Open Access
ARTICLE
Optimizing Deep Learning for Computer-Aided Diagnosis of Lung Diseases: An Automated Method Combining Evolutionary Algorithm, Transfer Learning, and Model Compression
1 College of Information Technology, Kingdom University, Riffa, 40434, Bahrain
2 SMART Lab, University of Tunis, ISG, Tunis, Tunisia
3 Department of Information Systems, College of Computer Engineering and Sciences, Prince Sattam bin Abdulaziz University, Al-Kharj, 11942, Saudi Arabia
* Corresponding Author: Ali Louati. Email:
(This article belongs to the Special Issue: Intelligent Biomedical Image Processing and Computer Vision)
Computer Modeling in Engineering & Sciences 2024, 138(3), 2519-2547. https://doi.org/10.32604/cmes.2023.030806
Received 24 April 2023; Accepted 23 August 2023; Issue published 15 December 2023
Abstract
Recent developments in Computer Vision have presented novel opportunities to tackle complex healthcare issues, particularly in the field of lung disease diagnosis. One promising avenue involves the use of chest X-Rays, which are commonly utilized in radiology. To fully exploit their potential, researchers have suggested utilizing deep learning methods to construct computer-aided diagnostic systems. However, constructing and compressing these systems presents a significant challenge, as it relies heavily on the expertise of data scientists. To tackle this issue, we propose an automated approach that utilizes an evolutionary algorithm (EA) to optimize the design and compression of a convolutional neural network (CNN) for X-Ray image classification. Our approach accurately classifies radiography images and detects potential chest abnormalities and infections, including COVID-19. Furthermore, our approach incorporates transfer learning, where a pre-trained CNN model on a vast dataset of chest X-Ray images is fine-tuned for the specific task of detecting COVID-19. This method can help reduce the amount of labeled data required for the task and enhance the overall performance of the model. We have validated our method via a series of experiments against state-of-the-art architectures.Keywords
Cite This Article
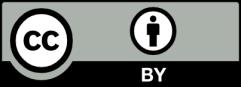
This work is licensed under a Creative Commons Attribution 4.0 International License , which permits unrestricted use, distribution, and reproduction in any medium, provided the original work is properly cited.