Open Access
ARTICLE
Computational Analysis of Novel Extended Lindley Progressively Censored Data
1 Department of Mathematical Sciences, College of Science, Princess Nourah bint Abdulrahman University, P.O. Box 84428, Riyadh, 11671, Saudi Arabia
2 Department of Statistics, Faculty of Science, King Abdulaziz University, Jeddah, 21589, Saudi Arabia
3 Department of Statistics, Faculty of Commerce, Zagazig University, Zagazig, Egypt
4 Faculty of Technology and Development, Zagazig University, Zagazig, 44519, Egypt
* Corresponding Author: Ahmed Elshahhat. Email:
(This article belongs to the Special Issue: Advanced Computational Models for Decision-Making of Complex Systems in Engineering)
Computer Modeling in Engineering & Sciences 2024, 138(3), 2571-2596. https://doi.org/10.32604/cmes.2023.030582
Received 13 April 2023; Accepted 07 September 2023; Issue published 15 December 2023
Abstract
A novel extended Lindley lifetime model that exhibits unimodal or decreasing density shapes as well as increasing, bathtub or unimodal-then-bathtub failure rates, named the Marshall-Olkin-Lindley (MOL) model is studied. In this research, using a progressive Type-II censored, various inferences of the MOL model parameters of life are introduced. Utilizing the maximum likelihood method as a classical approach, the estimators of the model parameters and various reliability measures are investigated. Against both symmetric and asymmetric loss functions, the Bayesian estimates are obtained using the Markov Chain Monte Carlo (MCMC) technique with the assumption of independent gamma priors. From the Fisher information data and the simulated Markovian chains, the approximate asymptotic interval and the highest posterior density interval, respectively, of each unknown parameter are calculated. Via an extensive simulated study, the usefulness of the various suggested strategies is assessed with respect to some evaluation metrics such as mean squared errors, mean relative absolute biases, average confidence lengths, and coverage percentages. Comparing the Bayesian estimations based on the asymmetric loss function to the traditional technique or the symmetric loss function-based Bayesian estimations, the analysis demonstrates that asymmetric loss function-based Bayesian estimations are preferred. Finally, two data sets, representing vinyl chloride and repairable mechanical equipment items, have been investigated to support the approaches proposed and show the superiority of the proposed model compared to the other fourteen lifetime models.Keywords
Supplementary Material
Supplementary Material FileAbbreviations
ACI | Approximative confidence interval |
ACL | Average confidence length |
AIC | Akaike information criterion |
APE | Alpha power exponential |
Av.Es | Average estimates |
BIC | Bayesian information criterion |
BGR | Brooks-Gelman-Rubin |
CA | Consistent Akaike |
CP | Coverage percentage |
E | Exponential |
FP | Failure percentage |
G | Gamma |
GE | Generalized-exponential |
GEnt | General entropy |
HQ | Hannan-Quinn |
HPD | Highest posterior density |
HRF | Hazard rate function |
KS | Kolmogorov-Smirnov |
L | Lindley |
M-H | Metropolis-Hastings |
MCMC | Markov Chain Monte Carlo |
MLE | Maximum likelihood estimator |
MOAPE | Marshall-Olkin alpha power exponential |
MOE | Marshall-Olkin exponential |
MOG | Marshall-Olkin Gompertz |
MOGE | Marshall-Olkin generalized exponential |
MOL | Marshall-Olkin-Lindley |
MOLE | Marshall-Olkin logistic-exponential |
MONH | Marshall-Olkin Nadarajah-Haghighi |
MOW | Marshall-Olkin Weibull |
MRAB | Mean relative absolute bias |
NH | Nadarajah-Haghighi |
NL | Negative log-likelihood |
Probability density function | |
PT-IIC | Progressive Type-II censored |
Quantile-quantile | |
RF | Reliability function |
RME | Repairable mechanical equipment |
RMSE | Root mean squared-error |
SE | Squared error |
St.D | Standard deviation |
St.E | Standard-error |
W | Weibull |
One of the key research areas in the concept of distribution theory is the evolution of suggesting new statistical distributions. Such generalized distributions allow modelling for a range of disciplines, including reliability, engineering and medicine with even greater flexibility. The two-parameter Marshall-Olkin-Lindley (MOL) distribution suggested by Ghitany et al. [1] is one of the novel versions of Marshall-Olkin models that take the conventional Lindley distribution as a baseline distribution. Assume that X is a lifetime random variable of an experimental item that follows the MOL distribution, denoted by
Hence, its probability density function (PDF),
and
where
Figure 1: Various shapes for the density and hazard functions of MOEL distribution
Frequently, life testing studies are stopped before all of the components fail. Due to financial or time restrictions, it occurs. The observations that emerge from this type of scenario are known as the censored sample. The literature has developed a number of filtering techniques for the evaluation of various life-testing strategies. The two most popular censorship techniques among the various techniques are Types I and II. The experimental units cannot be removed during a life-testing experiment, however, under any of these censorship techniques. This adaptability is featured in a life-testing experiment with progressive censoring. Since the publication of the book by Balakrishnan et al. [3], extensive research has been conducted on the various facets of progressive censoring. A recent book by Balakrishnan et al. [4] has an extensive compilation of different studies connected to the progressive censorship strategy.
In order to estimate the parameters of the MOL distribution, we work with the progressive Type-II censored (PT-IIC) sample in this study. The PT-IIC sample can be explained as follows: assume that a life testing experiment involving
where A is a constant that is independent of the parameters and
Due to the MOL distribution’s flexibility and the PT-IIC scheme’s effectiveness in gathering sample data, no study investigated the estimation problems of the MOL distribution in the case of the PT-IIC sample. Also, in the original work of Ghitany et al. [1], they just used the maximum likelihood approach to estimate the parameters of the MOL distribution without saying anything about the Bayesian estimation method. In addition, they estimated only the unknown parameters, while it is of interest to reliability engineers and other practitioners to see the performance of the reliability measures of the used distribution. Therefore, this paper’s main goal is to examine frequentist and Bayesian inferences of the MOL distribution’s unknown parameters under the PT-IIC, along with the related reliability indices, such as the RF and HRF. As expected, it is found that the maximum likelihood estimators (MLEs) of
The remainder of the article is structured as follows. We present the MLEs and ACIs of the unknown parameters, RF and HRF, in Section 2. We acquire the Bayesian inference in Section 3. Sections 4 and 5 separately describe the findings of the Monte Carlo simulation and the analysis of two data sets, respectively. At last, we sum up the paper in Section 6.
2 Maximum Likelihood Estimation
In this part, we estimate the unknown parameters, RF and HRF of the MOL distribution using the method of maximum likelihood based on the PT-IIC sample. The ACIs of the different parameters are explained in addition to the point estimators. Assume that
where
where
and
where
and
Utilizing the asymptotic normality of the MLEs is the most common approach for establishing confidence bounds for the parameters. The MLEs’ asymptotic distribution can be expressed as
with
and
where
where
and
where
Suppose that
As a result, with
For analyzing failure time data, the Bayesian estimation approach has attracted a lot of attention. It uses one’s past knowledge of the parameters and also takes into account the information that is readily available. In this section, the Bayesian estimators of
The posterior distribution of the unknown parameters
where
If one setting
Now, in order to derive the Bayesian estimators, we take into account the SE and GEnt loss functions. The Bayesian estimator for the SE loss function is the posterior mean, which considers overestimation and underestimation equally. In contrast hand, the GEnt loss function offers different influences for overestimation and underestimation. Calabria et al. [12] introduced the GEnt loss function, which is defined as:
where
given that
and
It is obvious that it is difficult to determine the Bayesian estimators using (13) and (14) analytically. In order to acquire the Bayesian estimates of
and
It is evident that the conditional distributions of
Step 1. Set
Step 2. Begin with the initial guesses
Step 3. From (15), generate
Step 4. Use (16) to get
Step 5. Based on the generated
and
Step 6. Put
Step 7. Repeat steps 3–6, M times to compute
where
In this study, the first B generated samples are discarded in order to ensure convergence and remove the appeal of initial guesses. In this situation, we possess
To construct the HPD credible intervals of
where
To evaluate the behavior of the proposed estimators of
where
Once the 1,000 PT-IIC samples collected, the maximum likelihood and 95% ACI estimates of
To monitor whether the simulated Markovian sample is sufficiently close to the target posterior, beside the trace and autocorrelation plots, we purpose to consider the Brooks-Gelman-Rubin (BGR) diagnostic statistic, which evaluates the convergence by analyzing the difference between the variance-within chains and the variance-between chains for each model parameter, for details see [17]. To establish this purpose, by running two chains using
Figure 2: Trace (top) and Autocorrelation (bottom) plots for MCMC draws of
Figure 3: The BGR diagnostic for MCMC draws of
The average estimates (Av.Es) from classical (or Bayesian) approach of
where
Further, the comparison between point estimates of
and
respectively.
Furthermore, the comparison between interval estimates of the same unknown parameters is made using their average confidence lengths (ACLs) and coverage percentages (CPs) which can be computed as
and
respectively, where
Heatmap is a method of representing data graphically where values are depicted by color, making it easy to visualize complex data and understand it at a glance. So, via
Figure 4: Heatmap plots for the point and interval results of
Figure 5: Heatmap plots for the point and interval results of
Figure 6: Heatmap plots for the point and interval results of
Figure 7: Heatmap plots for the point and interval results of
From Figs. 4–7, in terms of the lowest RMSE, MRAB and ACL values as well as the highest CP values, the following comments can be drawn:
• Generally, the proposed point and interval estimates of
• As
• Bayesian estimates against the GEnt loss function perform superior than those obtained against the SE loss function, and both perform better compared to the other estimates due to the gamma prior information. Similar result is also observed in the case of HPD credible interval estimates.
• To evaluate the effect of parameter loss, it can be seen that the asymmetric Bayes estimates of
• Comparing the considered prior sets 1 and 2, due to the variance of prior 2 is smaller than the variance of prior 1, it is observed that the Bayesian estimates and associated HPD credible intervals under prior 2 of all unknown parameters have good perform than others.
• Asymmetric Bayesian estimates of
• Comparing the censoring schemes 1, 2 and 3, it is clear that the both proposed point and interval estimates of
• Finally, to estimate the MOL distribution parameters or its reliability characteristics under PT-IIC mechanism, the Bayesian M-H algorithm method is recommended.
In order to demonstrate the significance of the suggested inferential methodologies and the applicability of study objectives to actual phenomena, this part presents two practical applications from the domains of engineering and chemistry.
Vinyl chloride is a known human carcinogen and a rapidly burning colorless gas. In this application, 34 data points (measured in milligrams/liter) as presented in see Table 1 for vinyl chloride were taken from clean-up-gradient monitoring wells and analyzed. This data set was reported by Bhaumik et al. [19] and re-analyzed also by Elshahhat et al. [20], Alotaibi et al. [21], Elshahhat et al. [22].
To verify the flexibility of the MOL model, the MOL distribution is compared with fourteen well-known distributions, (for
Different goodness-of-fit metrics, including the negative log-likelihood (NL), Akaike information criterion (AIC), Bayesian information criterion (BIC), Hannan-Quinn (HQ), Consistent Akaike (CA), and Kolmogorov-Smirnov (KS) statistic with its p-value, must be taken into account when comparing two (or more) distributions. The given goodness criteria are computed using the maximum likelihood and its standard-error (St.E) of each unknown parameter, as shown in Table 2. It is evident that the MOL distribution offers a better fit than other rival distributions based on the lowest values of NL, AIC, BIC, HQ, CA, and KS as well as the greatest p-value.
We also provided the quantile-quantile (QQ) plot as a graphical demonstration, via
Figure 8: The Q-Q plots of the MOL and some competing distributions from vinyl chloride data
In Fig. 9, via
Figure 9: (a) Histograms and fitted PDFs and (b) Empirical and fitted RFs under vinyl chloride data
Now three different PT-IIC samples, from the complete vinyl chloride data, are generated with
From each sample in Table 3, useful statistics for the MCMC variates of
Figure 10: Trace (top) and Histograms (bottom) plots of
In each trace plot, the sample mean and two bounds of 95% HPD credible intervals of
In this application, from the engineering field, we will explain our theoretical results based on the time between consecutive failures for repairable mechanical equipment (RME) items depicted in Table 7. Murthy et al. [36] initially conveyed this data and it has also been examined by Elshahhat et al. [37], Nassar et al. [38], and Elshahhat et al. [39]. Employing the competitive statistical distributions as well as the model selection criteria proposed in Subsection 5.1, the MOL distribution based on the complete RME data is compared. All results of the MOL distribution and other models are provided in Table 8. It suggests that the MOL distribution is the most suitable model to fit the MRE data when compared to others.
Also, using the complete RME data, Fig. 11 displays the QQ plots of MOL, MOE, MOW, MOG, MOGE, MOLE, MONH and MOAPE distributions. It supports the same findings reported in Table 8 also. Further, three graphics of goodness-of-fit are investigated; (i) plot of histograms of RME data with fitted PDFs, and (ii) plot of the fitted and empirical RFs under RME data are shown in Fig. 12. It indicates that the MOL distribution is the best model compared to its competitive models.
Figure 11: The Q-Q plots of the competing models from mechanical equipments data
Figure 12: (a) Histograms and fitted PDFs and (b) Empirical and fitted RFs from the RME data
From the complete RME data, three different PT-IIC samples are generated with
From the PT-IIC sample generated by
Figure 13: Trace (top) and Histograms (bottom) plots of
In this study, we looked into the statistical inference of the Marshall-Olkin Lindley distribution’s unknown parameters, reliability, and hazard rate functions under progressively Type-II censored data. The various parameters of interest are inferred using both classical and Bayesian methods. The normal approximation of the maximum likelihood estimators is also used to create the approximate confidence intervals. The Bayesian estimations are addressed by employing independent gamma priors and symmetric and asymmetric loss functions. We have indicated that the explicit expressions of the proposed Bayesian estimators are not available. The Markov Chain Monte Carlo technique is employed as a result. For each parameter, the highest posterior density credible intervals are also attained. We conducted a thorough simulation analysis and examined two applications to real-world data sets to evaluate the effectiveness of the delivered estimations. The findings of the numerical study showed that when progressively Type-II censored data were given, the suggested point and interval estimations of the Marshall-Olkin Lindley distribution acted reasonably. More specifically, the highest posterior density credible intervals were advised and the Bayesian estimates utilizing the general entropy loss function outperformed all other estimates. In addition, the real data analysis showed that the Marshall-Olkin Lindley distribution could be used as a good model to fit vinyl chloride and repairable mechanical equipment data sets rather than some other Marshall-Olkin models, including Marshall-Olkin Weibull, Marshall-Olkin Gompertz, Marshall-Olkin generalized exponential and Marshall-Olkin logistic-exponential distributions. In future work, it is of interest to investigate the estimation problems of the considered distribution based on other censoring schemes like an adaptive progressive Type-II censoring scheme. Another significant future work to be addressed is exploring the performance of dependability metrics of the utilized model in the case of accelerated life tests.
Acknowledgement: The authors would desire to express their thanks to the editor and the three anonymous referees for useful suggestions and valuable comments. Princess Nourah bint Abdulrahman University Researchers Supporting Project and Princess Nourah bint Abdulrahman University, Riyadh, Saudi Arabia.
Funding Statement: This research was funded by Princess Nourah bint Abdulrahman University Researchers Supporting Project Number (PNURSP2023R50), Princess Nourah bint Abdulrahman University, Riyadh, Saudi Arabia.
Author Contributions: The authors confirm contribution to the paper as follows: study conception and design: R. Alotaibi, M. Nassar, and A. Elshahhat; data collection: A. Elshahhat; analysis and interpretation of results: A. Elshahhat; draft manuscript preparation: M. Nassar, R. Alotaibi. All authors reviewed the results and approved the final version of the manuscript.
Availability of Data and Materials: The data that support the findings of this study are available in the text.
Conflicts of Interest: The authors declare that they have no conflicts of interest to report regarding the present study.
Supplementary Materials: The supplementary material is available online at https://doi.org/10.32604/cmes.2023.030582.
References
1. Ghitany, M. E., Al-Mutairi, D. K., Al-Awadhi, F. A., Al-Burais, M. M. (2012). Marshall-Olkin extended Lindley distribution and its application. International Journal of Applied Mathematics, 25(5), 709–721. [Google Scholar]
2. do Espirito Santo, A. P. J., Mazucheli, J. (2015). Comparison of estimation methods for the Marshall-Olkin extended Lindley distribution. Journal of Statistical Computation and Simulation, 85(17), 3437–3450. [Google Scholar]
3. Balakrishnan, N., Aggarwala, R. (2000). Progressive censoring: Theory, methods, and applications. Birkhäuser, Boston: Springer Science & Business Media. [Google Scholar]
4. Balakrishnan, N., Cramer, E. (2014). The art of progressive censoring. Birkhäuser, New York: Springer. [Google Scholar]
5. Sultan, K. S., Alsadat, N. H., Kundu, D. (2014). Bayesian and maximum likelihood estimations of the inverse Weibull parameters under progressive type-II censoring. Journal of Statistical Computation and Simulation, 84(10), 2248–2265. [Google Scholar]
6. Guo, L., Gui, W. (2018). Statistical inference of the reliability for generalized exponential distribution under progressive type-II censoring schemes. IEEE Transactions on Reliability, 67(2), 470–480. [Google Scholar]
7. Joukar, A., Ramezani, M., MirMostafaee, S. M. T. K. (2020). Estimation of P (X > Y) for the power Lindley distribution based on progressively type II right censored samples. Journal of Statistical Computation and Simulation, 90(2), 355–389. [Google Scholar]
8. Elshahhat, A., Rastogi, M. K. (2021). Estimation of parameters of life for an inverted Nadarajah-Haghighi distribution from Type-II progressively censored samples. Journal of the Indian Society for Probability and Statistics, 22(1), 113–154. [Google Scholar]
9. Alotaibi, R., Nassar, M., Rezk, H., Elshahhat, A. (2022). Inferences and engineering applications of alpha power Weibull distribution using progressive Type-II censoring. Mathematics, 10(16), 2901. [Google Scholar]
10. Okasha, H., Nassar, M. (2022). Product of spacing estimation of entropy for inverse Weibull distribution under progressive type-II censored data with applications. Journal of Taibah University for Science, 16(1), 259–269. [Google Scholar]
11. Maiti, K., Kayal, S., Kundu, D. (2022). Statistical Inference on the shannon and rényi entropy measures of generalized exponential distribution under the progressive censoring. SN Computer Science, 3(4), 1–21. [Google Scholar]
12. Calabria, R., Pulcini, G. (1994). An engineering approach to Bayes estimation for the Weibull distribution. Microelectronics Reliability, 34(5), 789–802. [Google Scholar]
13. Henningsen, A., Toomet, O. (2011). maxLik: A package for maximum likelihood estimation in R. Computational Statistics, 26(3), 443–458. [Google Scholar]
14. Kundu, D. (2008). Bayesian inference and life testing plan for the Weibull distribution in presence of progressive censoring. Technometrics, 50(2), 144–154. [Google Scholar]
15. Dey, S., Elshahhat, A., Nassar, M. (2022). Analysis of progressive type-II censored gamma distribution. Computational Statistics, 38(1), 481–508. [Google Scholar]
16. Plummer, M., Best, N., Cowles, K., Vines, K. (2006). CODA: Convergence diagnosis and output analysis for MCMC. R News, 6, 7–11. [Google Scholar]
17. Roy, V. (2020). Convergence diagnostics for Markov Chain Monte Carlo. Annual Review of Statistics and Its Application, 7(1), 387–412. [Google Scholar]
18. Elshahhat, A. (2022). R programming language for data analytics. The 55th Annual International Conference on Data Science, Egypt, Cairo University. https://doi.org/10.13140/RG.2.2.15044.09607/1 [Google Scholar] [CrossRef]
19. Bhaumik, D. K., Kapur, K., Gibbons, R. D. (2009). Testing parameters of a gamma distribution for small samples. Technometrics, 51(3), 326–334. [Google Scholar]
20. Elshahhat, A., Elemary, B. R. (2021). Analysis for xgamma parameters of life under type-II adaptive progressively hybrid censoring with applications in engineering and chemistry. Symmetry, 13(11), 2112. [Google Scholar]
21. Alotaibi, R., Nassar, M., Elshahhat, A. (2022). Computational analysis of XLindley parameters using adaptive Type-II progressive hybrid censoring with applications in chemical engineering. Mathematics, 10(18), 3355. [Google Scholar]
22. Elshahhat, A., Dutta, S., Abo-Kasem, O. E., Mohammed, H. S. (2023). Statistical analysis of the Gompertz-Makeham model using adaptive progressively hybrid Type-II censoring and its applications in various sciences. Journal of Radiation Research and Applied Sciences, 16(4), 100644. https://doi.org/10.1016/j.jrras.2023.100644 [Google Scholar] [CrossRef]
23. Marshall, A. W., Olkin, I. (1997). A new method for adding a parameter to a family of distributions with application to the exponential and Weibull families. Biometrika, 84(3), 641–652. [Google Scholar]
24. Cordeiro, G. M., Lemonte, A. J. (2013). On the Marshall-Olkin extended Weibull distribution. Statistical Papers, 54(2), 333–353. [Google Scholar]
25. Eghwerido, J. T., Ogbo, J. O., Omotoye, A. E. (2021). The Marshall-Olkin Gompertz distribution: Properties and applications. Statistica, 81, 183–215. [Google Scholar]
26. Ristić, M. M., Kundu, D. (2015). Marshall-Olkin generalized exponential distribution. Metron, 73(3), 317–333. [Google Scholar]
27. Mansoor, M., Tahir, M. H., Cordeiro, G. M., Provost, S. B., Alzaatreh, A. (2019). The Marshall-Olkin logistic-exponential distribution. Communications in Statistics-Theory and Methods, 48(2), 220–234. [Google Scholar]
28. Lemonte, A. J., Cordeiro, G. M., Moreno-Arenas, G. (2016). A new useful three-parameter extension of the exponential distribution. Statistics, 50, 312–337. [Google Scholar]
29. Nassar, M., Kumar, D., Dey, S., Cordeiro, G. M., Afify, A. Z. (2019). The Marshall-Olkin alpha power family of distributions with applications. Journal of Computational and Applied Mathematics, 351, 41–53. [Google Scholar]
30. Mahdavi, A., Kundu, D. (2017). A new method for generating distributions with an application to exponential distribution. Communications in Statistics-Theory and Methods, 46(13), 6543–6557. [Google Scholar]
31. Gupta, R. D., Kundu, D. (1999). Theory and methods: Generalized exponential distributions. Australian and New Zealand Journal of Statistics, 41(2), 173–188. [Google Scholar]
32. Nadarajah, S., Haghighi, F. (2011). An extension of the exponential distribution. Statistics, 45(6), 543–558. [Google Scholar]
33. Weibull, W. (1951). A statistical distribution function of wide applicability. Journal of Applied Mechanics, 18(3), 293–297. [Google Scholar]
34. Johnson, N., Kotz, S., Balakrishnan, N. (1994). Continuous univariate distributions. 2nd edition. New York: John Wiley and Sons. [Google Scholar]
35. Lindley, D. V. (1958). Fiducial distributions and Bayes’ theorem. Journal of the Royal Statistical Society: Series B, 20, 102–107. [Google Scholar]
36. Murthy, D. N. P., Xie, M., Jiang, R. (2004). Weibull models. In: Wiley series in probability and statistics. New Jersey: John Wiley and Sons. [Google Scholar]
37. Elshahhat, A., Aljohani, H. M., Afify, A. Z. (2021). Bayesian and classical inference under Type-II censored samples of the extended inverse gompertz distribution with engineering applications. Entropy, 23(12), 1578. [Google Scholar] [PubMed]
38. Nassar, M., Elshahhat, A. (2023). Estimation procedures and optimal censoring schemes for an improved adaptive progressively type-II censored Weibull distribution. Journal of Applied Statistics. https://doi.org/10.1080/02664763.2023.2230536 [Google Scholar] [CrossRef]
39. Elshahhat, A., Almetwally, E. M., Dey, S., Mohammed, H. S. (2023). Analysis of WE parameters of life using adaptive-progressively Type-II hybrid censored mechanical equipment data. Axioms, 12(7), 690. [Google Scholar]
Cite This Article
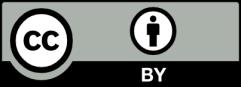