Open Access
ARTICLE
Heterophilic Graph Neural Network Based on Spatial and Frequency Domain Adaptive Embedding Mechanism
College of Information and Cyber Security, People’s Public Security University of China, Beijing, 100038, China
* Corresponding Author: Yijun Gu. Email:
(This article belongs to the Special Issue: Machine Learning-Guided Intelligent Modeling with Its Industrial Applications)
Computer Modeling in Engineering & Sciences 2024, 139(2), 1701-1731. https://doi.org/10.32604/cmes.2023.045129
Received 18 August 2023; Accepted 30 October 2023; Issue published 29 January 2024
Abstract
Graph Neural Networks (GNNs) play a significant role in tasks related to homophilic graphs. Traditional GNNs, based on the assumption of homophily, employ low-pass filters for neighboring nodes to achieve information aggregation and embedding. However, in heterophilic graphs, nodes from different categories often establish connections, while nodes of the same category are located further apart in the graph topology. This characteristic poses challenges to traditional GNNs, leading to issues of “distant node modeling deficiency” and “failure of the homophily assumption”. In response, this paper introduces the Spatial-Frequency domain Adaptive Heterophilic Graph Neural Networks (SFA-HGNN), which integrates adaptive embedding mechanisms for both spatial and frequency domains to address the aforementioned issues. Specifically, for the first problem, we propose the “Distant Spatial Embedding Module”, aiming to select and aggregate distant nodes through high-order random walk transition probabilities to enhance modeling capabilities. For the second issue, we design the “Proximal Frequency Domain Embedding Module”, constructing adaptive filters to separate high and low-frequency signals of nodes, and introduce frequency-domain guided attention mechanisms to fuse the relevant information, thereby reducing the noise introduced by the failure of the homophily assumption. We deploy the SFA-HGNN on six publicly available heterophilic networks, achieving state-of-the-art results in four of them. Furthermore, we elaborate on the hyperparameter selection mechanism and validate the performance of each module through experimentation, demonstrating a positive correlation between “node structural similarity”, “node attribute vector similarity”, and “node homophily” in heterophilic networks.Keywords
Cite This Article
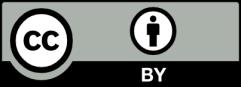
This work is licensed under a Creative Commons Attribution 4.0 International License , which permits unrestricted use, distribution, and reproduction in any medium, provided the original work is properly cited.