Open Access
ARTICLE
A Secure and Cost-Effective Training Framework Atop Serverless Computing for Object Detection in Blasting Sites
1 Department of Computer and Science, Shanghai Jiaotong University, Shanghai, 200240, China
2 Aerospace System Engineering Shanghai, Shanghai, 200240, China
3 State Key Laboratory of Precision Blasting, Jianghan University, Wuhan, 430056, China
4 Beijing Microelectronics Technology Institute, Beijing, 100076, China
* Corresponding Authors: Quanmin Xie. Email: ; Mengke Tian. Email:
(This article belongs to the Special Issue: Machine Learning Empowered Distributed Computing: Advance in Architecture, Theory and Practice)
Computer Modeling in Engineering & Sciences 2024, 139(2), 2139-2154. https://doi.org/10.32604/cmes.2023.043822
Received 13 July 2023; Accepted 15 November 2023; Issue published 29 January 2024
Abstract
The data analysis of blasting sites has always been the research goal of relevant researchers. The rise of mobile blasting robots has aroused many researchers’ interest in machine learning methods for target detection in the field of blasting. Serverless Computing can provide a variety of computing services for people without hardware foundations and rich software development experience, which has aroused people’s interest in how to use it in the field of machine learning. In this paper, we design a distributed machine learning training application based on the AWS Lambda platform. Based on data parallelism, the data aggregation and training synchronization in Function as a Service (FaaS) are effectively realized. It also encrypts the data set, effectively reducing the risk of data leakage. We rent a cloud server and a Lambda, and then we conduct experiments to evaluate our applications. Our results indicate the effectiveness, rapidity, and economy of distributed training on FaaS.Keywords
Cite This Article
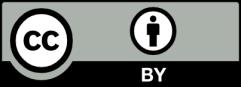
This work is licensed under a Creative Commons Attribution 4.0 International License , which permits unrestricted use, distribution, and reproduction in any medium, provided the original work is properly cited.