Open Access
ARTICLE
A Novel Predictive Model for Edge Computing Resource Scheduling Based on Deep Neural Network
1 School of Artificial Intelligence and Computer Science, Jiangnan University, Wuxi, 214122, China
2 The Center for Intelligent Systems and Applications Research, School of Artificial Intelligence and Computer Science, Jiangnan University, Wuxi, 214122, China
3 School of Information Engineering, Huzhou University, Huzhou, 313000, China
* Corresponding Author: Pengjiang Qian. Email:
# Ming Gao and Weiwei Cai contributed equally to this work
Computer Modeling in Engineering & Sciences 2024, 139(1), 259-277. https://doi.org/10.32604/cmes.2023.029015
Received 25 January 2023; Accepted 06 September 2023; Issue published 30 December 2023
Abstract
Currently, applications accessing remote computing resources through cloud data centers is the main mode of operation, but this mode of operation greatly increases communication latency and reduces overall quality of service (QoS) and quality of experience (QoE). Edge computing technology extends cloud service functionality to the edge of the mobile network, closer to the task execution end, and can effectively mitigate the communication latency problem. However, the massive and heterogeneous nature of servers in edge computing systems brings new challenges to task scheduling and resource management, and the booming development of artificial neural networks provides us with more powerful methods to alleviate this limitation. Therefore, in this paper, we proposed a time series forecasting model incorporating Conv1D, LSTM and GRU for edge computing device resource scheduling, trained and tested the forecasting model using a small self-built dataset, and achieved competitive experimental results.Keywords
Cite This Article
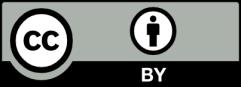