Open Access
ARTICLE
An Intelligent MCGDM Model in Green Suppliers Selection Using Interactional Aggregation Operators for Interval-Valued Pythagorean Fuzzy Soft Sets
1 School of Mathematical Sciences, Zhejiang Normal University, Jinhua, 321004, China
2 Department of Mathematics and Statistics, University of South Florida, Tampa, FL 33620-5700, USA
3 School of Mathematical and Statistical Sciences, North-West University, Mafikeng Campus, Mmabatho, 2735, South Africa
4 Department of Mathematics, University of Sargodha, Sargodha, 40100, Pakistan
5 Section of Mathematics, International Telematic University Uninettuno, Corso Vittorio Emanuele II, Roma, 00186, Italy
6 Near East University, Operational Research Center in Healthcare, Nicosia/Mersin, 99138, Turkey
7 Department of Statistics and Operations Research, College of Science, King Saud University, Riyadh, 11451, Saudi Arabia
* Corresponding Author: Wen-Xiu Ma. Email:
(This article belongs to the Special Issue: Advances in Ambient Intelligence and Social Computing under uncertainty and indeterminacy: From Theory to Applications)
Computer Modeling in Engineering & Sciences 2024, 139(2), 1829-1862. https://doi.org/10.32604/cmes.2023.030687
Received 18 April 2023; Accepted 21 June 2023; Issue published 29 January 2024
Abstract
Green supplier selection is an important debate in green supply chain management (GSCM), attracting global attention from scholars, especially companies and policymakers. Companies frequently search for new ideas and strategies to assist them in realizing sustainable development. Because of the speculative character of human opinions, supplier selection frequently includes unreliable data, and the interval-valued Pythagorean fuzzy soft set (IVPFSS) provides an exceptional capacity to cope with excessive fuzziness, inconsistency, and inexactness through the decision-making procedure. The main goal of this study is to come up with new operational laws for interval-valued Pythagorean fuzzy soft numbers (IVPFSNs) and create two interaction operators-the interval-valued Pythagorean fuzzy soft interaction weighted average (IVPFSIWA) and the interval-valued Pythagorean fuzzy soft interaction weighted geometric (IVPFSIWG) operators, and analyze their properties. These operators are highly advantageous in addressing uncertain problems by considering membership and non-membership values within intervals, providing a superior solution to other methods. Moreover, specialist judgments were calculated by the MCGDM technique, supporting the use of interaction AOs to regulate the interdependence and fundamental partiality of green supplier assessment aspects. Lastly, a statistical clarification of the planned method for green supplier selection is presented.Keywords
Green supply chain management (GSCM) is a tactical strategy that implements sustainable practices throughout the supply chain. Due to increasing concerns about the sustainability of the environment, the concept of GSCM acquired severe recognition in the past few years. One of the primary objectives of GSCM is to minimize a supply chain’s emissions and adverse environmental effects. As the world’s economic system grows, more significant resources are used. Still, resource depletion and degradation of the environment have increased indigenous, carrying more attention from researchers, businesses, the general public, and authorities globally [1]. As a consequence, approaches to sustainable development have been established, and many kinds of reinforced environmental regulations, laws, and policies have been placed that combine economic expansion with a sustainable environment [2]. GSCM is being demonstrated to be a viable approach for addressing the problem mentioned earlier. This type of administrative strategy infuses environmentally conscious thinking through the operational phases of the supply chain, including design, which is buying goods manufacturing, transportation, preservation, advertisement, and transaction [3]. The supplier is upstream and in the green supply chain, setting the basis for inter- and intra-organizational efficiency [4]. Consequently, selecting the most capable green supplier is essential for small businesses to contribute to environmental preservation while retaining their fundamental competitive advantage in supply chain management.
Multi-criteria group decision-making is deemed the most suitable method for determining the optimal alternative based on criteria or attributes. However, decisions in real-life situations may involve vague or unclear goals and limitations. In DM, dealing with uncertainties and ambiguities can be a challenge. To address this issue, fuzzy sets (FS) were introduced by Zadeh [5]. Later, interval-valued fuzzy sets (IVFS) were presented by Turksen [6] to handle situations where experts consider membership degrees (MD) in intervals during the DM process. However, FS and IVFS are limited because they do not provide information about alternative non-membership degrees (NMD). Atanassov [7] created the intuitionistic fuzzy set (IFS) to address these limitations. Wang et al. [8] added several operations and AOs for IFS, including the Einstein product and sum. Furthermore, the cubic intuitionistic fuzzy set (CIFS) was proposed by Garg et al. [9] to develop the concept of IFS further. Atanassov [10] extended the notion of IFS to the interval-valued intuitionistic fuzzy set (IVIFS). Torağay et al. [11] utilized the Delphi method based on a 2-Tuple fuzzy Linguistic representation and the TOPSIS model for faculty evaluation. To address these limitations, Yager [12] proposed the Pythagorean fuzzy set (PFS), which resolves issues with the linear relationship between MD and NMD and cases where
The earlier theories have various applications but may be ineffective in parametric chemistry. Molodtsov [27] developed the theory of soft sets (SS) to address uncertainty and misperceptions. Fu et al. [28] then developed some essential operations for interval-valued soft sets and demonstrated a technique for DM. Khalil et al. [29] established a novel DM methodology based on FSS. Other researchers have also proposed different variations of FSS, such as intuitionistic fuzzy soft sets (IFSS) [30]. Arora et al. [31] proposed a method that uses AOs for IFSS. Jiang et al. [32] developed the interval-valued IFSS (IVIFSS) with basic operations and their possessions. Zulqarnain et al. [33] proposed the TOPSIS technique for multiple attribute decision-making (MADM) problems in IVIFSS using the correlation coefficient (CC). Peng et al. [34] combined PFS and SS to create PFSS with some basic operations. Zulqarnain et al. [35,36] developed AOs and interaction AOs for PFSS and used their developed operators in green supply chain management (GSCM). Kirişci et al. [37] proposed a new DM approach that uses PFSS. Zulqarnain et al. [38,39] prolonged the Einstein-ordered weighted AOs for PFSS. Siddique et al. [40] developed a novel DM method based on a score matrix for PFSS to solve daily life hurdles. Zulqarnain et al. [41] extended the AOs for IVPFSS and presented a DM technique to solve the MAGDM problem. Selecting sustainable suppliers is vital to managing a sustainable supply chain, ensuring all stakeholders adhere to environmental, social, and ethical standards. Qiu et al. [42] chose the best private partner using a single-valued neutrosophic set. Chatterjee et al. [43] suggested that to create a healthier environment, it is important for practitioners to identify the key criteria necessary for implementing sustainable policies, particularly in the rapidly growing electronics sector. Stevic et al. [44] utilized the DM trial and evaluation laboratory method based on rough numbers to select suppliers for a construction company. Stojic et al. [45] established a new MCDM model to select suppliers for a polyvinyl chloride carpentry company. Stevic et al. [46] introduced a new method for measuring alternatives and ranking them according to compromise solutions for sustainable supplier selection in the healthcare industry.
The IVPFSS is a type of structure that combines two different systems, IVPFS and SS. These systems are used to handle information that is both certain and uncertain. When making decisions, it is important to be able to combine information from different sources to get a comprehensive understanding of the situation. However, the current tools for IVPFSS are not very effective when dealing with imprecise or uncertain data. This can make it difficult to determine the best course of action. Researchers have been exploring using interaction AOs for IVPFSS to address this issue. These AOs, such as IVPFSIWA and IVPFSIWG, can be compared to other fusion extensions of FS. The models that determine the overall NMD are based on the compatible NMD interval values. However, this approach can lead to adverse outcomes and make it difficult to assess alternatives accurately. To improve the effectiveness of these models, researchers are exploring the use of interval-valued Pythagorean fuzzy soft numbers (IVPFSNs) and their interactions. The current AOs used to check data concepts are ineffective and can lead to imprecise outcomes. By incorporating IVPFSNs and their interactions, researchers hope to develop better tools for decision-making that can handle uncertain and imprecise data more effectively. Let
The interactional AOs for IVPFSS are aimed at addressing the limitations of existing AOs. The traditional AOs do not consider the interaction between different attributes or experts, which can lead to biased or inaccurate DM. The interactional AOs allow for viewing interaction between different attributes or experts, which can lead to more comprehensive and accurate DM. These operators consider the weights assigned to different attributes or experts and their agreement and disagreement levels. Therefore, by introducing interactional AOs for IVPFSS, we can improve the accuracy and reliability of DM in various fields such as engineering, finance, medical diagnosis, sustainable supplier selection, and other areas where uncertainty and ambiguity are common. Sustainable supplier selection minimizes negative environmental and societal impacts while maximizing positive outcomes, such as increased efficiency and profitability. This is accomplished by integrating sustainability criteria into the supplier evaluation process. Evaluating potential suppliers involves considering quality, price, delivery time, and reliability factors to determine the best fit for a particular business or organization. The selection and assessment of suppliers are critical for ensuring the success of supply chain management and achieving the desired results. The literature highlights the need for an MCGDM method for sustainable supplier selection that considers environmental concerns and expert opinions. To address these limitations, we propose a strategy that uses IVPFSS information. IVPFSS is a hybrid intellectual structure that incorporates both IVPFSS and fuzzy soft information to improve the sorting process and help decision-makers deal with complex and incomplete information. Based on research findings, IVPFSS plays a crucial role in DM by integrating multiple sources into a single value.
• However, when dealing with MD and NMD, existing AOs may not yield accurate results, making an improved sorting process essential. Based on research findings, IVPFSS plays a crucial role in DM by consolidating multiple sources into a single value. Although combining IVPFS and SS is a widely recognized concept, integration with the IVPFSS background has been lacking. To address this, we propose extending IVPFSS by introducing interaction AOs with their fundamental properties. Our investigation aims to expand the concept of IVPFSS by introducing interaction operators based on rough data, with the following objectives:
• Extend the PFSS to IVPFSS and develop interaction AOs to effectively handle multiple attributes in DM processes.
• While interaction AOs for IVPFSS are a reputable and effective method for evaluating attributes in the DM process, they may not always provide accurate results. To address this, we present new operational laws for IVPFSNs.
• Introduce the IVPFSIWA and IVPFSIWG operators and their essential properties, utilizing the established operational laws.
• Design a new method using the established operators to address MCGDM problems in the IVPFSS environment.
• Sustainable supplier selection is crucial for sustainable supply chain management and a significant step in professional development. It aims to ensure that all aspects meet concrete conditions.
• Compare the newly proposed MCGDM technique and existing methods to evaluate the effectiveness and superiority of the new approach.
This paper’s organization content is divided into five sections. Section 2 overviews some crucial concepts that will facilitate the subsequent study. Section 3 introduces new operational laws for IVPFSNs and presents the IVPFSIWA and IVPFSIWG operators based on these laws. These operators are developed to improve the boundaries of communicating indefinite and unreliable data in real-life surroundings. Section 4 proposes an MCGDM method using the newly developed interaction AOs. This section describes how the proposed method can be used to select sustainable suppliers effectively. To demonstrate the practicality of the approach, we provide a numerical example of sustainable supplier selection in this section. Finally, Section 5 compares the proposed technique with existing approaches, showing its efficiency and effectiveness. The comparison will help to understand the advantages of the proposed method over other existing methods.
This section lays the foundation for the subsequent work by providing essential definitions.
2.1 Definition [5]
where
2.2 Definition [6]
where
2.3 Definition [10]
where
Furthermore,
2.4 Definition [23]
where
2.5 Definition [27]
A soft set is defined as a pair
Also, it can be defined as follows:
2.6 Definition [32]
An interval-valued intuitionistic fuzzy soft set is defined as a pair
where
2.7 Definition [41]
An interval-valued Pythagorean fuzzy soft set is defined as a pair
where
Also,
Simply IVPFSN can be expressed as
2.8 Definition [41]
Let
1.
2.
3.
4.
For the collection of IVPFSNs
It is noticed that the IVPFSWA and IVPFSWG operators provide some unattractive outcomes in certain conditions, as mentioned in 1.1. To overwhelm such consequences, we present the interaction AOs for IVPFSNs.
3 Interaction Aggregation Operators for Interval Valued Pythagorean Fuzzy Soft Sets
This section will describe the concept of interaction operational laws in the context of IVPFSNs. Utilizing these operational laws, we will introduce the IVPFSIWA and IVPFSIWG operators.
3.1 Interaction Operational Laws for IVPFSNs
Let
1.
2.
3.
4.
3.2 Interval Valued Pythagorean Fuzzy Soft Interaction Weighted Average Operator
Suppose we have a collection of IVPFSNs denoted by
3.2.1 Theorem
Let
The weight vectors for experts and attributes are denoted by
Proof:
To demonstrate the IVPFSIWA operator, we will employ the principle of mathematical induction.
For
For
This demonstrates that the theorem given above is true for
For
Therefore, it holds for
3.2.2 Example
Suppose
Applying the theorem mentioned above
3.3 Properties of IVPFSIWA Operator
If
Proof: As we know that all
As
Let
Proof: As we know that
Similarly,
Let IVPFSIWA (
Using the score function, we have
Therefore, utilizing the ordering relation between two IVPFSNs, we can obtain the following:
Prove that
Proof: Suppose we have an IVPFSN represented by
So,
3.4 Interval Valued Pythagorean Fuzzy Soft Interaction Weighted Geometric Operator
Suppose we have a collection of IVPFSNs denoted by
Let
The weight vectors for experts and attributes are denoted by
Proof: To demonstrate the IVPFSIWG operator, we will employ the principle of mathematical induction:
For
For
This demonstrates that the theorem given above is true for
For
Therefore, it holds for
Suppose
Applying the theorem mentioned above
If
Proof: As we know that all
IVPFSIWG (
As
Let
Proof: As we know that
Similarly, we can prove that
Let
So, by using the score function, we have
Therefore, utilizing the ordering relation between two IVPFSNs, we can obtain the following:
Prove that
Proof: Suppose we have an IVPFSN represented by
So,
4 Proposed MCGDM Approach Based on Developed Operators
This section presents a solution for MCGDM problems using the newly proposed IVPFSIWA and IVPFSIWG operators, along with numerical examples.
Let
To solve the MCGDM problem using the proposed IVPFSIWG and IVPFSIWA operators, the following procedure can be applied concisely:
Step-1: Obtain a decision matrix using IVPFSNs for alternatives relative to experts.
where
Step-2: Transforming the decision matrix by normalizing it and transforming the evaluations of cost-type parameters into evaluations of benefit-type parameters through the normalization equation.
Step-3: Using the IVPFSIWA and IVPFSIWG operators that have been developed, the IVPFSNs
Step-4: Determine the score values of
Step-5: Select the maximum score value alternative and examine the ranking.
A case study on sustainable supplier selection could involve evaluating and choosing suppliers based on various sustainability factors, such as environmental impact, ethical practices, and social responsibility. The goal of the case study would be to determine which suppliers meet the sustainability criteria and should be considered for partnership. A comprehensive review of the supplier’s policies on sustainability, procedures, and achievement reports may be part of the procedure. The information provided might be utilized to create an evaluation matrix that is then examined employing an MCGDM methodology. Employing experts with sufficient knowledge and experience in sustainable supplier selection may help execute the case study. Experts can offer comments regarding the sustainability regulations, analyze the suppliers, and give details about the final DM method. A case study could highlight the significance of prospective supplier selection, the real-world execution of MCGDM methods in supplier selection, and the importance of experts in the fields of the DM approach. Moreover, the case research results can offer helpful insight into the ability and effectiveness of using MCGDM approaches for better permanent supplier selection. Quality of products and services, delivery performance, cost, financial stability, technical expertise, sustainability, innovation, new product development, supply chain management, communication and collaboration, reputation, and other factors are important in supplier selection. In this investigation, we merely investigate the following factors to determine the most appropriate supplier:
• Quality of products and services
• Cost
• Sustainability
• Reputation
4.2.1 Quality of Products and Services
Quality is a significant factor in supplier selection since it determines the goods or services delivered to the consumer. Poor-quality goods or services from a supplier may culminate in consumers’ frustration, product recalls, and business loss. Conversely, a supplier who regularly produces goods and services of superior quality may boost and strengthen a company’s credibility, advertise client fulfilment, and build an ongoing relationship with the organization. A few of the most significant quality factors in a supplier’s services and goods are
• Product reliability and durability
• Consistently fulfilling product specifications and standards
• Timeliness and accuracy of delivery
• Responsiveness to consumer inquiries and complaints
• Technical assistance and after-sales services.
Before and during the business interaction, companies must evaluate the superiority of a supplier’s goods or services to ensure that the supplier fulfils their requirements and meets a suitable quality level.
When choosing suppliers for a business, the cost is a significant factor. A supplier’s product or service’s monetary value is referred to as cost. It involves features such as the cost of the product, handling and transportation fees, taxes, and additional related costs. When selecting a supplier, it is important to look at the pricing procedures, cost structures, and overall accessibility to ensure that the price of the goods and services they provide equates with the organisation’s budget and goal capabilities. Also, consider the cost of maintaining a relationship and any subsequent fees that may occur. By thoroughly investigating the expense of contracting with a supplier, a business can make sensible choices to get the best value for its money while preserving its different supplier selection specifications.
As firms strive to limit their environmental effect and promote ethical business practices, sustainability is becoming increasingly essential in supplier selection. Sustainable factors in supplier selection comprise a supplier’s environmental record, energy consumption, reducing waste, and utilization of resources. Corporations can also consider a supplier’s dedication to sustainability via accreditation and initiatives such as eco-labelling, recycling initiatives, and volunteerism in the community. As consumers and stakeholders demand firms their duties with integrity and reduce their impact on the environment, sustainably becomes becoming increasingly important in supplier selection. The environmental factors that influence sustainability may consist of the following:
• Resource exhaustion and preservation
• Energy consumption and effectiveness
• Water usage and preservation
• Emissions and pollution control
• Waste administration and reprocessing
• Climate change and greenhouse gas emissions
• Biodiversity and habitation conservation
• Land use and conservation
• Ecosystem healthiness and resilience
• Natural resource management and safety.
These elements can impact a company’s or supplier’s long-term existence.
When choosing a provider, reputation is an important topic to remember. A reputable supplier is more likely to deliver excellent services and goods, be reliable, and comply with ethical standards. A supplier’s reputation may influence the customer’s reputation. Therefore, selecting a supplier with an enviable industry reputation is critical. Moreover, a supplier’s reputation may impact its ability to win agreements and collaboration, threatening a company’s viability over time. As a result, when choosing which supplier to work with, it is important to look at the company’s reputation. The following criteria are often used when evaluating supplier reputation:
• Financial stability: It is necessary to assess suppliers’ financial stability to guarantee that they can fulfil orders and deliver consistent quality throughout time.
• Industry experience: The knowledge and competence of a provider in their area can impact the quality of their products and services.
• Customer satisfaction: A track record of satisfied customers might reflect a supplier’s dependability and quality.
• Innovation: Suppliers who spend in R&D and bring new and innovative products and services to market may have an advantage over competitors.
• Ethical and social responsibility: The supplier’s principles, labour methods, and dedication toward environmental stability are important factors that might impact their reputation.
• Quality management system: The devotion of a supplier to quality assurance and continuous advancement can indicate the dependability and standards of their goods or services.
• Delivery performance: On-time delivery and robust supply chain management are important aspects of supplier selection since both can significantly affect a business’s performance.
Suppose
Step-1: Obtain Pythagorean fuzzy soft decision matrices Tables 1–4.
Step-2: Employing the normalization process in Tables 5–8, modify the decision matrix by normalizing it and transforming the assessments of cost-type parameters into evaluations of benefit-type parameters. Because
Step-3: By utilizing the proposed IVPFSIWA operator on the gathered data, we can extract the viewpoints of the decision-makers,
Similarly,
Step-4: To determine the score values for all alternatives, we can employ the score function
Step-5: From the above calculation, we get
Step-1: Attain IVPFS decision matrices (Tables 1–4).
Step-2: Transforming the decision matrix by normalizing it and transforming the evaluations of cost-type parameters into evaluations of benefit-type parameters through the normalization equation given in Tables 5–8. Because
Step-3: By utilizing the proposed IVPFSIWG operator on the gathered data, we can extract the viewpoints of the decision-makers,
Similarly,
Step-4: To calculate the score values for all alternatives, we can employ the score function
Step-5: The calculation results in the ranking of alternatives
In the following part, the practicality of the proposed strategy will be evaluated by comparing it to existing methods.
5.1 Supremacy of the Planned Technique
This paper presents a highly efficient and feasible approach to managing MCGDM complications in the IVPFSS setting. We propose an innovative MCGDM model incorporating the IVPFSIWA and IVPFSIWG operators that is more advanced and capable of dealing with the complexities of MCGDM than conventional approaches. Our model is flexible, adaptable, and can handle changing conditions while meeting desired outputs. Different models’ ranking process varies, affecting the rankings of the proposed method compared to their expectations. Our systematic study results demonstrate that current procedures’ outcomes are inconsistent compared to hybrid structures. Among hybrid IVFS, IVIFS, IVPFS, and IVIFSS, IVPFSS is a popular choice due to its ability to handle ambiguous and uncertain data in DM procedures. Our proposed method is more efficient and effective than other mixed FS structures, as it can better deal with ambiguous and uncertain data in the DM process. Moreover, the details of our proposed technique and limitations of the prevailing aggregation operators and our proposed operators are in Table 9.
It appears that a novel challenge has recently arisen. This paper will demonstrate the justification behind using a novel MCGDM method established to fulfil the specific requirements of a specific business. Although the reality there are various existing strategies, the suggested method differentiates because of its distinctive hybrids structures, which include FS, IVFS, IFS, IVIFS, PFS, IVPFS, FSS, IVFSS, IFSS, IVIFSS, and PFSS. Such mixed structures still have constraints in thoroughly determining the circumstance. To address these drawbacks, we proposed an MCGDM technique based on interaction AOs for IVPFSS, which can accommodate parameters with MD and NMD in intervals which include
The manuscript compares the proposed technique to existing methods in the interval-valued Pythagorean fuzzy sets (IVPFS), interval-valued intuitionistic fuzzy soft sets (IVIFSS), and interval-valued Pythagorean fuzzy soft sets (IVPFSS) settings, as shown in Table 10. The current IVIFWA and IVIFWG operators [47,48] and the TOPSIS model [24] do not consider the parametrized values of alternatives. The IVIFSWA and IVIFSWG operators [33] account for the decision-makers preferences but face limitations when the sum of the upper values of MD and NMD exceeds one. Similarly, the IVPFWA [23] operator and IVPFWG [25] operator also struggle to handle the parametrized values of alternatives. Zulqarnain et al. [41] introduced the AOs for IVPFSS to address these issues. However, when the upper values of MD and NMD add up to one or less, the IVPFSS reduces to IVIFSS, making it a more generalized form of interval-valued Pythagorean fuzzy sets. The proposed operators in this study are shown to be more efficient, reliable, and successful than the existing methods, as evidenced by the comparison presented in Table 10.
As a result, we have numerous reasons to be astounded by the illegal use and inefficiency of the DM methodology of the predominant operators we noticed. Planned encouragement for this method-related activity has a minor influence on adverse reasons. It reduces the structure of unreliable and anticipated facts in the DM intensification in such a manner. Fig. 1 displays a visual illustration of the comparative evaluation.
Figure 1: Comparative analysis of the proposed approach with existing models
5.3 Merits and Advantages of the Planned Model
The model that has been suggested has multiple features that improve its dependability and performance, such as
• The professionals frequently address obstacles while determining features and classifying them according to several attributes since these decisions are rarely exact. The suggested approach examines this problem using the idea of interval-valued Pythagorean fuzzy parameterization that reflects attribute volatility.
• The established approach is capable of comparable values of attributes in estimates, delivering clarification to areas with uncertainties. This helps by delivering 2D MD and NMD functions in interval form and implementing stage variables to deal with repetition.
• The MCGDM approach allows professionals to perform judgments relying upon multiple factors, proving more productive than relying on just one parameter. This aspect becomes particularly beneficial in the context of IVPFSS.
• This research presents mathematical justifications for the AOs used in the suggested method, confirming the approach’s validity. This consistency ensures impartiality and consistency in decision-making processes, and the methodology is suitable for various applications requesting precision and reliability.
• The research’s concentration on IVPFSS indicates a significant hypothetical incursion, as it is the most integrated IVPFSS method. The proliferation of interactional operational laws for IVPFSS scenarios and the case regarding their basic possessions are auxiliary propagated the uncertain build for IVPFSS, which contributed to more unified and reliable DM approaches in the environment of IVPFSS, which contributed to intellectual growth in FS and DM.
Decision-makers often face challenges when assessing alternatives due to the strict requirements of DM exploration. To overcome these limitations, the IVPFSS mathematical structure can communicate unreliable and indefinite data in real-life situations. The DM system is also robust and can help classify the most helpful alternative while considering the existing approach to isolate statistical decision-makers. This study presents a new method for sustainable supplier selection using the IVPFSS setting. The proposed method considers the complexity of real-life situations and addresses the limitations of previous methods that did not consider interactions and complicated circumstances among attributes. We introduce interactional operational laws for IVPFSS and develop IVPFSIWA and IVPFSIWG operators with desired properties. Based on these operators, we propose a decision-making method to address complications in MCGDM. To demonstrate the robustness of the proposed methodology, we provide a detailed mathematical illustration for sustainable supplier selection and compare it with existing methods, showing that it is the most practical and effective solution for MCGDM. Future studies will concentrate on constructing Einstein-ordered aggregation operators (AOs) and designing distance and similarity measures with distinctive features. The technique may also be modified to incorporate interval-valued q-ROFSS, integrating significant operations and the decision-making procedures that go with them. Moreover, combining IVPFSNs with other MCGDM approaches has the potential for practical applications in various fields, including medical diagnostics, material selection, pattern recognition, information fusion, and supply chain management. Also, IVPFSNs can be investigated by employing multiple topological, algebraic, and ordered structures and the corresponding decision-making strategies.
Acknowledgement: Researchers Supporting Project Number (RSP2024R167), King Saud University, Riyadh, Saudi Arabia.
Funding Statement: This project is funded by King Saud University, Riyadh, Saudi Arabia.
Author Contributions: The authors confirm contribution to the paper as follows: study conception and design: R.M.Z, W.X.M, H.A; data collection: I.S; analysis and interpretation of results: I.S, H.A, S.A; draft manuscript preparation: R.M.Z, S.A. All authors reviewed the results and approved the final version of the manuscript.
Availability of Data Materials: All the data used and analyzed is available in the manuscript.
Conflicts of Interest: The authors declare that they have no conflicts of interest to report regarding the present study.
References
1. Moghaddas, Z., Tosarkani, B. M., Yousefi, S. (2022). Resource reallocation for improving sustainable supply chain performance: An inverse data envelopment analysis. International Journal of Production Economics, 252, 108560. [Google Scholar]
2. Hosseini, Z. S., Flapper, S. D., Pirayesh, M. (2022). Sustainable supplier selection and order allocation under demand, supplier availability and supplier grading uncertainties. Computers & Industrial Engineering, 165, 107811. [Google Scholar]
3. Prajapati, D., Pratap, S., Zhang, M., Huang, G. Q. (2022). Sustainable forward-reverse logistics for multi-product delivery and pickup in B2C E-commerce towards the circular economy. International Journal of Production Economics, 253, 108606. [Google Scholar]
4. Banaeian, N., Mobli, H., Fahimnia, B., Nielsen, I. E., Omid, M. (2018). Green supplier selection using fuzzy group decision making methods: A case study from the agri-food industry. Computers & Operations Research, 89, 337–347. [Google Scholar]
5. Zadeh, L. A. (1965). Fuzzy sets. Information and Control, 8, 338–353. [Google Scholar]
6. Turksen, I. B. (1986). Interval valued fuzzy sets based on normal forms. Fuzzy Sets and Systems, 20(2), 191–210. [Google Scholar]
7. Atanassov, K. (1986). Intuitionistic fuzzy sets. Fuzzy Sets and Systems, 20, 87–96. [Google Scholar]
8. Wang, W., Liu, X. (2011). Intuitionistic fuzzy geometric aggregation operators based on Einstein operations. International Journal of Intelligent Systems, 26(11), 1049–1075. [Google Scholar]
9. Garg, H., Kaur, G. (2019). Cubic intuitionistic fuzzy sets and its fundamental properties. Journal of Multiple-Valued Logic & Soft Computing, 33(6), 507–537. [Google Scholar]
10. Atanassov, K. T. (1999). Interval-valued intuitionistic fuzzy sets. In: Intuitionistic fuzzy sets, pp. 139–177. Heidelberg: Physica. [Google Scholar]
11. Torağay, O., Arikan, M. (2015). Performance evaluation of faculty departments by a Delphi method based on 2-Tuple fuzzy Linguistic representation model and TOPSIS. International Journal of Basic and Applied Sciences, 15(5), 11. [Google Scholar]
12. Yager, R. R. (2013). Pythagorean membership grades in multi-criteria decision making. IEEE Transactions on Fuzzy Systems, 22(4), 958–965. [Google Scholar]
13. Rahman, K., Abdullah, S., Ahmed, R., Ullah, M. (2017). Pythagorean fuzzy Einstein weighted geometric aggregation operator and their application to multiple attribute group decision making. Journal of Intelligent & Fuzzy Systems, 33(1), 635–647. [Google Scholar]
14. Giri, B. C., Molla, M. U., Biswas, P. (2022). Pythagorean fuzzy DEMATEL method for supplier selection in sustainable supply chain management. Expert Systems with Applications, 193, 116396. [Google Scholar]
15. Zhang, X., Xu, Z. (2014). Extension of TOPSIS to multiple criteria decision making with Pythagorean fuzzy sets. International Journal of Intelligent Systems, 29(12), 1061–1078. [Google Scholar]
16. Wei, G., Lu, M. (2018). Pythagorean fuzzy power aggregation operators in multiple attribute decision making. International Journal of Intelligent Systems, 33(1), 169–186. [Google Scholar]
17. Wang, L., Li, N. (2020). Pythagorean fuzzy interaction power Bonferroni means aggregation operators in multiple attribute decision making. International Journal of Intelligent Systems, 35(1), 150–183. [Google Scholar]
18. Chaurasiya, R., Jain, D. (2023). Hybrid MCDM method on Pythagorean fuzzy set and its application. Decision Making: Applications in Management and Engineering, 6(1), 379–398. [Google Scholar]
19. El-Morsy, S. (2023). Stock portfolio optimization using Pythagorean fuzzy numbers. Journal of Operational and Strategic Analytics, 1(1), 8–13. [Google Scholar]
20. Zhang, X. (2016). A novel approach based on similarity measure for Pythagorean fuzzy multiple criteria group decision making. International Journal of Intelligent Systems, 31(6), 593–611. [Google Scholar]
21. Anusha, G., Ramana, P. V., Sarkar, R. (2023). Hybridizations of Archimedean copula and generalized MSM operators and their applications in interactive decision-making with q-rung probabilistic dual hesitant fuzzy environment. Decision Making: Applications in Management and Engineering, 6(1), 646–678. [Google Scholar]
22. Khan, M. R., Ullah, K., Khan, Q. (2023). Multi-attribute decision-making using Archimedean aggregation operator in T-spherical fuzzy environment. Reports in Mechanical Engineering, 4(1), 18–38. [Google Scholar]
23. Peng, X., Yang, Y. (2016). Fundamental properties of interval-valued Pythagorean fuzzy aggregation operators. International Journal of Intelligent Systems, 31(5), 444–487. [Google Scholar]
24. Yu, C., Shao, Y., Wang, K., Zhang, L. (2019). A group decision making sustainable supplier selection approach using extended TOPSIS under interval-valued Pythagorean fuzzy environment. Expert Systems with Applications, 121, 1–17. [Google Scholar]
25. Rahman, K., Abdullah, S., Shakeel, M., Ali Khan, M. S., Ullah, M. (2017). Interval-valued Pythagorean fuzzy geometric aggregation operators and their application to group decision making problem. Cogent Mathematics, 4(1), 1338638. [Google Scholar]
26. Mu, Z., Zeng, S., Wang, P. (2021). Novel approach to multi-attribute group decision-making based on interval-valued Pythagorean fuzzy power Maclaurin symmetric mean operator. Computers & Industrial Engineering, 155, 107049. [Google Scholar]
27. Molodtsov, D. (1999). Soft set theory—First results. Computers & Mathematics with Applications, 37(4–5), 19–31. [Google Scholar]
28. Fu, W., Khalil, A. M., Zahran, A. M., Basheer, R. (2021). Possibility belief interval-valued soft set and its application in decision making. Journal of Intelligent & Fuzzy Systems, 41(1), 231–249. [Google Scholar]
29. Khalil, A. M., Li, S. G., Lin, Y., Li, H. X., Ma, S. G. (2020). A new expert system in prediction of lung cancer disease based on fuzzy soft sets. Soft Computing, 24(18), 14179–14207. [Google Scholar]
30. Maji, P. K., Biswas, R., Roy, A. R. (2001). Intuitionistic fuzzy soft sets. Journal of Fuzzy Mathematics, 9, 677–692. [Google Scholar]
31. Arora, R., Garg, H. (2018). A robust aggregation operators for multi-criteria decision-making with intuitionistic fuzzy soft set environment. Scientia Iranica, 25(2), 931–942. [Google Scholar]
32. Jiang, Y., Tang, Y., Chen, Q., Liu, H., Tang, J. (2010). Interval-valued intuitionistic fuzzy soft sets and their properties. Computers & Mathematics with Applications, 60(3), 906–918. [Google Scholar]
33. Zulqarnain, R. M., Xin, X. L., Saqlain, M., Khan, W. A. (2021). TOPSIS method based on the correlation coefficient of interval-valued intuitionistic fuzzy soft sets and aggregation operators with their application in decision-making. Journal of Mathematics, 2021, 1–16. https://doi.org/10.1155/2021/6656858 [Google Scholar] [CrossRef]
34. Peng, X. D., Yang, Y., Song, J., Jiang, Y. (2015). Pythagorean fuzzy soft set and its application. Computer Engineering, 41(7), 224–229. [Google Scholar]
35. Zulqarnain, R. M., Xin, X. L., Garg, H., Khan, W. A. (2021). Aggregation operators of pythagorean fuzzy soft sets with their application for green supplier chain management. Journal of Intelligent and Fuzzy Systems, 40(3), 5545–5563. https://doi.org/10.3233/JIFS-202781 [Google Scholar] [CrossRef]
36. Zulqarnain, R. M., Xin, X. L., Garg, H., Ali, R. (2021). Interaction aggregation operators to solve multi criteria decision making problem under pythagorean fuzzy soft environment. Journal of Intelligent & Fuzzy Systems, 41(1), 1151–1171. [Google Scholar]
37. Kirişci, M., Şimşek, N. (2022). Decision making method related to Pythagorean Fuzzy soft sets with infectious diseases application. Journal of King Saud University-Computer and Information Sciences, 34(8), 5968–5978. [Google Scholar]
38. Zulqarnain, R. M., Siddique, I., Ahmad, S., Iampan, A., Jovanov, G. et al. (2021). Pythagorean fuzzy soft Einstein ordered weighted average operator in sustainable supplier selection problem. Mathematical Problems in Engineering, 2021(4), 1–16. https://doi.org/10.1155/2021/2559979 [Google Scholar] [CrossRef]
39. Zulqarnain, R. M., Siddique, I., EI-Morsy, S. (2022). Einstein-ordered weighted geometric operator for pythagorean fuzzy soft set with its application to solve MAGDM problem. Mathematical Problems in Engineering, 2022(4), 1–14. https://doi.org/10.1155/2022/5199427 [Google Scholar] [CrossRef]
40. Siddique, I., Zulqarnain, R. M., Ali, R., Alburaikan, A., Iampan, A. et al. (2021). A decision-making approach based on score matrix for pythagorean fuzzy soft set. Computational Intelligence and Neuroscience, 2021(3), 1–16. https://doi.org/10.1155/2021/5447422 [Google Scholar] [PubMed] [CrossRef]
41. Zulqarnain, R. M., Siddique, I., Iampan, A., Baleanu, D. (2022). Aggregation operators for interval-valued Pythagorean fuzzy soft set with their application to solve multi-attribute group decision making problem. Computer Modeling in Engineering & Sciences, 131(3), 1717–1750. https://doi.org/10.32604/cmes.2022.019408 [Google Scholar] [CrossRef]
42. Qiu, P., Sorourkhah, A., Kausar, N., Cagin, T., Edalatpanah, S. A. (2023). Simplifying the complexity in the problem of choosing the best private-sector partner. Systems, 11(2), 80. [Google Scholar]
43. Chatterjee, K., Pamucar, D., Zavadskas, E. K. (2018). Evaluating the performance of suppliers based on using the R’AMATELMAIRCA method for green supply chain implementation in electronics industry. Journal of Cleaner Production, 184, 101–129. [Google Scholar]
44. Stevic, Z., Pamucar, D., Vasiljevic, M., Stojic, G., Korica, S. (2017). Novel integrated multicriteria model for supplier selection: Case study construction company. Symmetry, 9(11), 279. [Google Scholar]
45. Stojic, G., Stevic, Z., Antucheviciene, J., Pamucar, D., Vasiljevic, M. (2018). A novel rough WASPAS approach for supplier selection in a company manufacturing PVC carpentry products. Information, 9(5), 121. [Google Scholar]
46. Stevic, Z., Pamucar, D., Puska, A., Chatterjee, P. (2020). Sustainable supplier selection in healthcare industries using a new MCDM method: Measurement of alternatives and ranking according to COmpromise solution (MARCOS). Computers Industrial Engineering, 140, 106231. [Google Scholar]
47. Wang, W., Liu, X., Qin, Y. (2012). Interval-valued intuitionistic fuzzy aggregation operators. Journal of Systems Engineering and Electronics, 23(4), 574–580. [Google Scholar]
48. Xu, Z., Chen, J. (2007). On geometric aggregation over interval-valued intuitionistic fuzzy information. The Fourth International Conference on Fuzzy Systems and Knowledge Discovery (FSKD 2007), pp. 466–471. Haikou, China. [Google Scholar]
Cite This Article
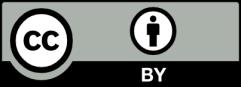