Open Access
ARTICLE
Deep Global Multiple-Scale and Local Patches Attention Dual-Branch Network for Pose-Invariant Facial Expression Recognition
1 School of Electrical and Information Engineering, Jiangsu University, Zhenjiang, 212013, China
2 School of Electrical Engineering, Yancheng Institute of Technology, Yancheng, 224051, China
* Corresponding Author: Xingqiao Liu. Email:
Computer Modeling in Engineering & Sciences 2024, 139(1), 405-440. https://doi.org/10.32604/cmes.2023.031040
Received 11 May 2023; Accepted 28 September 2023; Issue published 30 December 2023
Abstract
Pose-invariant facial expression recognition (FER) is an active but challenging research topic in computer vision. Especially with the involvement of diverse observation angles, FER makes the training parameter models inconsistent from one view to another. This study develops a deep global multiple-scale and local patches attention (GMS-LPA) dual-branch network for pose-invariant FER to weaken the influence of pose variation and self-occlusion on recognition accuracy. In this research, the designed GMS-LPA network contains four main parts, i.e., the feature extraction module, the global multiple-scale (GMS) module, the local patches attention (LPA) module, and the model-level fusion model. The feature extraction module is designed to extract and normalize texture information to the same size. The GMS model can extract deep global features with different receptive fields, releasing the sensitivity of deeper convolution layers to pose-variant and self-occlusion. The LPA module is built to force the network to focus on local salient features, which can lower the effect of pose variation and self-occlusion on recognition results. Subsequently, the extracted features are fused with a model-level strategy to improve recognition accuracy. Extensive experiments were conducted on four public databases, and the recognition results demonstrated the feasibility and validity of the proposed methods.Keywords
Cite This Article
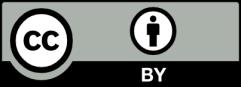
This work is licensed under a Creative Commons Attribution 4.0 International License , which permits unrestricted use, distribution, and reproduction in any medium, provided the original work is properly cited.