Open Access
ARTICLE
Improving Federated Learning through Abnormal Client Detection and Incentive
1 College of Computer and Information, Hohai University, Nanjing, 211100, China
2 Key Laboratory of Water Big Data Technology of Ministry of Water Resources, Hohai University, Nanjing, 211100, China
* Corresponding Author: Yingchi Mao. Email:
Computer Modeling in Engineering & Sciences 2024, 139(1), 383-403. https://doi.org/10.32604/cmes.2023.031466
Received 19 June 2023; Accepted 28 September 2023; Issue published 30 December 2023
Abstract
Data sharing and privacy protection are made possible by federated learning, which allows for continuous model parameter sharing between several clients and a central server. Multiple reliable and high-quality clients must participate in practical applications for the federated learning global model to be accurate, but because the clients are independent, the central server cannot fully control their behavior. The central server has no way of knowing the correctness of the model parameters provided by each client in this round, so clients may purposefully or unwittingly submit anomalous data, leading to abnormal behavior, such as becoming malicious attackers or defective clients. To reduce their negative consequences, it is crucial to quickly detect these abnormalities and incentivize them. In this paper, we propose a Federated Learning framework for Detecting and Incentivizing Abnormal Clients (FL-DIAC) to accomplish efficient and security federated learning. We build a detector that introduces an auto-encoder for anomaly detection and use it to perform anomaly identification and prevent the involvement of abnormal clients, in particular for the anomaly client detection problem. Among them, before the model parameters are input to the detector, we propose a Fourier transform-based anomaly data detection method for dimensionality reduction in order to reduce the computational complexity. Additionally, we create a credit score-based incentive structure to encourage clients to participate in training in order to make clients actively participate. Three training models (CNN, MLP, and ResNet-18) and three datasets (MNIST, Fashion MNIST, and CIFAR-10) have been used in experiments. According to theoretical analysis and experimental findings, the FL-DIAC is superior to other federated learning schemes of the same type in terms of effectiveness.Keywords
Cite This Article
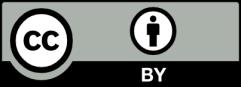
This work is licensed under a Creative Commons Attribution 4.0 International License , which permits unrestricted use, distribution, and reproduction in any medium, provided the original work is properly cited.