541–555. [Google Scholar]
52. Nguyen, B., Zhuang, X., Rabczuk, T. (2018). Numerical model for the characterization of Maxwell-Wagner relaxation in piezoelectric and flexoelectric composite material. Computers & Structures, 208, 75–91. [Google Scholar]
53. Liu, C., Wang, J., Xu, G., Kamlah, M., Zhang, T. Y. (2019). An isogeometric approach to flexoelectric effect in ferroelectric materials. International Journal of Solids and Structures, 162, 198–210. [Google Scholar]
54. Yin, S., Wang, X., Wang, S., Zhang, G., Liu, J. et al. (2023). Curvature-based flexoelectric nanobeams: Analytical and numerical isogeometric analyses. Applied Mathematical Modelling, 124, 840–859. [Google Scholar]
55. Gupta, M., Meguid, S., Kundalwal, S. (2022). Synergistic effect of surface-flexoelectricity on electromechanical response of BN-based nanobeam. International Journal of Mechanics and Materials in Design, 18, 1–17. [Google Scholar]
56. Hughes, T. J., Cottrell, J. A., Bazilevs, Y. (2005). Isogeometric analysis: CAD, finite elements, NURBS, exact geometry and mesh refinement. Computer Methods in Applied Mechanics and Engineering, 194(39–41), 4135–4195. [Google Scholar]
57. Chen, L., Zhao, J., Lian, H., Yu, B., Atroshchenko, E. et al. (2023). A bem broadband topology optimization strategy based on taylor expansion and soar method-application to 2D acoustic scattering problems. International Journal for Numerical Methods in Engineering, 2023, 1–32. [Google Scholar]
58. Lu, C., Chen, L., Luo, J., Chen, H. (2023). Acoustic shape optimization based on isogeometric boundary element method with subdivision surfaces. Engineering Analysis with Boundary Elements, 146, 951–965. [Google Scholar]
59. Shen, X., Du, C., Jiang, S., Zhang, P., Chen, L. (2024). Multivariate uncertainty analysis of fracture problems through model order reduction accelerated SBFEM. Applied Mathematical Modelling, 125, 218–240. [Google Scholar]
60. Jiang, F., Zhao, W., Chen, L., Zheng, C., Chen, H. (2021). Combined shape and topology optimization for sound barrier by using the isogeometric boundary element method. Engineering Analysis with Boundary Elements, 124, 124–136. [Google Scholar]
61. Wang, Y., Liao, Z., Shi, S., Wang, Z., Poh, L. H. (2020). Data-driven structural design optimization for petal-shaped auxetics using isogeometric analysis. Computer Modeling in Engineering & Sciences, 122(2), 433–458. https://doi.org/10.32604/cmes.2020.08680 [Google Scholar] [CrossRef]
62. Li, H., Zhao, J., Guo, X., Cheng, Y., Xu, Y. et al. (2022). Sensitivity analysis of flexoelectric materials surrogate model based on the isogeometric finite element method. Frontiers in Physics, 10, 1111159. [Google Scholar]
63. Nguyen-Thanh, V. M., Zhuang, X., Rabczuk, T. (2019). A deep energy method for finite deformation hyperelasticity. European Journal of Mechanics–A/Solids, 80, 103874. [Google Scholar]
64. Schillinger, D., Dede, L., Scott, M. A., Evans, J. A., Borden, M. J. et al. (2012). An isogeometric design-through-analysis methodology based on adaptive hierarchical refinement of NURBS, immersed boundary methods, and T-spline CAD surfaces. Computer Methods in Applied Mechanics and Engineering, 249, 116–150. [Google Scholar]
65. Chen, L., Lian, H., Xu, Y., Li, S., Liu, Z. et al. (2023). Generalized isogeometric boundary element method for uncertainty analysis of time-harmonic wave propagation in infinite domains. Applied Mathematical Modelling, 114, 360–378. [Google Scholar]
66. Jahanbin, R., Rahman, S. (2020). Stochastic isogeometric analysis in linear elasticity. Computer Methods in Applied Mechanics and Engineering, 364, 112928. [Google Scholar]
67. Liu, Z., Yang, M., Cheng, J., Tan, J. (2021). A new stochastic isogeometric analysis method based on reduced basis vectors for engineering structures with random field uncertainties. Applied Mathematical Modelling, 89, 966–990. [Google Scholar]
68. Chen, L., Li, K., Peng, X., Lian, H., Lin, X. et al. (2021). Isogeometric boundary element analysis for 2D transient heat conduction problem with radial integration method. Computer Modeling in Engineering & Sciences, 126(1), 125–146. https://doi.org/10.32604/cmes.2021.012821 [Google Scholar] [CrossRef]
69. Chen, L., Lu, C., Zhao, W., Chen, H., Zheng, C. (2020). Subdivision surfaces-boundary element accelerated by fast multipole for the structural acoustic problem. Journal of Theoretical and Computational Acoustics, 28(2), 2050011. [Google Scholar]
70. Hamdia, K. M., Ghasemi, H., Bazi, Y., Alhichri, H., Alajlan, N. et al. (2019). A novel deep learning based method for the computational material design of flexoelectric nanostructures with topology optimization. Finite Elements in Analysis and Design, 165, 21–30. [Google Scholar]
71. Oishi, A., Yagawa, G. (2017). Computational mechanics enhanced by deep learning. Computer Methods in Applied Mechanics and Engineering, 327, 327–351. [Google Scholar]
72. Jung, J., Yoon, K., Lee, P. S. (2020). Deep learned finite elements. Computer Methods in Applied Mechanics and Engineering, 372, 113401. [Google Scholar]
73. Ghasemi, H., Park, H. S., Rabczuk, T. (2017). A level-set based IGA formulation for topology optimization of flexoelectric materials. Computer Methods in Applied Mechanics and Engineering, 313, 239–258. [Google Scholar]
74. Chen, L., Lian, H., Natarajan, S., Zhao, W., Chen, X. et al. (2022). Multi-frequency acoustic topology optimization of sound-absorption materials with isogeometric boundary element methods accelerated by frequency-decoupling and model order reduction techniques. Computer Methods in Applied Mechanics and Engineering, 395, 114997. [Google Scholar]
75. Chen, L., Lian, H., Liu, Z., Gong, Y., Zheng, C. et al. (2022). Bi-material topology optimization for fully coupled structural-acoustic systems with isogeometric FEM–BEM. Engineering Analysis with Boundary Elements, 135, 182–195. [Google Scholar]
76. Chen, L., Wang, Z., Peng, X., Yang, J., Wu, P. et al. (2021). Modeling pressurized fracture propagation with the isogeometric BEM. Geomechanics and Geophysics for Geo-Energy and Geo-Resources, 7(3), 51. [Google Scholar]
77. Chen, L., Zhang, Y., Lian, H., Atroshchenko, E., Ding, C. et al. (2020). Seamless integration of computer-aided geometric modeling and acoustic simulation: Isogeometric boundary element methods based on Catmull-Clark subdivision surfaces. Advances in Engineering Software, 149, 102879. [Google Scholar]
78. Christensen, P. W., Klarbring, A. (2008). An introduction to structural optimization, vol. 153. Netherlands: Springer Science & Business Media. [Google Scholar]
79. Abdollahi, A., Peco, C., Millan, D., Arroyo, M., Arias, I. (2014). Computational evaluation of the flexoelectric effect in dielectric solids. Journal of Applied Physics, 116(9), 093502. [Google Scholar]
Figure A1: Algorithm 1: Neural network structure random search algorithm
Cite This Article
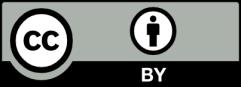
This work is licensed under a Creative Commons Attribution 4.0 International License , which permits unrestricted use, distribution, and reproduction in any medium, provided the original work is properly cited.