Open Access
ARTICLE
An End-To-End Hyperbolic Deep Graph Convolutional Neural Network Framework
1 School of Information Network Security, People’s Public Security University of China, Beijing, 100038, China
2 Institute of Computing Technology, China Academy of Railway Sciences Corporation Limited, Beijing, 100081, China
* Corresponding Author: Fanliang Bu. Email:
Computer Modeling in Engineering & Sciences 2024, 139(1), 537-563. https://doi.org/10.32604/cmes.2023.044895
Received 11 August 2023; Accepted 31 October 2023; Issue published 30 December 2023
Abstract
Graph Convolutional Neural Networks (GCNs) have been widely used in various fields due to their powerful capabilities in processing graph-structured data. However, GCNs encounter significant challenges when applied to scale-free graphs with power-law distributions, resulting in substantial distortions. Moreover, most of the existing GCN models are shallow structures, which restricts their ability to capture dependencies among distant nodes and more refined high-order node features in scale-free graphs with hierarchical structures. To more broadly and precisely apply GCNs to real-world graphs exhibiting scale-free or hierarchical structures and utilize multi-level aggregation of GCNs for capturing high-level information in local representations, we propose the Hyperbolic Deep Graph Convolutional Neural Network (HDGCNN), an end-to-end deep graph representation learning framework that can map scale-free graphs from Euclidean space to hyperbolic space. In HDGCNN, we define the fundamental operations of deep graph convolutional neural networks in hyperbolic space. Additionally, we introduce a hyperbolic feature transformation method based on identity mapping and a dense connection scheme based on a novel non-local message passing framework. In addition, we present a neighborhood aggregation method that combines initial structural features with hyperbolic attention coefficients. Through the above methods, HDGCNN effectively leverages both the structural features and node features of graph data, enabling enhanced exploration of non-local structural features and more refined node features in scale-free or hierarchical graphs. Experimental results demonstrate that HDGCNN achieves remarkable performance improvements over state-of-the-art GCNs in node classification and link prediction tasks, even when utilizing low-dimensional embedding representations. Furthermore, when compared to shallow hyperbolic graph convolutional neural network models, HDGCNN exhibits notable advantages and performance enhancements.Keywords
Cite This Article
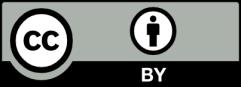
This work is licensed under a Creative Commons Attribution 4.0 International License , which permits unrestricted use, distribution, and reproduction in any medium, provided the original work is properly cited.