Open Access
ARTICLE
A Bitcoin Address Multi-Classification Mechanism Based on Bipartite Graph-Based Maximization Consensus
1 College of Information Engineering, Yangzhou University, Yangzhou, 225127, China
2 Cyberspace Institute Advanced Technology, Guangzhou University, Guangzhou, 510006, China
3 Research and Development Center for E-Learning, Ministry of Education, Beijing, 100039, China
4 Institute of High Performance Computing (IHPC), Agency for Science, Technology and Research (A*STAR), Singapore, 138632, Singapore
5 School of Physics and Materials Science, Guangzhou University, Guangzhou, 510006, China
* Corresponding Author: Lejun Zhang. Email:
(This article belongs to the Special Issue: The Bottleneck of Blockchain Techniques: Scalability, Security and Privacy Protection)
Computer Modeling in Engineering & Sciences 2024, 139(1), 783-800. https://doi.org/10.32604/cmes.2023.043469
Received 03 July 2023; Accepted 28 September 2023; Issue published 30 December 2023
Abstract
Bitcoin is widely used as the most classic electronic currency for various electronic services such as exchanges, gambling, marketplaces, and also scams such as high-yield investment projects. Identifying the services operated by a Bitcoin address can help determine the risk level of that address and build an alert model accordingly. Feature engineering can also be used to flesh out labeled addresses and to analyze the current state of Bitcoin in a small way. In this paper, we address the problem of identifying multiple classes of Bitcoin services, and for the poor classification of individual addresses that do not have significant features, we propose a Bitcoin address identification scheme based on joint multi-model prediction using the mapping relationship between addresses and entities. The innovation of the method is to (1) Extract as many valuable features as possible when an address is given to facilitate the multi-class service identification task. (2) Unlike the general supervised model approach, this paper proposes a joint prediction scheme for multiple learners based on address-entity mapping relationships. Specifically, after obtaining the overall features, the address classification and entity clustering tasks are performed separately, and the results are subjected to graph-based maximization consensus. The final result is made to baseline the individual address classification results while satisfying the constraint of having similarly behaving entities as far as possible. By testing and evaluating over 26,000 Bitcoin addresses, our feature extraction method captures more useful features. In addition, the combined multi-learner model obtained results that exceeded the baseline classifier reaching an accuracy of 77.4%.Keywords
Cite This Article
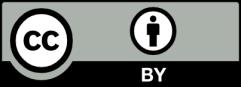
This work is licensed under a Creative Commons Attribution 4.0 International License , which permits unrestricted use, distribution, and reproduction in any medium, provided the original work is properly cited.