Open Access
ARTICLE
Block Incremental Dense Tucker Decomposition with Application to Spatial and Temporal Analysis of Air Quality Data
1 Department of Artificial Intelligence, Ajou University, Suwon-si, 16499, Korea
2 Department of Software and Computer Engineering, Ajou University, Suwon-si, 16499, Korea
* Corresponding Author: Lee Sael. Email:
Computer Modeling in Engineering & Sciences 2024, 139(1), 319-336. https://doi.org/10.32604/cmes.2023.031150
Received 17 May 2023; Accepted 22 September 2023; Issue published 30 December 2023
Abstract
How can we efficiently store and mine dynamically generated dense tensors for modeling the behavior of multidimensional dynamic data? Much of the multidimensional dynamic data in the real world is generated in the form of time-growing tensors. For example, air quality tensor data consists of multiple sensory values gathered from wide locations for a long time. Such data, accumulated over time, is redundant and consumes a lot of memory in its raw form. We need a way to efficiently store dynamically generated tensor data that increase over time and to model their behavior on demand between arbitrary time blocks. To this end, we propose a Block Incremental Dense Tucker Decomposition (BID-Tucker) method for efficient storage and on-demand modeling of multidimensional spatiotemporal data. Assuming that tensors come in unit blocks where only the time domain changes, our proposed BID-Tucker first slices the blocks into matrices and decomposes them via singular value decomposition (SVD). The SVDs of the sliced matrices are stored instead of the raw tensor blocks to save space. When modeling from data is required at particular time blocks, the SVDs of corresponding time blocks are retrieved and incremented to be used for Tucker decomposition. The factor matrices and core tensor of the decomposed results can then be used for further data analysis. We compared our proposed BID-Tucker with D-Tucker, which our method extends, and vanilla Tucker decomposition. We show that our BID-Tucker is faster than both D-Tucker and vanilla Tucker decomposition and uses less memory for storage with a comparable reconstruction error. We applied our proposed BID-Tucker to model the spatial and temporal trends of air quality data collected in South Korea from 2018 to 2022. We were able to model the spatial and temporal air quality trends. We were also able to verify unusual events, such as chronic ozone alerts and large fire events.Graphical Abstract
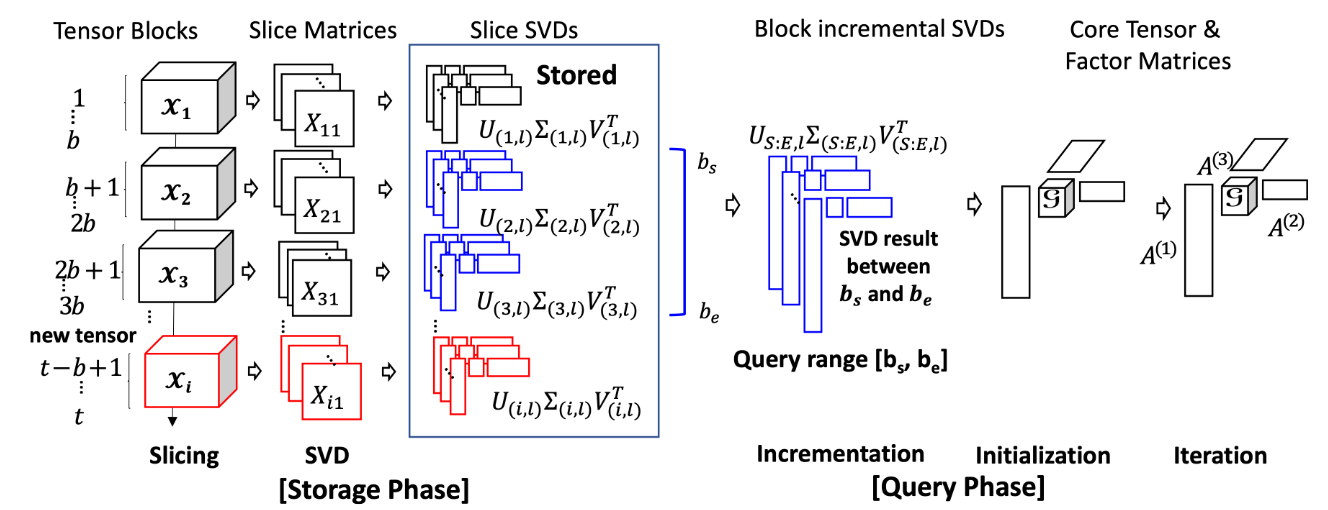
Keywords
Cite This Article
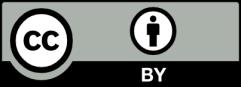