Open Access
ARTICLE
User Purchase Intention Prediction Based on Improved Deep Forest
1 School of Computer Science and Engineering, North Minzu University, Yinchuan, 750030, China
2 Laboratory of Graphics and Images of the State Ethnic Affairs Commission, North Minzu University, Yinchuan, 750030, China
* Corresponding Author: Qiancheng Yu. Email:
(This article belongs to the Special Issue: Machine Learning-Guided Intelligent Modeling with Its Industrial Applications)
Computer Modeling in Engineering & Sciences 2024, 139(1), 661-677. https://doi.org/10.32604/cmes.2023.044255
Received 25 July 2023; Accepted 18 September 2023; Issue published 30 December 2023
Abstract
Widely used deep neural networks currently face limitations in achieving optimal performance for purchase intention prediction due to constraints on data volume and hyperparameter selection. To address this issue, based on the deep forest algorithm and further integrating evolutionary ensemble learning methods, this paper proposes a novel Deep Adaptive Evolutionary Ensemble (DAEE) model. This model introduces model diversity into the cascade layer, allowing it to adaptively adjust its structure to accommodate complex and evolving purchasing behavior patterns. Moreover, this paper optimizes the methods of obtaining feature vectors, enhancement vectors, and prediction results within the deep forest algorithm to enhance the model's predictive accuracy. Results demonstrate that the improved deep forest model not only possesses higher robustness but also shows an increase of 5.02% in AUC value compared to the baseline model. Furthermore, its training runtime speed is 6 times faster than that of deep models, and compared to other improved models, its accuracy has been enhanced by 0.9%.Keywords
Cite This Article
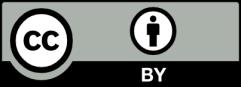