Open Access
REVIEW
AI Fairness–From Machine Learning to Federated Learning
1 Consciousness Studies Program, School of Humanities, National Institute of Advanced Studies, Bangalore, 560012, India
2 Research Institute of Intelligent Engineering and Data Applications, Shanghai Institute of Technology, Shanghai, 201418, China
3 Research Center of Ecology and Environment of Central Asia, Chinese Academy of Sciences, Urumqi, 830011, China
4 Applied Nonlinear Science Lab, Anand International College of Engineering, Jaipur, 391320, India
5 London Institute of Technology, The ASE-London CTI of SCO, London, CR26EQ, UK
6 Sino-Indian Joint Research Center of AI and Robotics, The IMT Institute, Bhubaneswar, 752054, India
* Corresponding Author: Wenfeng Wang. Email:
(This article belongs to the Special Issue: Federated Learning Algorithms, Approaches, and Systems for Internet of Things)
Computer Modeling in Engineering & Sciences 2024, 139(2), 1203-1215. https://doi.org/10.32604/cmes.2023.029451
Received 20 February 2023; Accepted 15 November 2023; Issue published 29 January 2024
Abstract
This article reviews the theory of fairness in AI–from machine learning to federated learning, where the constraints on precision AI fairness and perspective solutions are also discussed. For a reliable and quantitative evaluation of AI fairness, many associated concepts have been proposed, formulated and classified. However, the inexplicability of machine learning systems makes it almost impossible to include all necessary details in the modelling stage to ensure fairness. The privacy worries induce the data unfairness and hence, the biases in the datasets for evaluating AI fairness are unavoidable. The imbalance between algorithms’ utility and humanization has further reinforced such worries. Even for federated learning systems, these constraints on precision AI fairness still exist. A perspective solution is to reconcile the federated learning processes and reduce biases and imbalances accordingly.Keywords
Cite This Article
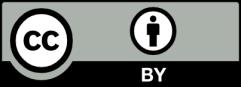
This work is licensed under a Creative Commons Attribution 4.0 International License , which permits unrestricted use, distribution, and reproduction in any medium, provided the original work is properly cited.