Open Access
ARTICLE
Enhancing Healthcare Data Security and Disease Detection Using Crossover-Based Multilayer Perceptron in Smart Healthcare Systems
Department of Industrial Engineering, College of Engineering, King Saud University, P.O. Box-800, Riyadh, 11421, Saudi Arabia
* Corresponding Author: Mustufa Haider Abidi. Email:
(This article belongs to the Special Issue: Smart and Secure Solutions for Medical Industry)
Computer Modeling in Engineering & Sciences 2024, 139(1), 977-997. https://doi.org/10.32604/cmes.2023.044169
Received 23 July 2023; Accepted 27 October 2023; Issue published 30 December 2023
Abstract
The healthcare data requires accurate disease detection analysis, real-time monitoring, and advancements to ensure proper treatment for patients. Consequently, Machine Learning methods are widely utilized in Smart Healthcare Systems (SHS) to extract valuable features from heterogeneous and high-dimensional healthcare data for predicting various diseases and monitoring patient activities. These methods are employed across different domains that are susceptible to adversarial attacks, necessitating careful consideration. Hence, this paper proposes a crossover-based Multilayer Perceptron (CMLP) model. The collected samples are pre-processed and fed into the crossover-based multilayer perceptron neural network to detect adversarial attacks on the medical records of patients. Once an attack is detected, healthcare professionals are promptly alerted to prevent data leakage. The paper utilizes two datasets, namely the synthetic dataset and the University of Queensland Vital Signs (UQVS) dataset, from which numerous samples are collected. Experimental results are conducted to evaluate the performance of the proposed CMLP model, utilizing various performance measures such as Recall, Precision, Accuracy, and F1-score to predict patient activities. Comparing the proposed method with existing approaches, it achieves the highest accuracy, precision, recall, and F1-score. Specifically, the proposed method achieves a precision of 93%, an accuracy of 97%, an F1-score of 92%, and a recall of 92%.Keywords
Cite This Article
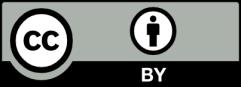
This work is licensed under a Creative Commons Attribution 4.0 International License , which permits unrestricted use, distribution, and reproduction in any medium, provided the original work is properly cited.