Open Access
ARTICLE
A Deep Learning Approach for Landmines Detection Based on Airborne Magnetometry Imaging and Edge Computing
1 Faculty of Computing and Information Technology, King Abdulaziz University, Jeddah, Saudi Arabia
2 Department of Computer Science and Engineering, Thapar Institute of Engineering and Technology, Deemed to be University, Patiala, India
* Corresponding Author: Ahmed Barnawi. Email:
(This article belongs to the Special Issue: Machine Learning Empowered Distributed Computing: Advance in Architecture, Theory and Practice)
Computer Modeling in Engineering & Sciences 2024, 139(2), 2117-2137. https://doi.org/10.32604/cmes.2023.044184
Received 23 July 2023; Accepted 15 November 2023; Issue published 29 January 2024
Abstract
Landmines continue to pose an ongoing threat in various regions around the world, with countless buried landmines affecting numerous human lives. The detonation of these landmines results in thousands of casualties reported worldwide annually. Therefore, there is a pressing need to employ diverse landmine detection techniques for their removal. One effective approach for landmine detection is UAV (Unmanned Aerial Vehicle) based Airborne Magnetometry, which identifies magnetic anomalies in the local terrestrial magnetic field. It can generate a contour plot or heat map that visually represents the magnetic field strength. Despite the effectiveness of this approach, landmine removal remains a challenging and resource-intensive task, fraught with risks. Edge computing, on the other hand, can play a crucial role in critical drone monitoring applications like landmine detection. By processing data locally on a nearby edge server, edge computing can reduce communication latency and bandwidth requirements, allowing real-time analysis of magnetic field data. It enables faster decision-making and more efficient landmine detection, potentially saving lives and minimizing the risks involved in the process. Furthermore, edge computing can provide enhanced security and privacy by keeping sensitive data close to the source, reducing the chances of data exposure during transmission. This paper introduces the MAGnetometry Imaging based Classification System (MAGICS), a fully automated UAV-based system designed for landmine and buried object detection and localization. We have developed an efficient deep learning-based strategy for automatic image classification using magnetometry dataset traces. By simulating the proposal in various network scenarios, we have successfully detected landmine signatures present in the magnetometry images. The trained models exhibit significant performance improvements, achieving a maximum mean average precision value of 97.8%.Keywords
Cite This Article
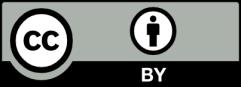
This work is licensed under a Creative Commons Attribution 4.0 International License , which permits unrestricted use, distribution, and reproduction in any medium, provided the original work is properly cited.