Open Access
ARTICLE
Comparative Analysis of ARIMA and LSTM Model-Based Anomaly Detection for Unannotated Structural Health Monitoring Data in an Immersed Tunnel
1 School of Naval Architecture, Ocean and Civil Engineering, Shanghai Jiao Tong University, Shanghai, 200240, China
2 Key Laboratory of Road and Bridge Detection and Maintenance Technology of Zhejiang Province, Hangzhou, 311305, China
3 Zhejiang Scientific Research Institute of Transport, Hangzhou, 310023, China
4 State Key Laboratory of Coal Mine Dynamics and Control, Chongqing University, Chongqing, 400044, China
5 Hong Kong-Zhuhai-Macao Bridge Authority, Zhuhai, 519060, China
* Corresponding Authors: Hao Tian. Email: ; Hui Wang. Email:
(This article belongs to the Special Issue: Computer-Aided Uncertainty Modeling and Reliability Evaluation for Complex Engineering Structures)
Computer Modeling in Engineering & Sciences 2024, 139(2), 1797-1827. https://doi.org/10.32604/cmes.2023.045251
Received 21 August 2023; Accepted 27 October 2023; Issue published 29 January 2024
Abstract
Structural Health Monitoring (SHM) systems have become a crucial tool for the operational management of long tunnels. For immersed tunnels exposed to both traffic loads and the effects of the marine environment, efficiently identifying abnormal conditions from the extensive unannotated SHM data presents a significant challenge. This study proposed a model-based approach for anomaly detection and conducted validation and comparative analysis of two distinct temporal predictive models using SHM data from a real immersed tunnel. Firstly, a dynamic predictive model-based anomaly detection method is proposed, which utilizes a rolling time window for modeling to achieve dynamic prediction. Leveraging the assumption of temporal data similarity, an interval prediction value deviation was employed to determine the abnormality of the data. Subsequently, dynamic predictive models were constructed based on the Autoregressive Integrated Moving Average (ARIMA) and Long Short-Term Memory (LSTM) models. The hyperparameters of these models were optimized and selected using monitoring data from the immersed tunnel, yielding viable static and dynamic predictive models. Finally, the models were applied within the same segment of SHM data, to validate the effectiveness of the anomaly detection approach based on dynamic predictive modeling. A detailed comparative analysis discusses the discrepancies in temporal anomaly detection between the ARIMA- and LSTM-based models. The results demonstrated that the dynamic predictive model-based anomaly detection approach was effective for dealing with unannotated SHM data. In a comparison between ARIMA and LSTM, it was found that ARIMA demonstrated higher modeling efficiency, rendering it suitable for short-term predictions. In contrast, the LSTM model exhibited greater capacity to capture long-term performance trends and enhanced early warning capabilities, thereby resulting in superior overall performance.Keywords
Cite This Article
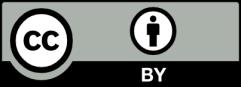
This work is licensed under a Creative Commons Attribution 4.0 International License , which permits unrestricted use, distribution, and reproduction in any medium, provided the original work is properly cited.