Open Access
ARTICLE
A Hybrid Classification and Identification of Pneumonia Using African Buffalo Optimization and CNN from Chest X-Ray Images
1 Computer Science Department, Community College, King Saud University, Riyadh, 11437, Saudi Arabia
2 Information System Department, Faculty of Computers and Information, Assiut University, Assiut, Egypt
3 Mathematics Department, Faculty of Science, Al-Azhar University, Cairo, Egypt
4 Cyber Technology Institute (CTI), De Montfort University, Leicester, UK
* Corresponding Author: Nasser Alalwan. Email:
(This article belongs to the Special Issue: Intelligent Biomedical Image Processing and Computer Vision)
Computer Modeling in Engineering & Sciences 2024, 138(3), 2497-2517. https://doi.org/10.32604/cmes.2023.029910
Received 14 March 2023; Accepted 01 August 2023; Issue published 15 December 2023
Abstract
An illness known as pneumonia causes inflammation in the lungs. Since there is so much information available from various X-ray images, diagnosing pneumonia has typically proven challenging. To improve image quality and speed up the diagnosis of pneumonia, numerous approaches have been devised. To date, several methods have been employed to identify pneumonia. The Convolutional Neural Network (CNN) has achieved outstanding success in identifying and diagnosing diseases in the fields of medicine and radiology. However, these methods are complex, inefficient, and imprecise to analyze a big number of datasets. In this paper, a new hybrid method for the automatic classification and identification of Pneumonia from chest X-ray images is proposed. The proposed method (ABO-CNN) utilized the African Buffalo Optimization (ABO) algorithm to enhance CNN performance and accuracy. The Weinmed filter is employed for pre-processing to eliminate unwanted noises from chest X-ray images, followed by feature extraction using the Grey Level Co-Occurrence Matrix (GLCM) approach. Relevant features are then selected from the dataset using the ABO algorithm, and ultimately, high-performance deep learning using the CNN approach is introduced for the classification and identification of Pneumonia. Experimental results on various datasets showed that, when contrasted to other approaches, the ABO-CNN outperforms them all for the classification tasks. The proposed method exhibits superior values like 96.95%, 88%, 86%, and 86% for accuracy, precision, recall, and F1-score, respectively.Keywords
Cite This Article
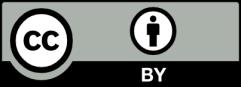
This work is licensed under a Creative Commons Attribution 4.0 International License , which permits unrestricted use, distribution, and reproduction in any medium, provided the original work is properly cited.