Open Access
ARTICLE
Traffic Control Based on Integrated Kalman Filtering and Adaptive Quantized Q-Learning Framework for Internet of Vehicles
1 Faculty of Electronic and Computer Engineering, Universiti Teknikal Malaysia Melaka (UTeM), Durian Tunggal, Malaysia
2 College of Computing & Informatics, Saudi Electronic University, Riyadh, Saudi Arabia
3 Department of Electrical, Electronic and System Engineering, Universiti Kebangsaan Malaysia, UKM Bangi, Selangor, Malaysia
4 Computer Technology Engineering, College of Engineering Technology, Al-Kitab University, Kirkuk, Iraq
5 Faculty of Engineering and Electronic Engineering, Universiti Tun Hussein Onn Malaysia (UTHM), Batu Pahat, Malaysia
6 Research Center Department, The University of Mashreq, Baghdad, Iraq
* Corresponding Authors: Othman S. Al-Heety. Email: ; Zahriladha Zakaria. Email:
Computer Modeling in Engineering & Sciences 2024, 138(3), 2103-2127. https://doi.org/10.32604/cmes.2023.029509
Received 23 February 2023; Accepted 06 July 2023; Issue published 15 December 2023
Abstract
Intelligent traffic control requires accurate estimation of the road states and incorporation of adaptive or dynamically adjusted intelligent algorithms for making the decision. In this article, these issues are handled by proposing a novel framework for traffic control using vehicular communications and Internet of Things data. The framework integrates Kalman filtering and Q-learning. Unlike smoothing Kalman filtering, our data fusion Kalman filter incorporates a process-aware model which makes it superior in terms of the prediction error. Unlike traditional Q-learning, our Q-learning algorithm enables adaptive state quantization by changing the threshold of separating low traffic from high traffic on the road according to the maximum number of vehicles in the junction roads. For evaluation, the model has been simulated on a single intersection consisting of four roads: east, west, north, and south. A comparison of the developed adaptive quantized Q-learning (AQQL) framework with state-of-the-art and greedy approaches shows the superiority of AQQL with an improvement percentage in terms of the released number of vehicles of AQQL is 5% over the greedy approach and 340% over the state-of-the-art approach. Hence, AQQL provides an effective traffic control that can be applied in today’s intelligent traffic system.Keywords
Cite This Article
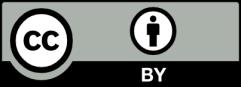
This work is licensed under a Creative Commons Attribution 4.0 International License , which permits unrestricted use, distribution, and reproduction in any medium, provided the original work is properly cited.