Open Access
REVIEW
Evolutionary Neural Architecture Search and Its Applications in Healthcare
1 School of Economics and Management, Hebei University of Technology, Tianjin, 300401, China
2 School of Artificial Intelligence, Hebei University of Technology, Tianjin, 300401, China
3 School of Electrical Engineering, Hebei University of Technology, Tianjin, 300401, China
4 Department of Game Design, Uppsala University, Uppsala, S-75105, Sweden
* Corresponding Authors: Jie Li. Email: ; Bin Cao. Email:
Computer Modeling in Engineering & Sciences 2024, 139(1), 143-185. https://doi.org/10.32604/cmes.2023.030391
Received 03 April 2023; Accepted 09 October 2023; Issue published 30 December 2023
Abstract
Most of the neural network architectures are based on human experience, which requires a long and tedious trial-and-error process. Neural architecture search (NAS) attempts to detect effective architectures without human intervention. Evolutionary algorithms (EAs) for NAS can find better solutions than human-designed architectures by exploring a large search space for possible architectures. Using multiobjective EAs for NAS, optimal neural architectures that meet various performance criteria can be explored and discovered efficiently. Furthermore, hardware-accelerated NAS methods can improve the efficiency of the NAS. While existing reviews have mainly focused on different strategies to complete NAS, a few studies have explored the use of EAs for NAS. In this paper, we summarize and explore the use of EAs for NAS, as well as large-scale multiobjective optimization strategies and hardware-accelerated NAS methods. NAS performs well in healthcare applications, such as medical image analysis, classification of disease diagnosis, and health monitoring. EAs for NAS can automate the search process and optimize multiple objectives simultaneously in a given healthcare task. Deep neural network has been successfully used in healthcare, but it lacks interpretability. Medical data is highly sensitive, and privacy leaks are frequently reported in the healthcare industry. To solve these problems, in healthcare, we propose an interpretable neuroevolution framework based on federated learning to address search efficiency and privacy protection. Moreover, we also point out future research directions for evolutionary NAS. Overall, for researchers who want to use EAs to optimize NNs in healthcare, we analyze the advantages and disadvantages of doing so to provide detailed guidance, and propose an interpretable privacy-preserving framework for healthcare applications.Graphic Abstract
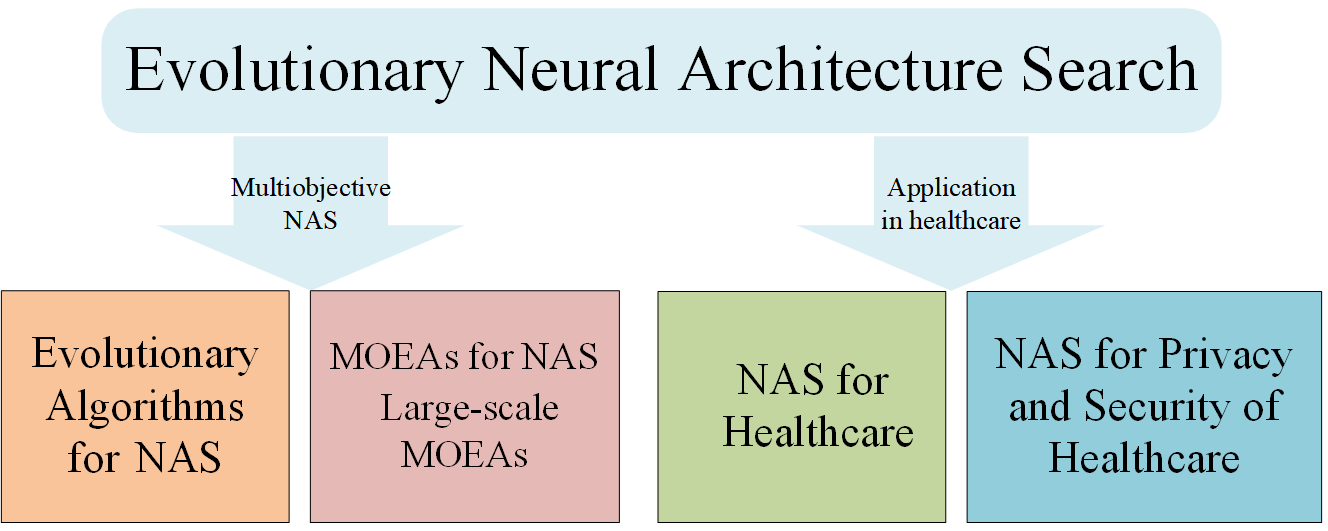
Keywords
Cite This Article
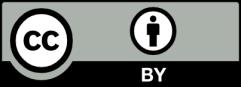
This work is licensed under a Creative Commons Attribution 4.0 International License , which permits unrestricted use, distribution, and reproduction in any medium, provided the original work is properly cited.