Open Access
ARTICLE
Toward Improved Accuracy in Quasi-Static Elastography Using Deep Learning
1 Department of Engineering Mechanics, State Key Laboratory of Structural Analysis for Industrial Equipment, Dalian University of Technology, Dalian, 116023, China
2 International Research Center for Computational Mechanics, Dalian University of Technology, Dalian, 116023, China
3 DUT-BSU Joint Institute, Dalian University of Technology, Dalian, 116023, China
4 Department of Automotive Engineering, Tongji University, Shanghai, 201804, China
5 Amazon.com, Seattle, WA, 98109, USA
6 School of Automotive Engineering, Dalian University of Technology, Dalian, 116023, China
* Corresponding Author: Xuefeng Zhu. Email:
(This article belongs to the Special Issue: Machine Learning Based Computational Mechanics)
Computer Modeling in Engineering & Sciences 2024, 139(1), 911-935. https://doi.org/10.32604/cmes.2023.043810
Received 12 July 2023; Accepted 08 September 2023; Issue published 30 December 2023
Abstract
Elastography is a non-invasive medical imaging technique to map the spatial variation of elastic properties of soft tissues. The quality of reconstruction results in elastography is highly sensitive to the noise induced by imaging measurements and processing. To address this issue, we propose a deep learning (DL) model based on conditional Generative Adversarial Networks (cGANs) to improve the quality of nonhomogeneous shear modulus reconstruction. To train this model, we generated a synthetic displacement field with finite element simulation under known nonhomogeneous shear modulus distribution. Both the simulated and experimental displacement fields are used to validate the proposed method. The reconstructed results demonstrate that the DL model with synthetic training data is able to improve the quality of the reconstruction compared with the well-established optimization method. Moreover, we emphasize that our DL model is only trained on synthetic data. This might provide a way to alleviate the challenge of obtaining clinical or experimental data in elastography. Overall, this work addresses several fatal issues in applying the DL technique into elastography, and the proposed method has shown great potential in improving the accuracy of the disease diagnosis in clinical medicine.Keywords
Cite This Article
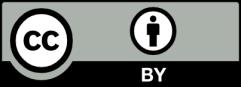