Open Access
ARTICLE
Tool Wear State Recognition with Deep Transfer Learning Based on Spindle Vibration for Milling Process
1 School of Aerospace Engineering, Xiamen University, Xiamen, 361005, China
2 School of Aerospace Science and Technology, Xidian University, Xi'an, 710126, China
* Corresponding Author: Binqiang Chen. Email:
(This article belongs to the Special Issue: AI and Machine Learning Modeling in Civil and Building Engineering)
Computer Modeling in Engineering & Sciences 2024, 138(3), 2825-2844. https://doi.org/10.32604/cmes.2023.030378
Received 03 April 2023; Accepted 28 July 2023; Issue published 15 December 2023
Abstract
The wear of metal cutting tools will progressively rise as the cutting time goes on. Wearing heavily on the tool will generate significant noise and vibration, negatively impacting the accuracy of the forming and the surface integrity of the workpiece. Hence, during the cutting process, it is imperative to continually monitor the tool wear state and promptly replace any heavily worn tools to guarantee the quality of the cutting. The conventional tool wear monitoring models, which are based on machine learning, are specifically built for the intended cutting conditions. However, these models require retraining when the cutting conditions undergo any changes. This method has no application value if the cutting conditions frequently change. This manuscript proposes a method for monitoring tool wear based on unsupervised deep transfer learning. Due to the similarity of the tool wear process under varying working conditions, a tool wear recognition model that can adapt to both current and previous working conditions has been developed by utilizing cutting monitoring data from history. To extract and classify cutting vibration signals, the unsupervised deep transfer learning network comprises a one-dimensional (1D) convolutional neural network (CNN) with a multi-layer perceptron (MLP). To achieve distribution alignment of deep features through the maximum mean discrepancy algorithm, a domain adaptive layer is embedded in the penultimate layer of the network. A platform for monitoring tool wear during end milling has been constructed. The proposed method was verified through the execution of a full life test of end milling under multiple working conditions with a Cr12MoV steel workpiece. Our experiments demonstrate that the transfer learning model maintains a classification accuracy of over 80%. In comparison with the most advanced tool wear monitoring methods, the presented model guarantees superior performance in the target domains.Keywords
Cite This Article
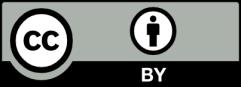
This work is licensed under a Creative Commons Attribution 4.0 International License , which permits unrestricted use, distribution, and reproduction in any medium, provided the original work is properly cited.