Open Access
ARTICLE
A Deep Learning Approach to Shape Optimization Problems for Flexoelectric Materials Using the Isogeometric Finite Element Method
1 College of Architecture and Civil Engineering, Xinyang Normal University, Xinyang, 464000, China
2 Henan Unsaturated Soil and Special Soil Engineering Technology Research Center, Xinyang Normal University, Xinyang, 464000, China
3 College of Intelligent Construction, Wuchang University of Technology, Wuhan, 430223, China
4 College of Civil Engineering and Architecture, Dalian University, Dalian, 116622, China
5 Henan International Joint Laboratory of Structural Mechanics and Computational Simulation, School of Architecture and Civil Engineering, Huanghuai University, Zhumadian, 463000, China
* Corresponding Authors: Xiaohui Yuan. Email: ; Yanming Xu. Email:
(This article belongs to the Special Issue: Structural Design and Optimization)
Computer Modeling in Engineering & Sciences 2024, 139(2), 1935-1960. https://doi.org/10.32604/cmes.2023.045668
Received 04 September 2023; Accepted 27 October 2023; Issue published 29 January 2024
Abstract
A new approach for flexoelectric material shape optimization is proposed in this study. In this work, a proxy model based on artificial neural network (ANN) is used to solve the parameter optimization and shape optimization problems. To improve the fitting ability of the neural network, we use the idea of pre-training to determine the structure of the neural network and combine different optimizers for training. The isogeometric analysis-finite element method (IGA-FEM) is used to discretize the flexural theoretical formulas and obtain samples, which helps ANN to build a proxy model from the model shape to the target value. The effectiveness of the proposed method is verified through two numerical examples of parameter optimization and one numerical example of shape optimization.Keywords
Cite This Article
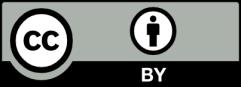
This work is licensed under a Creative Commons Attribution 4.0 International License , which permits unrestricted use, distribution, and reproduction in any medium, provided the original work is properly cited.