Open Access
ARTICLE
Stroke Risk Assessment Decision-Making Using a Machine Learning Model: Logistic-AdaBoost
1 School of Science, Wuhan University of Technology, Wuhan, 430070, China
2 Wuhan University of Technology Hospital, Wuhan University of Technology, Wuhan, 430070, China
* Corresponding Author: Tingting Huang. Email:
(This article belongs to the Special Issue: Data-Driven Robust Group Decision-Making Optimization and Application)
Computer Modeling in Engineering & Sciences 2024, 139(1), 699-724. https://doi.org/10.32604/cmes.2023.044898
Received 11 August 2023; Accepted 28 September 2023; Issue published 30 December 2023
Abstract
Stroke is a chronic cerebrovascular disease that carries a high risk. Stroke risk assessment is of great significance in preventing, reversing and reducing the spread and the health hazards caused by stroke. Aiming to objectively predict and identify strokes, this paper proposes a new stroke risk assessment decision-making model named Logistic-AdaBoost (Logistic-AB) based on machine learning. First, the categorical boosting (CatBoost) method is used to perform feature selection for all features of stroke, and 8 main features are selected to form a new index evaluation system to predict the risk of stroke. Second, the borderline synthetic minority oversampling technique (SMOTE) algorithm is applied to transform the unbalanced stroke dataset into a balanced dataset. Finally, the stroke risk assessment decision-making model Logistic-AB is constructed, and the overall prediction performance of this new model is evaluated by comparing it with ten other similar models. The comparison results show that the new model proposed in this paper performs better than the two single algorithms (logistic regression and AdaBoost) on the four indicators of recall, precision, F1 score, and accuracy, and the overall performance of the proposed model is better than that of common machine learning algorithms. The Logistic-AB model presented in this paper can more accurately predict patients’ stroke risk.Keywords
Cite This Article
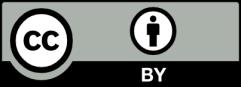
This work is licensed under a Creative Commons Attribution 4.0 International License , which permits unrestricted use, distribution, and reproduction in any medium, provided the original work is properly cited.