Open Access
ARTICLE
A Railway Fastener Inspection Method Based on Abnormal Sample Generation
1 Higher Vocational and Technical College, Shanghai University of Engineering Science, Shanghai, 200437, China
2 School of Urban Railway Transportation, Shanghai University of Engineering Science, Shanghai, 201620, China
3 Shanghai Engineering Research Centre of Vibration and Noise Control Technologies for Rail Transit, Shanghai University of Engineering Science, Shanghai, 201620, China
* Corresponding Author: Liming Li. Email:
(This article belongs to the Special Issue: Failure Detection Algorithms, Methods and Models for Industrial Environments)
Computer Modeling in Engineering & Sciences 2024, 139(1), 565-592. https://doi.org/10.32604/cmes.2023.043832
Received 13 August 2023; Accepted 24 October 2023; Issue published 30 December 2023
Abstract
Regular fastener detection is necessary to ensure the safety of railways. However, the number of abnormal fasteners is significantly lower than the number of normal fasteners in real railways. Existing supervised inspection methods have insufficient detection ability in cases of imbalanced samples. To solve this problem, we propose an approach based on deep convolutional neural networks (DCNNs), which consists of three stages: fastener localization, abnormal fastener sample generation based on saliency detection, and fastener state inspection. First, a lightweight YOLOv5s is designed to achieve fast and precise localization of fastener regions. Then, the foreground clip region of a fastener image is extracted by the designed fastener saliency detection network (F-SDNet), combined with data augmentation to generate a large number of abnormal fastener samples and balance the number of abnormal and normal samples. Finally, a fastener inspection model called Fastener ResNet-8 is constructed by being trained with the augmented fastener dataset. Results show the effectiveness of our proposed method in solving the problem of sample imbalance in fastener detection. Qualitative and quantitative comparisons show that the proposed F-SDNet outperforms other state-of-the-art methods in clip region extraction, reaching MAE and max F-measure of 0.0215 and 0.9635, respectively. In addition, the FPS of the fastener state inspection model reached 86.2, and the average accuracy reached 98.7% on 614 augmented fastener test sets and 99.9% on 7505 real fastener datasets.Keywords
Cite This Article
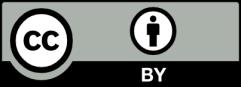
This work is licensed under a Creative Commons Attribution 4.0 International License , which permits unrestricted use, distribution, and reproduction in any medium, provided the original work is properly cited.