Open Access
ARTICLE
An Optimized System of Random Forest Model by Global Harmony Search with Generalized Opposition-Based Learning for Forecasting TBM Advance Rate
1 School of Resources and Safety Engineering, Central South University, Changsha, 410083, China
2 School of Civil and Environmental Engineering, University of Technology Sydney, New South Wales, Australia
3 Centre for Advanced Modelling and Geospatial Information Systems, School of Civil and Environmental Engineering, Faculty of Engineering and Information Technology, University of Technology Sydney, New South Wales, Australia
4 Civil and Infrastructure Engineering Discipline, School of Engineering, Royal Melbourne Institute of Technology (RMIT), Melbourne, Victoria, 3001, Australia
* Corresponding Authors: Jian Zhou. Email: ,
(This article belongs to the Special Issue: Meta-heuristic Algorithms in Materials Science and Engineering)
Computer Modeling in Engineering & Sciences 2024, 138(3), 2873-2897. https://doi.org/10.32604/cmes.2023.029938
Received 15 March 2023; Accepted 20 July 2023; Issue published 15 December 2023
Abstract
As massive underground projects have become popular in dense urban cities, a problem has arisen: which model predicts the best for Tunnel Boring Machine (TBM) performance in these tunneling projects? However, performance level of TBMs in complex geological conditions is still a great challenge for practitioners and researchers. On the other hand, a reliable and accurate prediction of TBM performance is essential to planning an applicable tunnel construction schedule. The performance of TBM is very difficult to estimate due to various geotechnical and geological factors and machine specifications. The previously-proposed intelligent techniques in this field are mostly based on a single or base model with a low level of accuracy. Hence, this study aims to introduce a hybrid random forest (RF) technique optimized by global harmony search with generalized opposition-based learning (GOGHS) for forecasting TBM advance rate (AR). Optimizing the RF hyper-parameters in terms of, e.g., tree number and maximum tree depth is the main objective of using the GOGHS-RF model. In the modelling of this study, a comprehensive database with the most influential parameters on TBM together with TBM AR were used as input and output variables, respectively. To examine the capability and power of the GOGHS-RF model, three more hybrid models of particle swarm optimization-RF, genetic algorithm-RF and artificial bee colony-RF were also constructed to forecast TBM AR. Evaluation of the developed models was performed by calculating several performance indices, including determination coefficient (R2), root-mean-square-error (RMSE), and mean-absolute-percentage-error (MAPE). The results showed that the GOGHS-RF is a more accurate technique for estimating TBM AR compared to the other applied models. The newly-developed GOGHS-RF model enjoyed R2 = 0.9937 and 0.9844, respectively, for train and test stages, which are higher than a pre-developed RF. Also, the importance of the input parameters was interpreted through the SHapley Additive exPlanations (SHAP) method, and it was found that thrust force per cutter is the most important variable on TBM AR. The GOGHS-RF model can be used in mechanized tunnel projects for predicting and checking performance.Keywords
Cite This Article
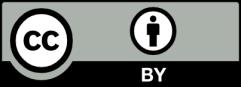
This work is licensed under a Creative Commons Attribution 4.0 International License , which permits unrestricted use, distribution, and reproduction in any medium, provided the original work is properly cited.