Open Access
ARTICLE
Fuzzy Difference Equations in Diagnoses of Glaucoma from Retinal Images Using Deep Learning
1 Department of Mathematics, Voorhees College, Vellore, Tamil Nadu, India
2 Chandigarh Group of Colleges, Jhanjeri, Mohali, Punjab, India
3 Department of Computer Engineering, College of Computer and Information Sciences, King Saud University, P.O. Box 51178, Riyadh, 11543, Saudi Arabia
4 Faculty of Science and Technology, School of IT and Systems, University of Canberra, Canberra, Australia
5 Operations Research Department, Faculty of Graduate Studies for Statistical Research, Cairo University, Giza, 12613, Egypt
6 Applied Science Research Center, Applied Science Private University, Amman, 11937, Jordan
* Corresponding Authors: Ali Wagdy Mohamed. Email: ,
# Lord Buddha Education Foundation, Kathmandu, Nepal (When the paper was submitted, Dr. Kautish was in LBEF Campus, Nepal. But currently he is in Chandigarh Group of Colleges, Jhanjeri, Mohali, Punjab, India.)
(This article belongs to the Special Issue: Advances in Ambient Intelligence and Social Computing under uncertainty and indeterminacy: From Theory to Applications)
Computer Modeling in Engineering & Sciences 2024, 139(1), 801-816. https://doi.org/10.32604/cmes.2023.030902
Received 01 May 2023; Accepted 21 September 2023; Issue published 30 December 2023
Abstract
The intuitive fuzzy set has found important application in decision-making and machine learning. To enrich and utilize the intuitive fuzzy set, this study designed and developed a deep neural network-based glaucoma eye detection using fuzzy difference equations in the domain where the retinal images converge. Retinal image detections are categorized as normal eye recognition, suspected glaucomatous eye recognition, and glaucomatous eye recognition. Fuzzy degrees associated with weighted values are calculated to determine the level of concentration between the fuzzy partition and the retinal images. The proposed model was used to diagnose glaucoma using retinal images and involved utilizing the Convolutional Neural Network (CNN) and deep learning to identify the fuzzy weighted regularization between images. This methodology was used to clarify the input images and make them adequate for the process of glaucoma detection. The objective of this study was to propose a novel approach to the early diagnosis of glaucoma using the Fuzzy Expert System (FES) and Fuzzy differential equation (FDE). The intensities of the different regions in the images and their respective peak levels were determined. Once the peak regions were identified, the recurrence relationships among those peaks were then measured. Image partitioning was done due to varying degrees of similar and dissimilar concentrations in the image. Similar and dissimilar concentration levels and spatial frequency generated a threshold image from the combined fuzzy matrix and FDE. This distinguished between a normal and abnormal eye condition, thus detecting patients with glaucomatous eyes.Keywords
Cite This Article
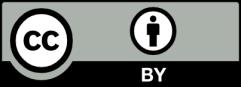
This work is licensed under a Creative Commons Attribution 4.0 International License , which permits unrestricted use, distribution, and reproduction in any medium, provided the original work is properly cited.