Open Access
ARTICLE
CALTM: A Context-Aware Long-Term Time-Series Forecasting Model
1 School of Computer and Computing Science, Hangzhou City University, Hangzhou, 310015, China
2 School of Computer Science and Engineering, Macau University of Science and Technology, Macau, 999078, China
3 College of Computer Science and Technology, Zhejiang University, Hangzhou, 310058, China
* Corresponding Author: Canghong Jin. Email:
(This article belongs to the Special Issue: Machine Learning Empowered Distributed Computing: Advance in Architecture, Theory and Practice)
Computer Modeling in Engineering & Sciences 2024, 139(1), 873-891. https://doi.org/10.32604/cmes.2023.043230
Received 26 June 2023; Accepted 27 September 2023; Issue published 30 December 2023
Abstract
Time series data plays a crucial role in intelligent transportation systems. Traffic flow forecasting represents a precise estimation of future traffic flow within a specific region and time interval. Existing approaches, including sequence periodic, regression, and deep learning models, have shown promising results in short-term series forecasting. However, forecasting scenarios specifically focused on holiday traffic flow present unique challenges, such as distinct traffic patterns during vacations and the increased demand for long-term forecastings. Consequently, the effectiveness of existing methods diminishes in such scenarios. Therefore, we propose a novel long-term forecasting model based on scene matching and embedding fusion representation to forecast long-term holiday traffic flow. Our model comprises three components: the similar scene matching module, responsible for extracting Similar Scene Features; the long-short term representation fusion module, which integrates scenario embeddings; and a simple fully connected layer at the head for making the final forecasting. Experimental results on real datasets demonstrate that our model outperforms other methods, particularly in medium and long-term forecasting scenarios.Keywords
Cite This Article
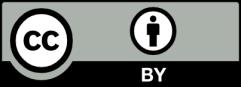
This work is licensed under a Creative Commons Attribution 4.0 International License , which permits unrestricted use, distribution, and reproduction in any medium, provided the original work is properly cited.