Open Access
ARTICLE
Enhanced Temporal Correlation for Universal Lesion Detection
1 School of Computer Science and Technology, Shandong University of Finance and Economics, Jinan, China
2 School of Information Science and Technology, Linyi University, Linyi, China
3 School of Creative Technologies, University of Portsmouth, Portsmouth, UK
* Corresponding Author: Muwei Jian. Email:
(This article belongs to the Special Issue: Deep Learning based Computational Methods for Abnormality Detection in Human Medical Images)
Computer Modeling in Engineering & Sciences 2024, 138(3), 3051-3063. https://doi.org/10.32604/cmes.2023.030236
Received 28 March 2023; Accepted 01 August 2023; Issue published 15 December 2023
Abstract
Universal lesion detection (ULD) methods for computed tomography (CT) images play a vital role in the modern clinical medicine and intelligent automation. It is well known that single 2D CT slices lack spatial-temporal characteristics and contextual information compared to 3D CT blocks. However, 3D CT blocks necessitate significantly higher hardware resources during the learning phase. Therefore, efficiently exploiting temporal correlation and spatial-temporal features of 2D CT slices is crucial for ULD tasks. In this paper, we propose a ULD network with the enhanced temporal correlation for this purpose, named TCE-Net. The designed TCE module is applied to enrich the discriminate feature representation of multiple sequential CT slices. Besides, we employ multi-scale feature maps to facilitate the localization and detection of lesions in various sizes. Extensive experiments are conducted on the DeepLesion benchmark demonstrate that this method achieves 66.84% and 78.18% for FS@0.5 and FS@1.0, respectively, outperforming compared state-of-the-art methods.Keywords
Cite This Article
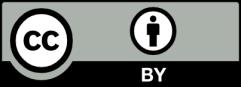
This work is licensed under a Creative Commons Attribution 4.0 International License , which permits unrestricted use, distribution, and reproduction in any medium, provided the original work is properly cited.